Parallel Studies on Learning and LTP in the Dentate Gyrus
mag(1988)
摘要
The concept of network remodelling after increased activity as a basic mechanism for the storage of neural representations forms a framework on which most physiological models of memory are built. Long-term potentiation of synaptic transmission in the hippocampus, an example of activity-dependent strengthening of synaptic connexions, has therefore attracted much attention as a plausible candidate for a physiological mechanism subserving mnemonic processes. The original assumption became strengthened as more was known about the characteristics, properties and mechanisms of LTP (e.g. Goddard, 1981; McNaughton, 1983; Voronin, 1983; Bliss and Lynch, 1987). As examples, the induction process, durability, and the cooperative/associative property of LTP, are all in the stream of the predictions one may formulate if LTP is to be viewed as a neural analog of learning. In addition, LTP is accompanied by the kind of biochemical and morphological changes at synaptic elements which have long been proposed as potential sustrates for the storage of information. However, the trace of an event, the engram, cannot be localized at a particular synapse but is rather distributed throughout the brain, synaptic reorganization occuring in multiple relays within the relevant network (Bloch and Laroche, 1984). Indeed synaptic plasticity is not in itself a model of memory, but a functional property upon which memorizing activity could, at least in part, be based. Parallel studies on learning and LTP thus foster a search for a neural change at a focused locus assumed to be part of a widespread, hypothetical network through which the information is encoded and retreived upon reexcitation. It follows that the question addressed is whether LTP at a selected node within the network may represent a valuable candidate for a mechanism upon which synaptic connexions are strenghtened as a result of a learning experience.
更多查看译文
关键词
Dentate Gyrus, Conditioning Session, Population Spike, Perforant Path, Relevant Network
AI 理解论文
溯源树
样例
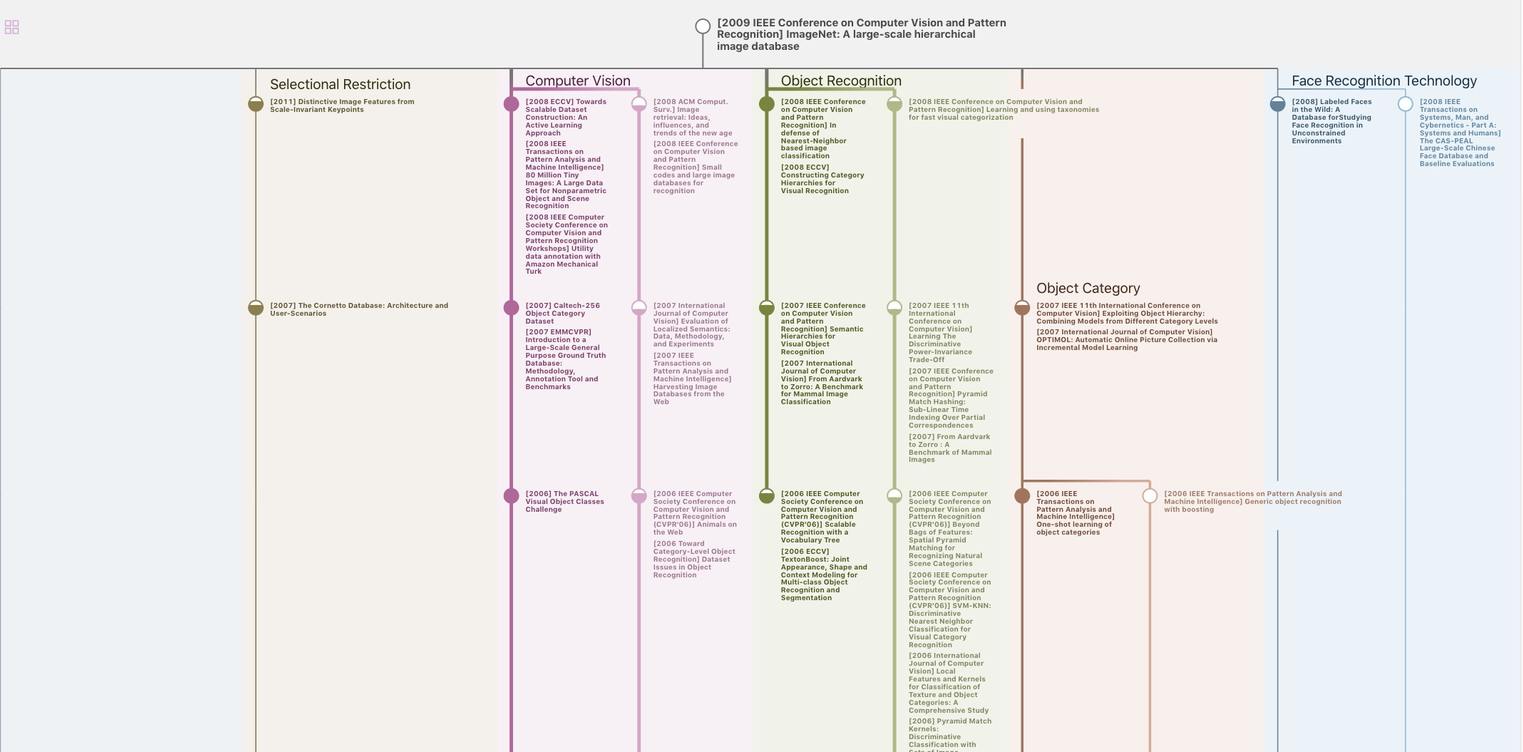
生成溯源树,研究论文发展脉络
Chat Paper
正在生成论文摘要