Multiple self-controlled case series for large-scale longitudinal observational databases.
BIOMETRICS(2013)
摘要
Characterization of relationships between time-varying drug exposures and adverse events (AEs) related to health outcomes represents the primary objective in postmarketing drug safety surveillance. Such surveillance increasingly utilizes large-scale longitudinal observational databases (LODs), containing time-stamped patient-level medical information including periods of drug exposure and dates of diagnoses for millions of patients. Statistical methods for LODs must confront computational challenges related to the scale of the data, and must also address confounding and other biases that can undermine efforts to estimate effect sizes. Methods that compare on-drug with off-drug periods within patient offer specific advantages over between patient analysis on both counts. To accomplish these aims, we extend the self-controlled case series (SCCS) for LODs. SCCS implicitly controls for fixed multiplicative baseline covariates since each individual acts as their own control. In addition, only exposed cases are required for the analysis, which is computationally advantageous. The standard SCCS approach is usually used to assess single drugs and therefore estimates marginal associations between individual drugs and particular AEs. Such analyses ignore confounding drugs and interactions and have the potential to give misleading results. In order to avoid these difficulties, we propose a regularized multiple SCCS approach that incorporates potentially thousands or more of time-varying confounders such as other drugs. The approach successfully handles the high dimensionality and can provide a sparse solution via an L1 regularizer. We present details of the model and the associated optimization procedure, as well as results of empirical investigations.
更多查看译文
关键词
Big Data,Conditional Poisson regression,Cyclic coordinate descent,Drug safety,Postmarketing surveillance,Regularized regression,Self-controlled case series,Statistical computing
AI 理解论文
溯源树
样例
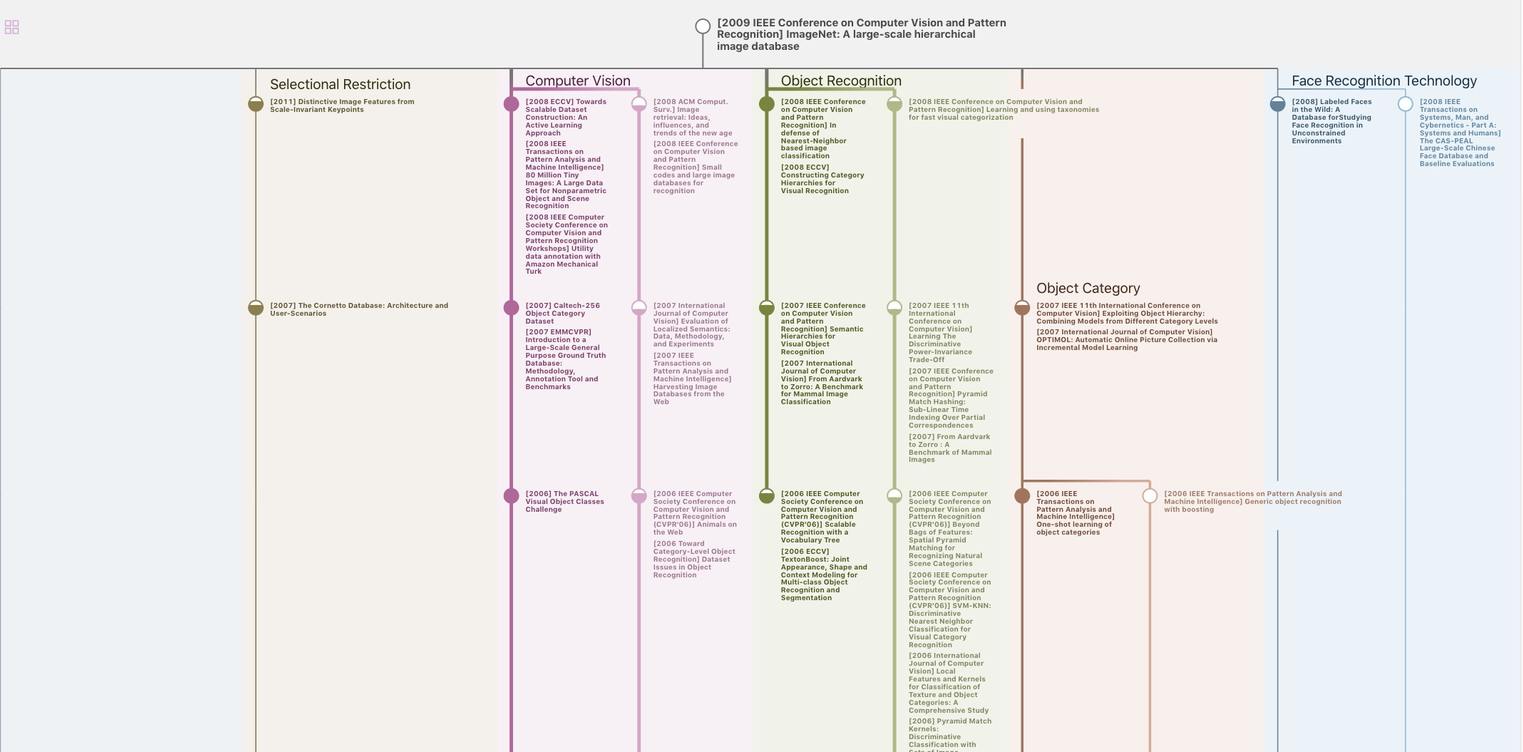
生成溯源树,研究论文发展脉络
Chat Paper
正在生成论文摘要