Monte Carlo conditional inference for log-linear and logistic models: a survey of current methodology
STATISTICAL METHODS IN MEDICAL RESEARCH(2003)
摘要
Nuisance parameters are parameters that are not of immediate interest to the experimenter. For log-linear and logistic models the null distribution of (most) statistics of interest depends on such parameters. Traditionally, nuisance parameters were eliminated by performing inference with respect to the chi-squared limiting distribution of common test statistics. An alternative solution is to eliminate the nuisance parameters by conditioning on their minimal sufficient statistics. The support of the resulting conditional distribution is often intractable, making null probability calculations challenging. An often feasible way to avoid complete enumeration of this support is to approximate conditional probabilities using Monte Carlo methods. In this article we survey recent developments in Monte Carlo conditional analysis for log-linear and logistic models focusing on the algorithms proposed by Booth and Butler,(1) Diaconis and Sturmfels,(2) Smith et al.,(3) and Mehta et al.(4) We illustrate these algorithms with simple motivating examples.
更多查看译文
关键词
logistic models,conditional inference,log-linear
AI 理解论文
溯源树
样例
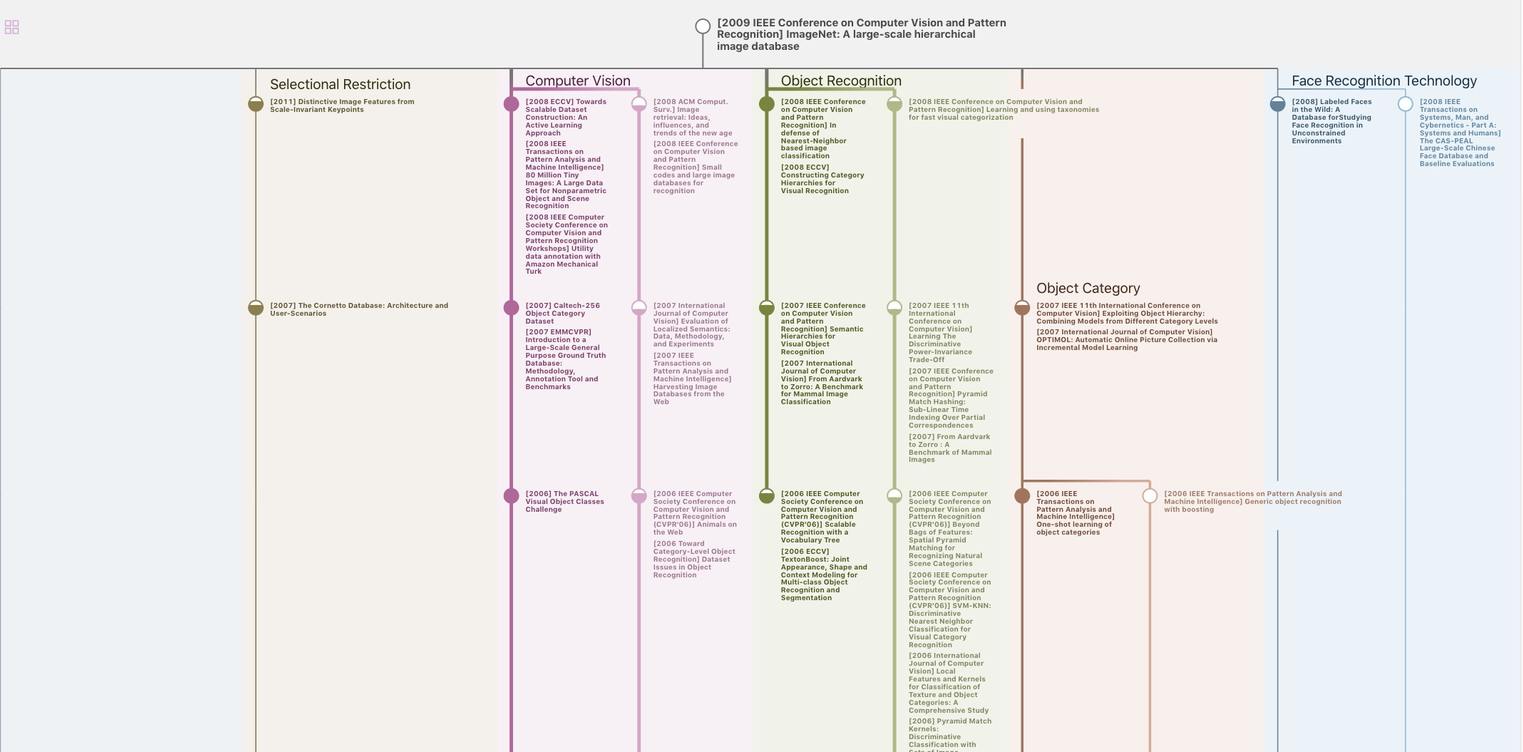
生成溯源树,研究论文发展脉络
Chat Paper
正在生成论文摘要