Continuous non-revisiting genetic algorithm with random search space re-partitioning and one-gene-flip mutation
IEEE Congress on Evolutionary Computation(2010)
摘要
In continuous non-revisiting genetic algorithm (cNrGA), the solution set with different order leads to different density estimation and hence different mutation step size. As a result, the performance of cNrGA depends on the order of the evaluated solutions. In this paper, we propose to remove this dependence by a search space re-partitioning strategy. At each iteration, the strategy re-shuffles the solutions into random order. The re-ordered sequence is then used to construct a new density tree, which leads to a new space partition sets. Afterwards, instead of randomly picking a mutant within a partition, a new adaptive one-gene-flip mutation is applied. Motivated from the fact that the proposed adaptive mutation concerns only small amount of partitions, we propose a new density tree construction algorithm. This algorithm refuses to partition the sub-regions which do not contain any individual to be mutated, which simplifies the tree topology as well as speeds up the construction time. The new cNrGA integrated with the proposed re-partitioning strategy (cNrGA/RP/OGF) is examined on 19 benchmark functions at dimensions ranging from 2 to 40. The simulation results show that cNrGA/RP/OGF is significantly superior to the original cNrGA at most of the test functions. Its average performance is also better than those of six benchmark EAs.
更多查看译文
关键词
tree topology,adaptive mutation,continuous nonrevisiting genetic algorithm,random search space repartitioning,trees (mathematics),one gene flip mutation,search problems,density estimation,density tree construction algorithm,genetic algorithms,iterative methods,topology,estimation,genetic algorithm,benchmark testing,search space,algorithm design and analysis,random search,simulation,construction industry
AI 理解论文
溯源树
样例
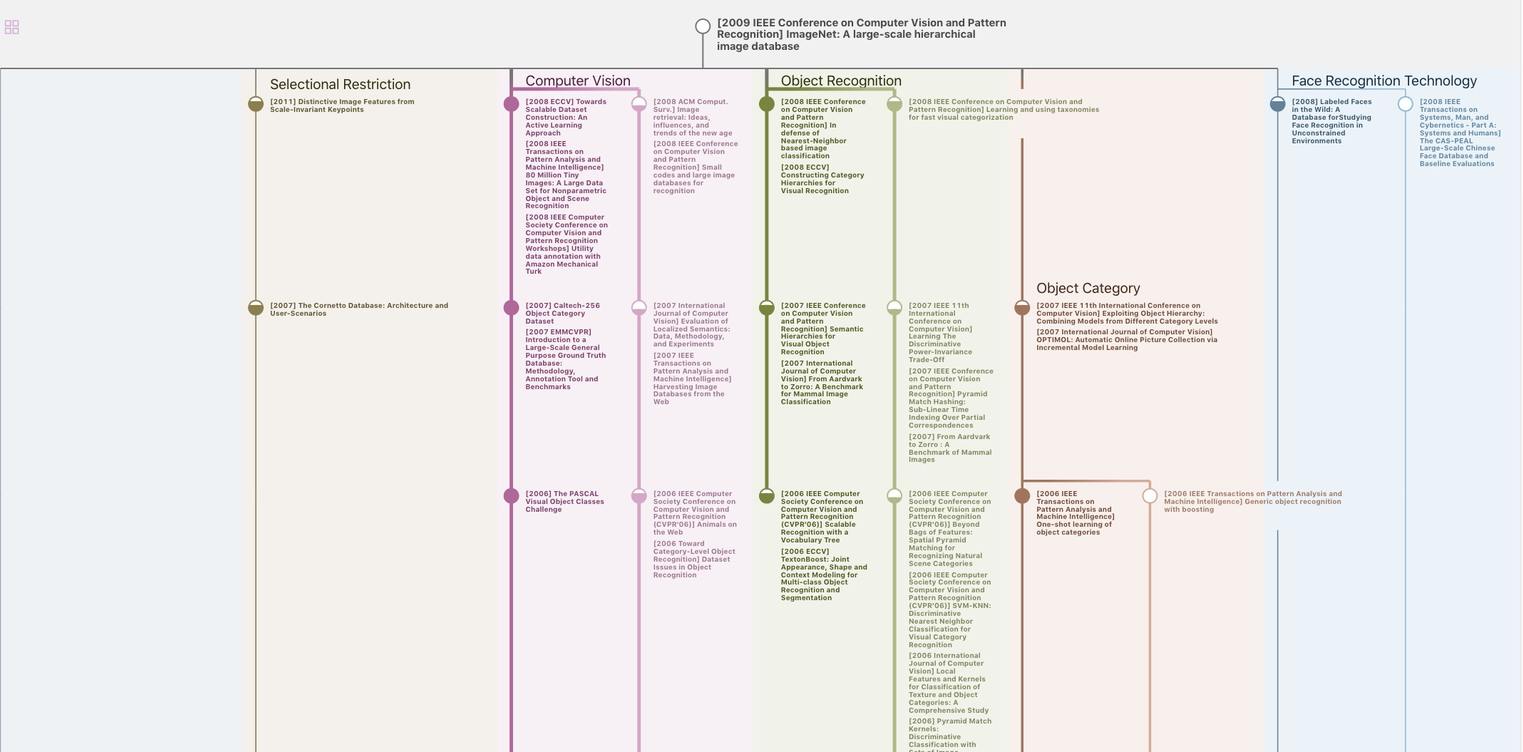
生成溯源树,研究论文发展脉络
Chat Paper
正在生成论文摘要