Deep convolutional autoencoder for urban land use classification using mobile device data
INTERNATIONAL JOURNAL OF GEOGRAPHICAL INFORMATION SCIENCE(2022)
摘要
Mobile phone data can provide insightful location-based information on the interactions between individuals and the urban environment, e.g. urban land-use types. Using mobile phone data, this study aims to recognize citywide land use classes in a highly accurate and timely manner, because land use classes are important for urban planning and urban management. How to extract key and discriminative features from mobile-phone-call time series is an essential problem for urban land-use classification. Considering the high dimensional characteristic of mobile-phone-call time series data, an improved land-use classification framework with deep convolutional autoencoder (DCAE) is proposed to automatically recognize land-use types in urban areas, which are geographical regions clustered based on the similarity of features in waveform and magnitude of mobile-phone-call time series. The method is validated using mobile phone data collected in Wuhan city, China. The experimental result shows that the key and discriminative features in waveform and magnitude can be extracted without human prior knowledge and these features extracted by DCAE can improve the performance to distinguish functionally similar land-use types, such as business and industrial/commercial types.
更多查看译文
关键词
Urban land-use classification,deep convolutional autoencoder model,mobile phone data,call detail records
AI 理解论文
溯源树
样例
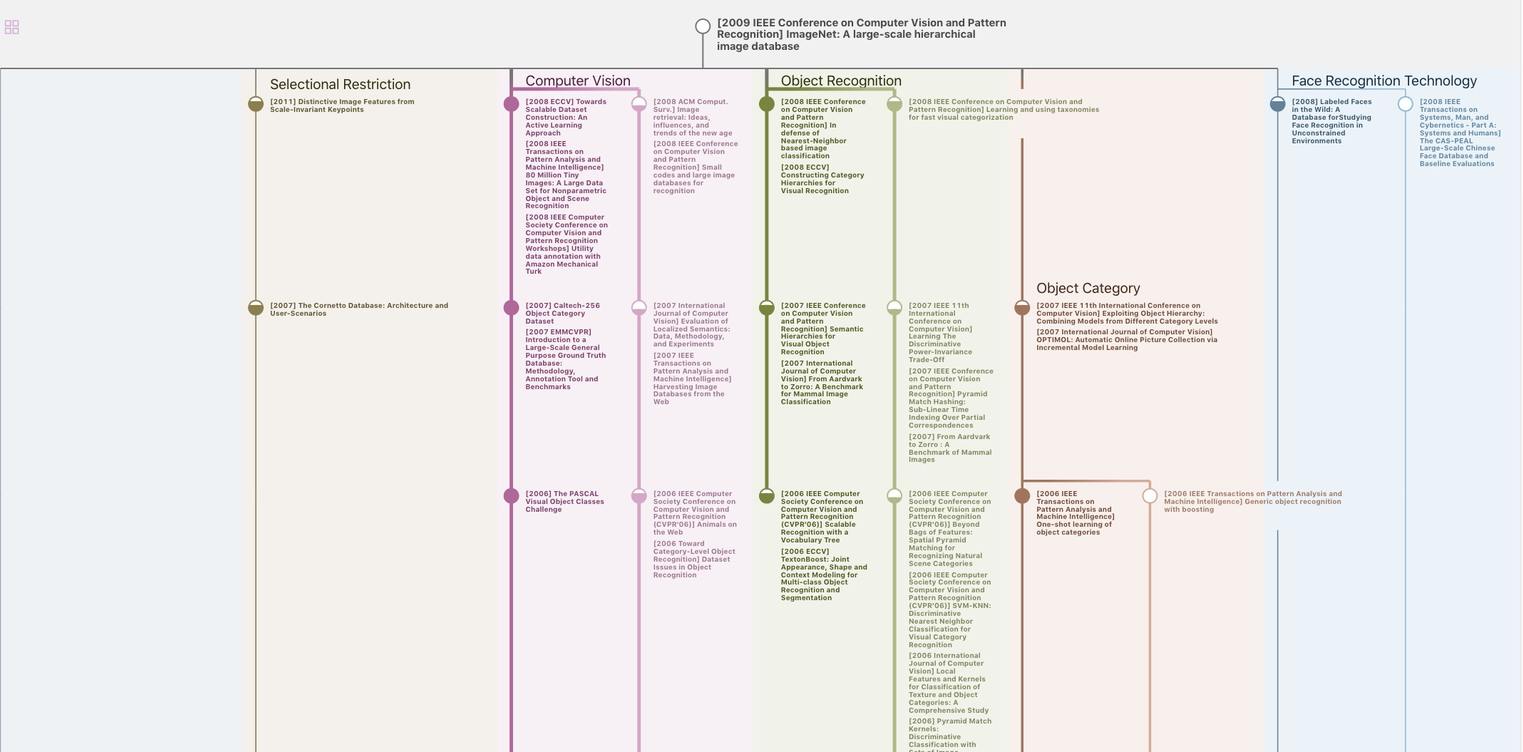
生成溯源树,研究论文发展脉络
Chat Paper
正在生成论文摘要