Diverse Cotraining Makes Strong Semi-Supervised Segmentor
2023 IEEE/CVF International Conference on Computer Vision (ICCV)(2023)
Abstract
Deep co-training has been introduced to semi-supervised segmentation and achieves impressive results, yet few studies have explored the working mechanism behind it. In this work, we revisit the core assumption that supports co-training: multiple compatible and conditionally independent views. By theoretically deriving the generalization upper bound, we prove the prediction similarity between two models negatively impacts the model's generalization ability. However, most current co-training models are tightly coupled together and violate this assumption. Such coupling leads to the homogenization of networks and confirmation bias which consequently limits the performance. To this end, we explore different dimensions of co-training and systematically increase the diversity from the aspects of input domains, different augmentations and model architectures to counteract homogenization. Our Diverse Co-training outperforms the state-of-the-art (SOTA) methods by a large margin across different evaluation protocols on the Pascal and Cityscapes. For example. we achieve the best mIoU of 76.2%, 77.7% and 80.2% on Pascal with only 92, 183 and 366 labeled images, surpassing the previous best results by more than 5%.
MoreTranslated text
Key words
Semi-Supervised Learning,Unsupervised Learning,Meta-Learning,Representation Learning,Semantic Segmentation
AI Read Science
Must-Reading Tree
Example
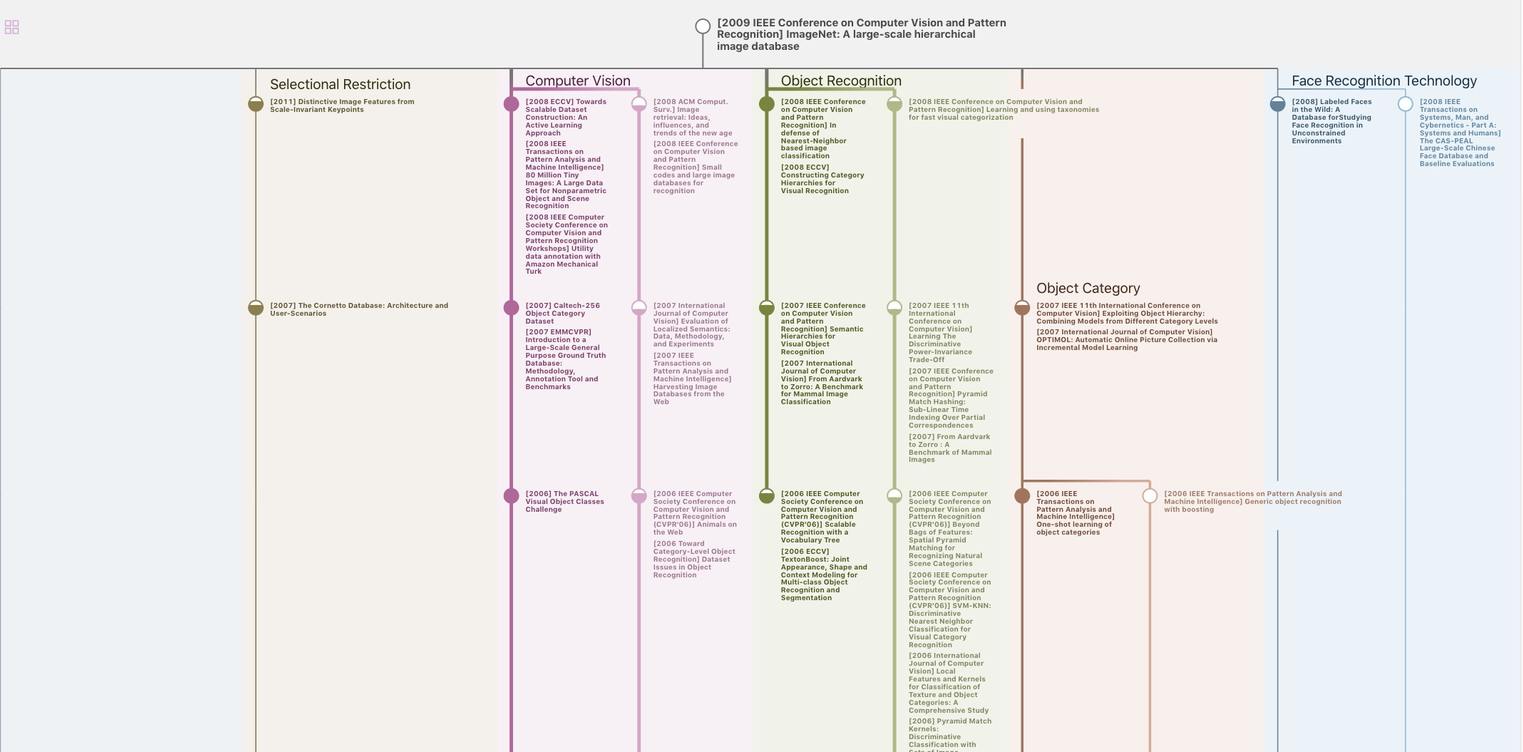
Generate MRT to find the research sequence of this paper
Chat Paper
Summary is being generated by the instructions you defined