Factual or Biased? Predicting Sentence-Level Factuality and Bias of News
CoRR(2023)
Abstract
We present a study on sentence-level factuality and bias of news articles across domains. While prior work in NLP has mainly focused on predicting the factuality of article-level news reporting and political-ideological bias of news media, we investigated the effects of framing bias in factual reporting across domains so as to predict factuality and bias at the sentence level, which may explain more accurately the overall reliability of the entire document. First, we manually produced a large sentence-level annotated dataset, titled FactNews, composed of 6,191 sentences from 100 news stories by three different outlets, resulting in 300 news articles. Further, we studied how biased and factual spans surface in news articles from different media outlets and different domains. Lastly, a baseline model for factual sentence prediction was presented by fine-tuning BERT. We also provide a detailed analysis of data demonstrating the reliability of the annotation and models.
MoreTranslated text
AI Read Science
Must-Reading Tree
Example
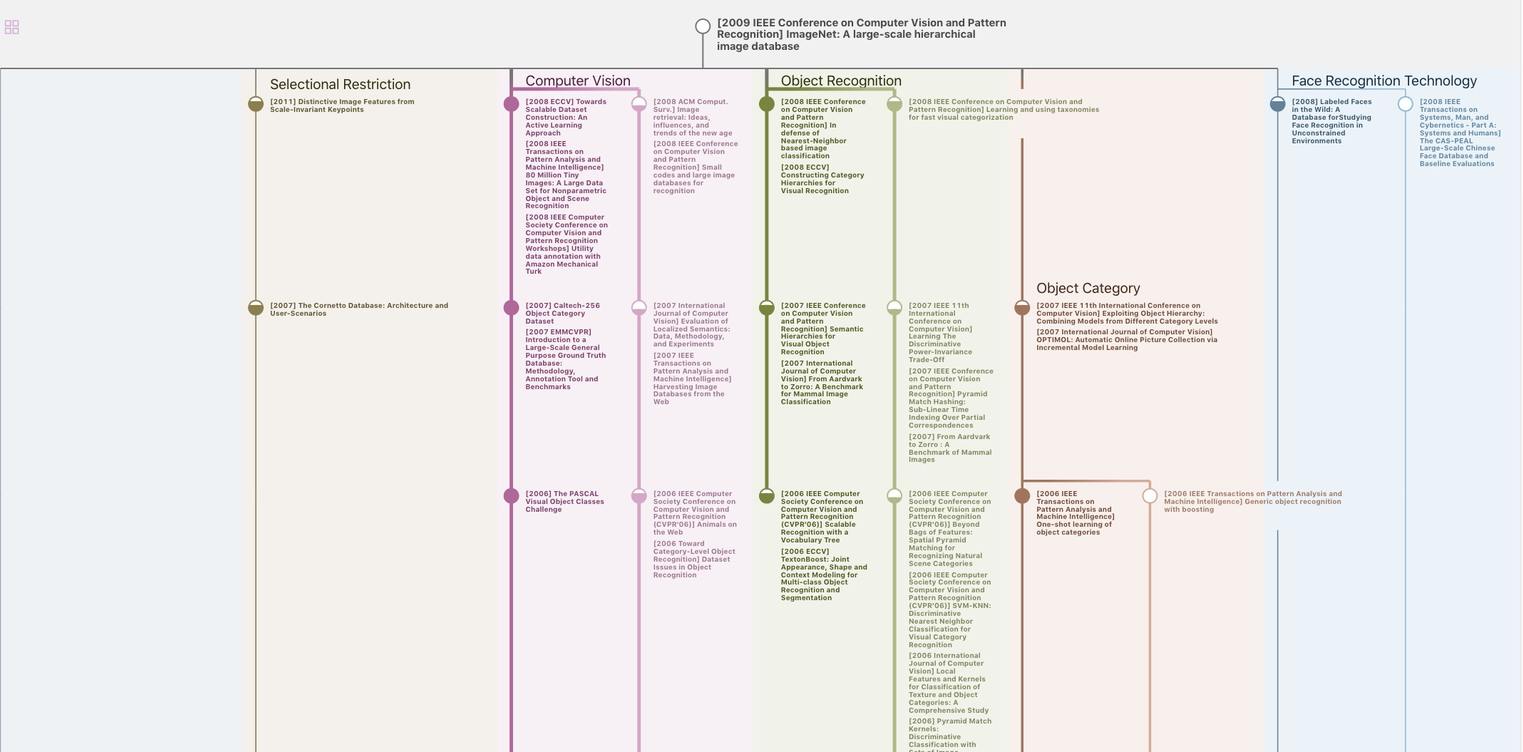
Generate MRT to find the research sequence of this paper
Chat Paper
Summary is being generated by the instructions you defined