A Generalizable Causal-Invariance-Driven Segmentation Model for Peripancreatic Vessels.
IEEE transactions on medical imaging(2024)
摘要
Segmenting peripancreatic vessels in CT, including the superior mesenteric artery (SMA), the coeliac artery (CA), and the partial portal venous system (PPVS), is crucial for preoperative resectability analysis in pancreatic cancer. However, the clinical applicability of vessel segmentation methods is impeded by the low generalizability on multi-center data, mainly attributed to the wide variations in image appearance, namely the spurious correlation factor. Therefore, we propose a causal-invariance-driven generalizable segmentation model for peripancreatic vessels. It incorporates interventions at both image and feature levels to guide the model to capture causal information by enforcing consistency across datasets, thus enhancing the generalization performance. Specifically, firstly, a contrast-driven image intervention strategy is proposed to construct image-level interventions by generating images with various contrast-related appearances and seeking invariant causal features. Secondly, the feature intervention strategy is designed, where various patterns of feature bias across different centers are simulated to pursue invariant prediction. The proposed model achieved high DSC scores (79.69%, 82.62%, and 83.10%) for the three vessels on a cross-validation set containing 134 cases. Its generalizability was further confirmed on three independent test sets of 233 cases. Overall, the proposed method provides an accurate and generalizable segmentation model for peripancreatic vessels and offers a promising paradigm for increasing the generalizability of segmentation models from a causality perspective. Our source codes will be released at https://github.com/SJTUBME-QianLab/PC_VesselSeg.
更多查看译文
关键词
Pancreatic cancer,peripancreatic vessel segmentation,generalization,causal learning
AI 理解论文
溯源树
样例
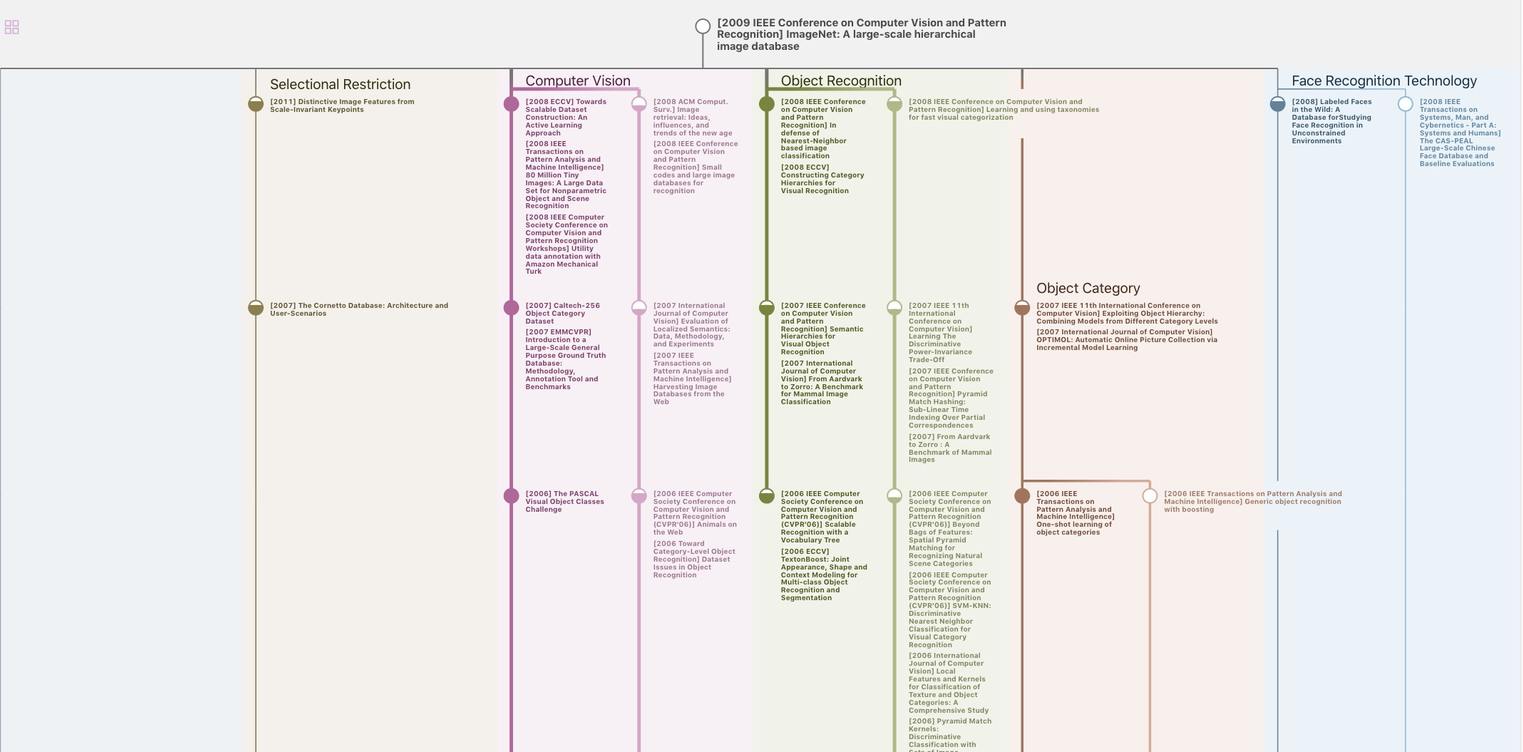
生成溯源树,研究论文发展脉络
Chat Paper
正在生成论文摘要