Compressed ghost imaging based on deep image prior using singular value decomposition
ENGINEERING ANALYSIS WITH BOUNDARY ELEMENTS(2023)
摘要
To improve the low-quality reconstruction of compressed ghost imaging (CGI) caused by non-negative measurement matrix, and avoid the requirements of large amount of training dataset and time-consuming training process, compressed ghost imaging based on deep image prior using singular value decomposition (SVDCGI-DIP) is proposed. The measurement matrix and corresponded measurements are first generated through numerical simulation and then simultaneously optimized with singular value decomposition without the change of optical setup of CGI. Secondly, deep image prior (DIP) method is applied to untrained generative network models to avoiding the long training process, and learned regularization is incorporated to further optimize network parameters to iteratively reconstruct the original image from only a few measurements of an image without training. Numerical experiments verify the superiority of our proposed SVDCGI-DIP method.
更多查看译文
关键词
Compressed ghost imaging, Deep learning, Deep image prior, Singular value decomposition compressed, ghost imaging
AI 理解论文
溯源树
样例
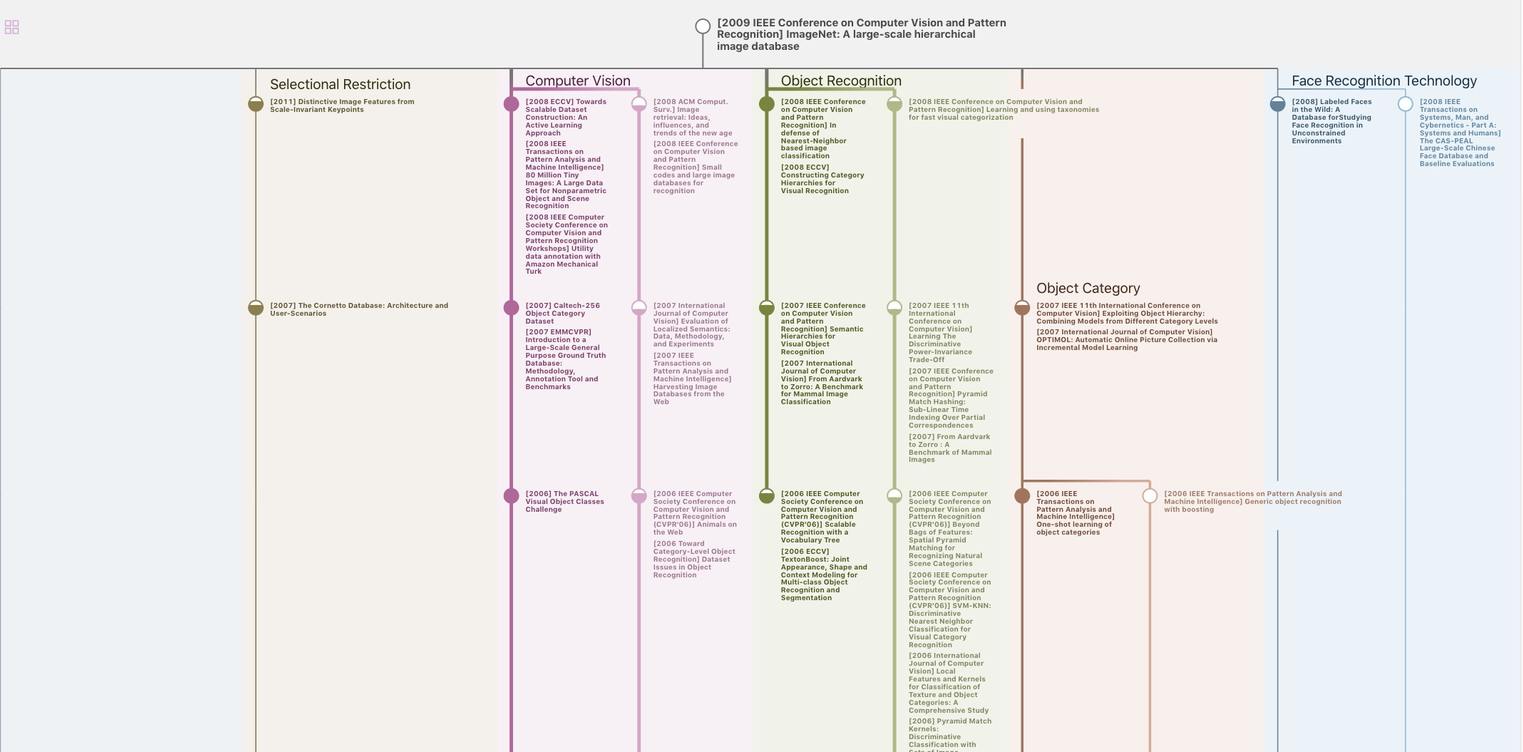
生成溯源树,研究论文发展脉络
Chat Paper
正在生成论文摘要