Disease Progression Modeling Using Hidden Markov Models
2012 ANNUAL INTERNATIONAL CONFERENCE OF THE IEEE ENGINEERING IN MEDICINE AND BIOLOGY SOCIETY (EMBC)(2012)
摘要
The development of novel treatments for many slowly progressing diseases, such as Alzheimer's disease (AD), is dependent on the ability to monitor and detect changes in disease progression. In some diseases the distinct clinical stages of the disease progress far too slowly to enable a quick evaluation of the efficacy of a given proposed treatment. To help improve the assessment of disease progression, we propose using Hidden Markov Models (HMM's) to model, in a more granular fashion, disease progression as compared to the clinical stages of the disease. Unlike many other applications of Hidden Markov Models, we train our HMM in an unsupervised way and then evaluate how effective the model is at uncovering underlying statistical patterns in disease progression by considering HMM states as disease stages. In this study, we focus on AD and show that our model, when evaluated on the cross validation data, can identify more granular disease stages than the three currently accepted clinical stages of "Normal", "MCI" (Mild Cognitive Impairment), and "AD".
更多查看译文
关键词
hidden markov models,neurophysiology
AI 理解论文
溯源树
样例
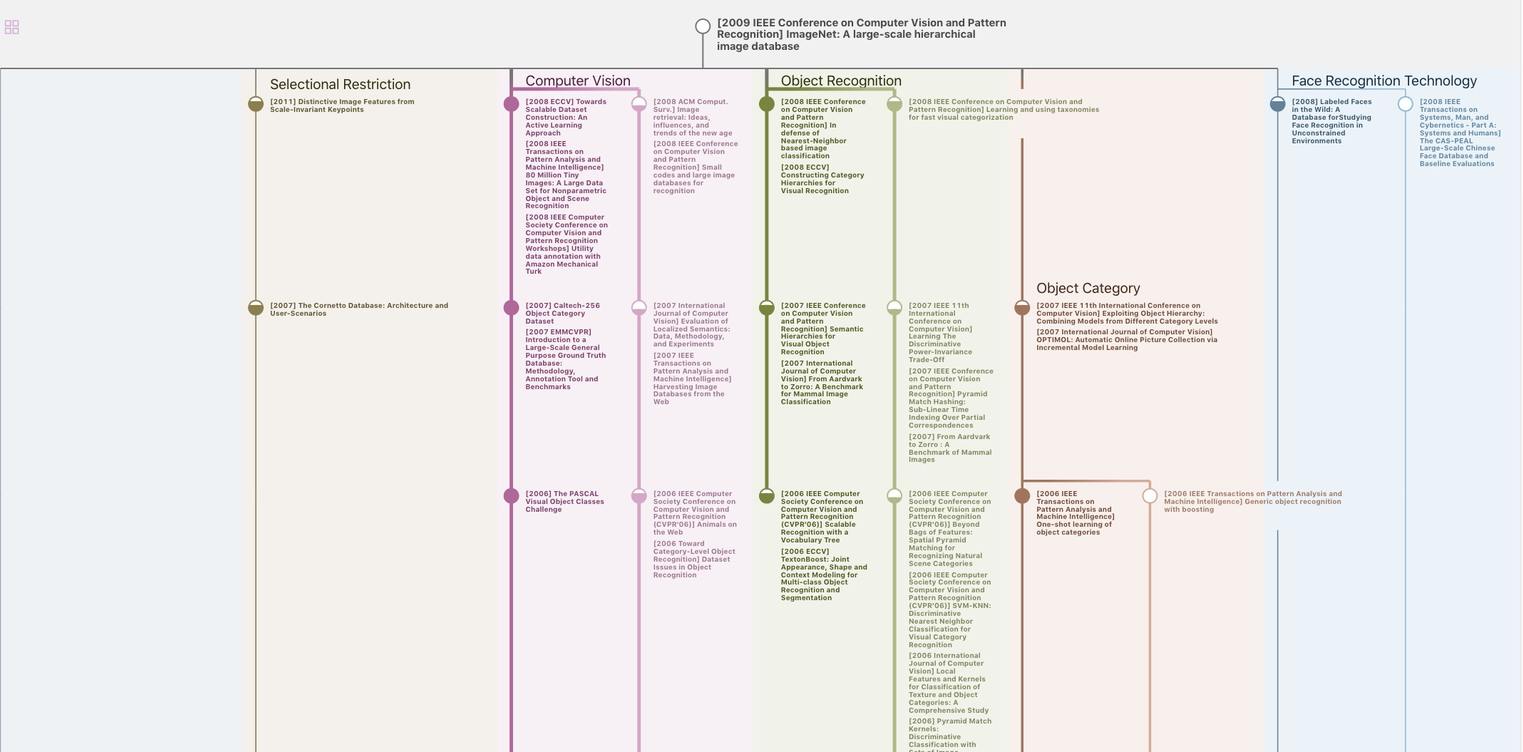
生成溯源树,研究论文发展脉络
Chat Paper
正在生成论文摘要