A heuristic representation learning based on evidential memberships: Case study of UCI-SPECTF
International Journal of Approximate Reasoning(2020)
摘要
The diagnosed features (samples) with multiple attributes of medical images always demand experts to reveal insight. Up to today, machine learning often cannot be a helpful expert. The reason lies in lacking evidential granules carrying knowledge and evidence for inferential learning. The shortage slows down representation learning which aims at discovering expressions for featuring concepts. Therefore, this paper proposes evidential memberships carrying preferential relevance to build a heuristic representation learning. Empirically, it solves local features and global representations with maximum coverage under challenges of shallow bury. For illustration, it is implemented on the testing data set of UCI-SPECTF.
更多查看译文
关键词
Machine learning (ML),Granules,Information aggregation (IA),Heuristic representation learning (HRL),Evidential membership (EM)
AI 理解论文
溯源树
样例
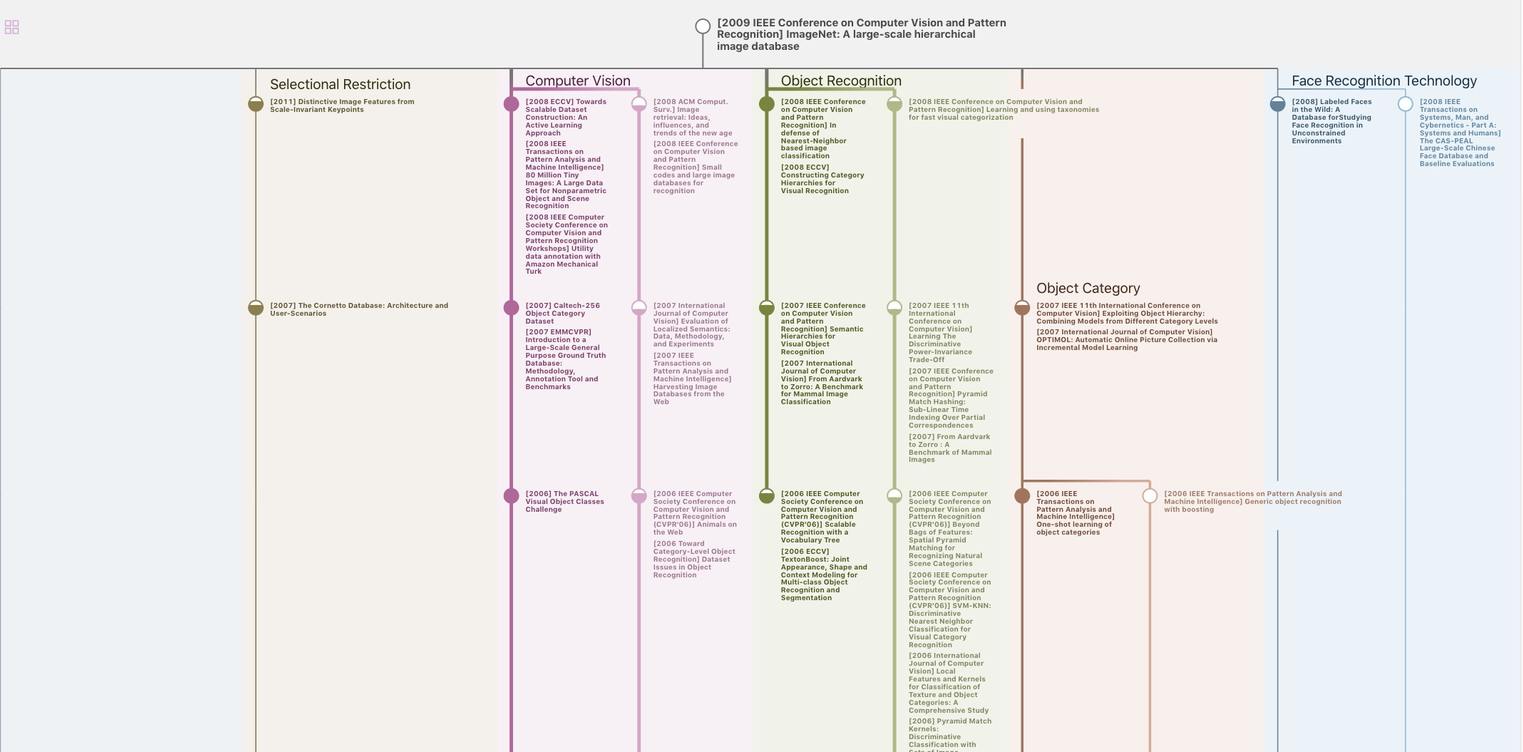
生成溯源树,研究论文发展脉络
Chat Paper
正在生成论文摘要