PB1855: USE OF MACHINE LEARNING MODEL TO IDENTIFY PATIENTS RECEIVING OPTIMAL TREATMENT AND THE ASSOCIATED NON-BIOLOGICAL FACTORS: A POPULATION-BASED STUDY
HemaSphere(2023)
Abstract
Topic: 4. Acute myeloid leukemia - Clinical Background: AML patients not admitted to specialised haematology unit (SHU) during their care pathway have less access to curative treatment. Aims: Our aim is to determine whether access to optimal curative treatment is based on the patient’s clinico-biological characteristics or affected by non-biological factors. Methods: Patients were described according to their treatment modalities (curative, non-curative and untreated). In 1000 times bootstrapping process, we trained machine learning (ML) algorithms to learn the clinico-biological characteristics that were used to decide the treatment modalities of AML gold standard patient (treated in academic hospitals by haematologists within 5 days of their diagnosis, n=297). Based on the ML model predictions, we create two groups of patients: optimal treatment patient (predicted as treated and effectively treated) versus non-optimal treatment patients (predicted as treated but effectively untreated). Then after, a multivariate logistic regression model was used to determine the non-biological factors associated with non-optimal treatments. Finally, the mean of the 1000 estimates was used as the Odds Ratio. Results: In our study, 499 patients (n=48.03%), 273 (26.28%) and 261 (25.12%) had respectively received curative, non-intensive and no treatment. The 3 most influential factors in the choice of treatment modalities were age (61.24% influence), secondary aspect of AML/MDS (21.43%) and presence of severe comorbidities (4.95%). In total, n=460 (44.5%) had received optimal treatment and n=101 (9.8%) received non-optimal treatment. Factors associated with non-optimal treatment were: living in Basse-Normandie (0.63 times) and living in a municipality located between 30 and 100km of an academic hospital. Summary/Conclusion: We show that non-biological factors, in particular geographical disparities have an impact on access to optimal curative treatment. This may be related to geographical disparities in the organisation of the AML care pathway or potentially to the situation of a geographical medical desert, which needs to be studied in more detail.Keywords: Artificial intelligence, Population, Acute myeloid leukemia, Epidemiology
MoreTranslated text
AI Read Science
Must-Reading Tree
Example
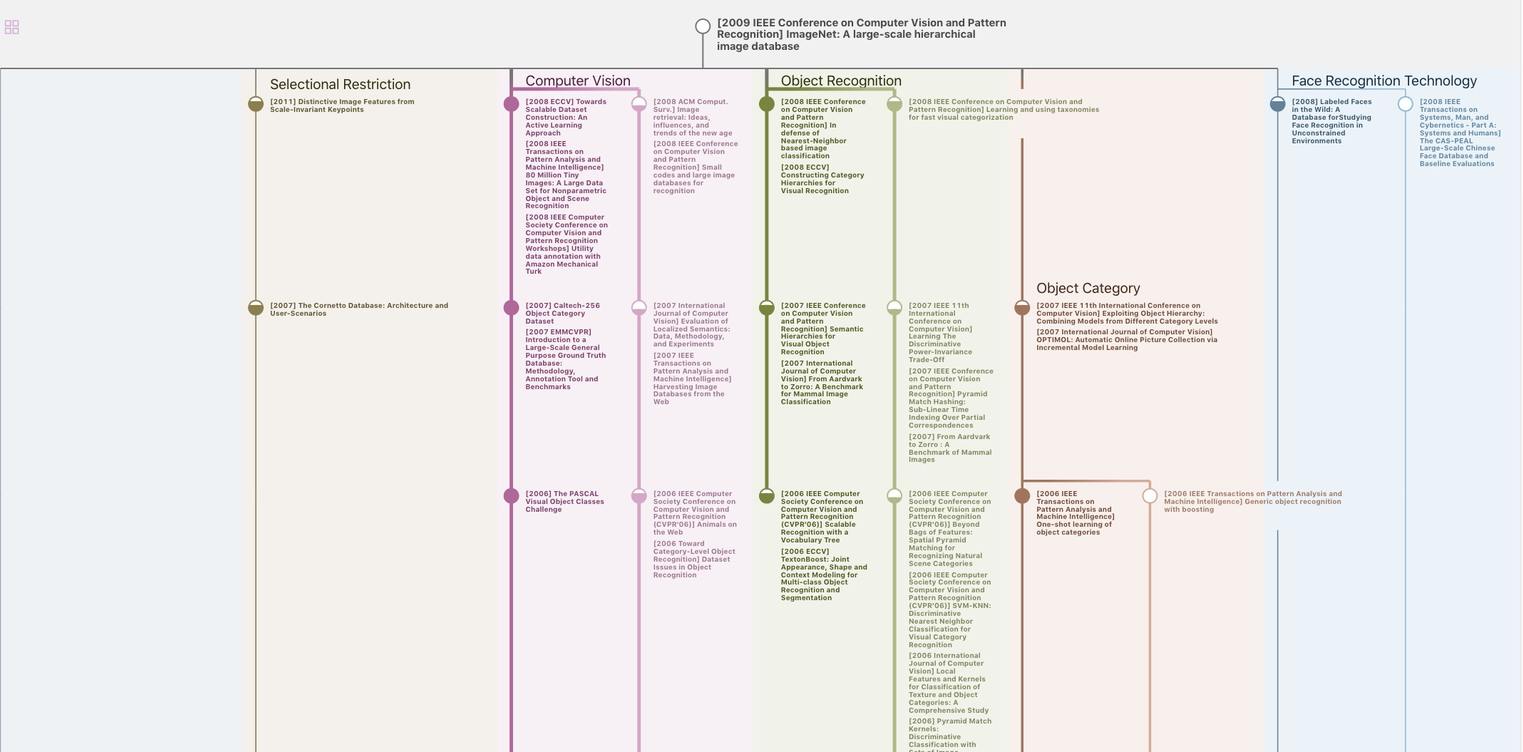
Generate MRT to find the research sequence of this paper
Chat Paper
Summary is being generated by the instructions you defined