QEA-QCNN: optimization of quantum convolutional neural network architecture based on quantum evolution
Memetic Computing(2024)
Abstract
Quantum neural network (QNN) is a research orientation that combines quantum computing and machine learning. It has the potential to solve the bottleneck problem of shortage of computing resource in deep learning, and is expected to become the first practical application scheme that demonstrate application level quantum advantages on current Noise Intermediate scale Quantum (NISQ) devices. However, limited by the current scale of NISQ devices, QNNs have fewer quantum bits and quantum circuits cannot be too deep. Currently, there is no clear design strategy for the architecture of QNN. Designing QNN architectures arbitrarily not only has high circuit complexity but also often poor network performance. Similar to classical convolutional neural network, in this paper, a quantum evolution-based optimization algorithm is proposed for design of quantum convolutional neural network (QCNN) architecture. The design of QNN architecture is viewed as a combinatorial optimization problem, and the quantum evolution algorithm is used to adaptively design the QCNN architecture with its global search ability in a large discrete search space. Comprehensive experimental results indicate that the proposed method can effectively reduce the complexity of QCNN circuits, reduce the difficulty of deploying quantum circuits, and further improve the expressibility of QCNN.
MoreTranslated text
Key words
Quantum convolutional neural network,Quantum architecture search,Quantum evolutionary algorithm,Parameterized quantum circuits
AI Read Science
Must-Reading Tree
Example
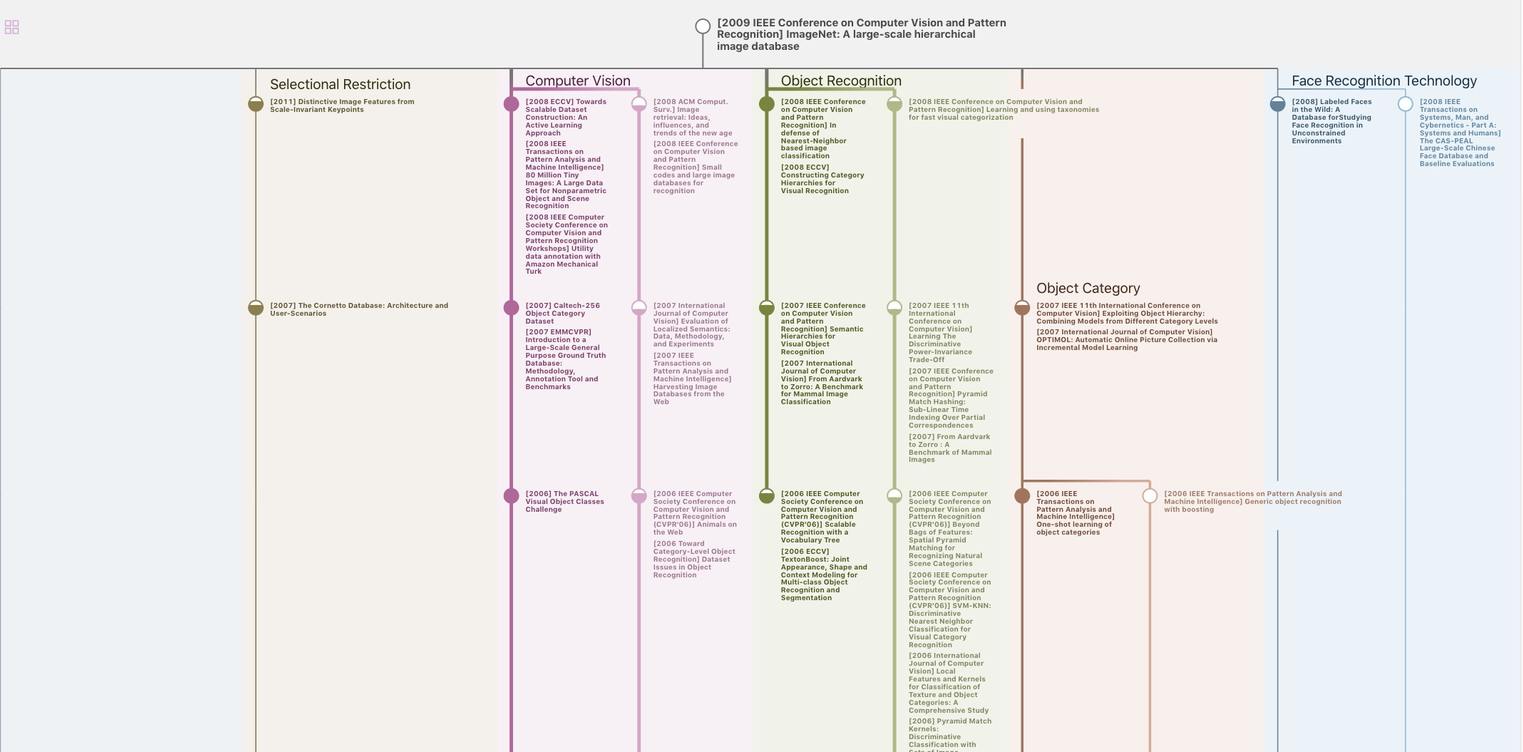
Generate MRT to find the research sequence of this paper
Chat Paper
Summary is being generated by the instructions you defined