Improving Activity Recognition Using Temporal Coherence
2013 35TH ANNUAL INTERNATIONAL CONFERENCE OF THE IEEE ENGINEERING IN MEDICINE AND BIOLOGY SOCIETY (EMBC)(2013)
摘要
Assessment of daily physical activity using data from wearable sensors has recently become a prominent research area in the biomedical engineering field and a substantial application for pattern recognition. In this paper, we present an accelerometer-based activity recognition scheme on the basis of a hierarchical structured classifier. A first step consists of distinguishing static activities from dynamic ones in order to extract relevant features for each activity type. Next, a separate classifier is applied to detect more specific activities of the same type. On top of our activity recognition system, we introduce a novel approach to take into account the temporal coherence of activities. Inter-activity transition information is modeled by a directed graph Markov chain. Confidence measures in activity classes are then evaluated from conventional classifier's outputs and coupled with the graph to reinforce activity estimation. Accurate results and significant improvement of activity detection are obtained when applying our system for the recognition of 9 activities for 48 subjects.
更多查看译文
关键词
pattern recognition,accuracy,hidden markov models,markov processes,feature extraction,acceleration,directed graphs,biomedical engineering,accelerometers,physical activity,coherence
AI 理解论文
溯源树
样例
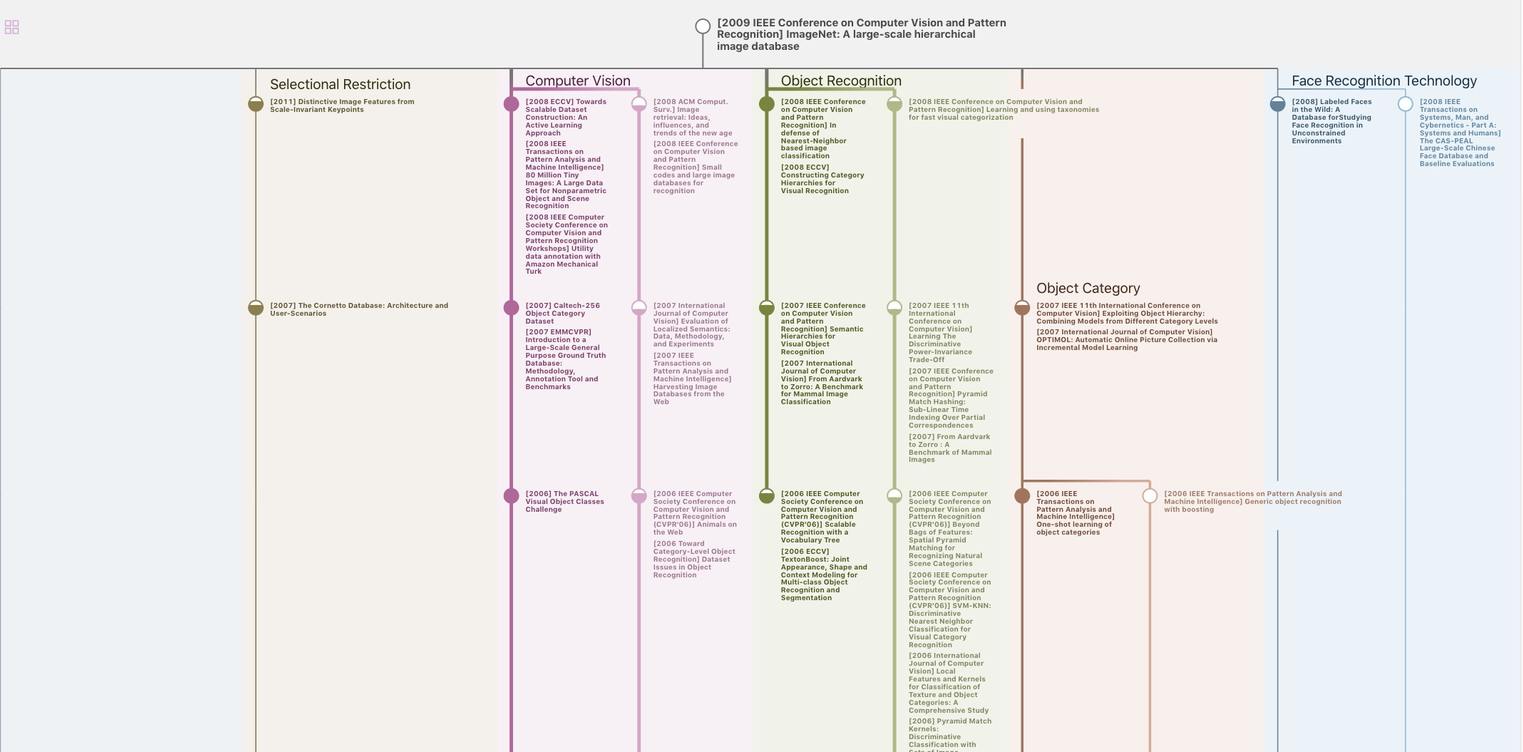
生成溯源树,研究论文发展脉络
Chat Paper
正在生成论文摘要