Computing Highly Correlated Positions Using Mutual Information And Graph Theory For G Protein-Coupled Receptors
PLOS ONE(2009)
摘要
G protein-coupled receptors (GPCRs) are a superfamily of seven transmembrane-spanning proteins involved in a wide array of physiological functions and are the most common targets of pharmaceuticals. This study aims to identify a cohort or clique of positions that share high mutual information. Using a multiple sequence alignment of the transmembrane (TM) domains, we calculated the mutual information between all inter-TM pairs of aligned positions and ranked the pairs by mutual information. A mutual information graph was constructed with vertices that corresponded to TM positions and edges between vertices were drawn if the mutual information exceeded a threshold of statistical significance. Positions with high degree (i.e. had significant mutual information with a large number of other positions) were found to line a well defined inter-TM ligand binding cavity for class A as well as class C GPCRs. Although the natural ligands of class C receptors bind to their extracellular N-terminal domains, the possibility of modulating their activity through ligands that bind to their helical bundle has been reported. Such positions were not found for class B GPCRs, in agreement with the observation that there are not known ligands that bind within their TM helical bundle. All identified key positions formed a clique within the MI graph of interest. For a subset of class A receptors we also considered the alignment of a portion of the second extracellular loop, and found that the two positions adjacent to the conserved Cys that bridges the loop with the TM3 qualified as key positions. Our algorithm may be useful for localizing topologically conserved regions in other protein families.
更多查看译文
关键词
engineering,crystal structure,g protein coupled receptor,graph theory,medicine,graphs,sequence alignment,ligand binding,entropy,physics,receptor binding,biology,g protein coupled receptors,mutual information,protein family,statistical significance,chemistry,multiple sequence alignment
AI 理解论文
溯源树
样例
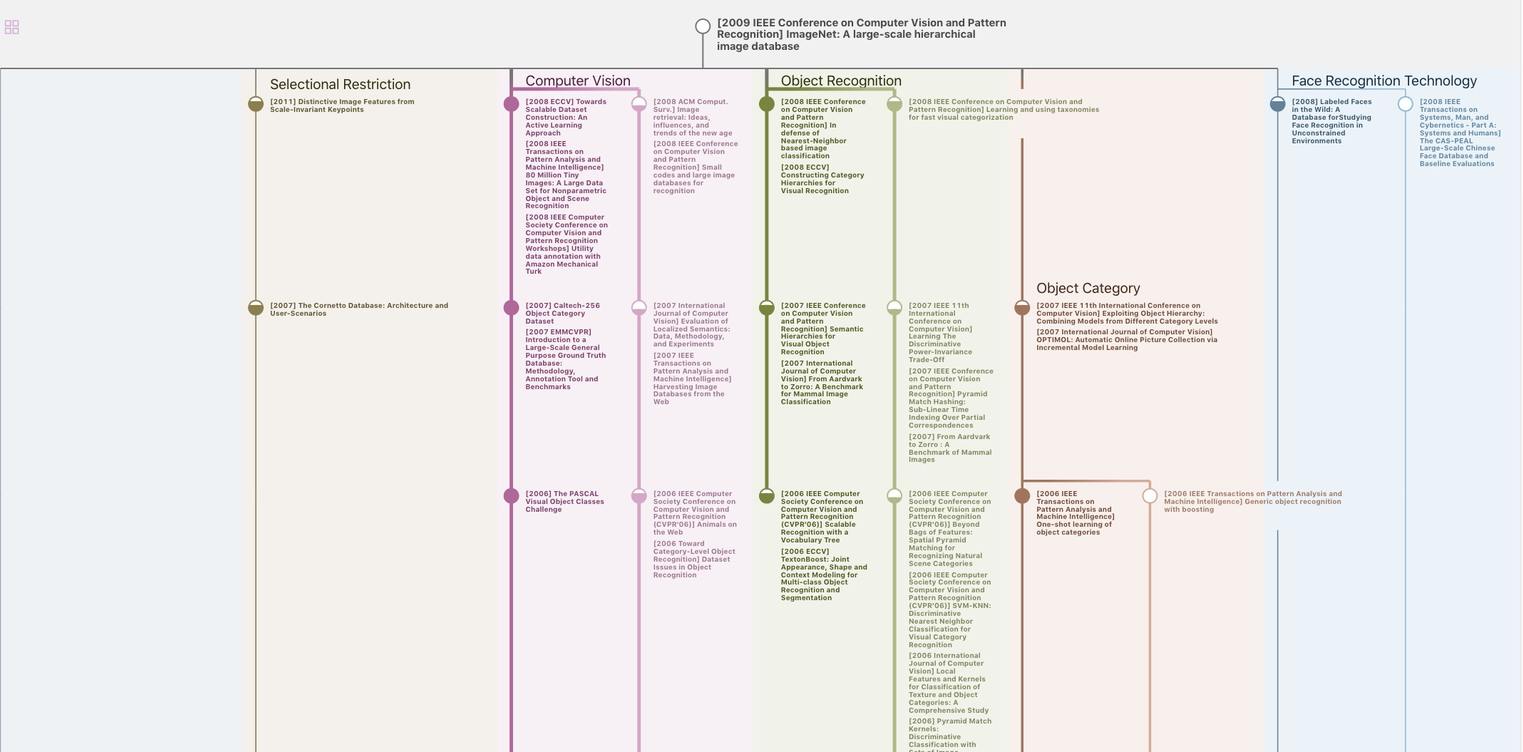
生成溯源树,研究论文发展脉络
Chat Paper
正在生成论文摘要