From in vitro EC₅₀ to in vivo dose-response for antiretrovirals using an HIV disease model. Part I: a framework.
Journal of pharmacokinetics and pharmacodynamics(2012)
Abstract
Plasma drug concentrations and human immunodeficiency virus (HIV)-1 ribonucleic acid (RNA) data from short-term monotherapy trials in antiretroviral (ARV) naïve/experienced HIV-infected patients for one drug (i.e., lead case, a representative drug for a given class) from four distinct classes of ARV drugs [non-nucleoside reverse transcriptase inhibitors (NNRTIs); nucleotide reverse transcriptase inhibitors (NtRTIs); co-receptor antagonists (CRAs); and integrase strand transfer inhibitors (INSTIs)] were obtained. For each drug (the lead case; for example, etravirine for NNRTIs), the pharmacokinetic (PK)-pharmacodynamic (PD) disease model was used to estimate the in vivo IC₅₀ value based on the relationship between plasma drug concentrations and HIV-1 RNA decline. The disease model was a mechanistic viral dynamic model with the addition of a transduction delay for HIV-1 RNA decay. The model characterized the observed time-course of plasma drug concentrations and HIV-1 RNA in each lead case trial reasonably well. The estimated viral dynamic parameters were in good agreement with the literature values. The scaling factor (SF) was calculated as a ratio using the estimated in vivo IC₅₀ value and protein binding corrected EC₅₀ value for the lead case. For NNRTIs, the calculated SF was found to be 9.47 based on etravirine analysis. Using the same approach, the SF for CRAs (maraviroc), INSTIs (GSK1349572), and NtRTIs (tenofovir) were 4.35, 1.63, and 0.27. The SF is reflective of the uncertainty between in vitro measurement of drug potency and the specific model-based drug potency parameter (IC₅₀). The use of SF and PK-PD disease models can be a valuable tool to predict dose-response of NMEs and support rational dose selection for monotherapy trials.
MoreTranslated text
Key words
HIV, EC50, IC50, Pharmacokinetics, Pharmacodynamics, Viral dynamics, Dose selection, Model based drug development
AI Read Science
Must-Reading Tree
Example
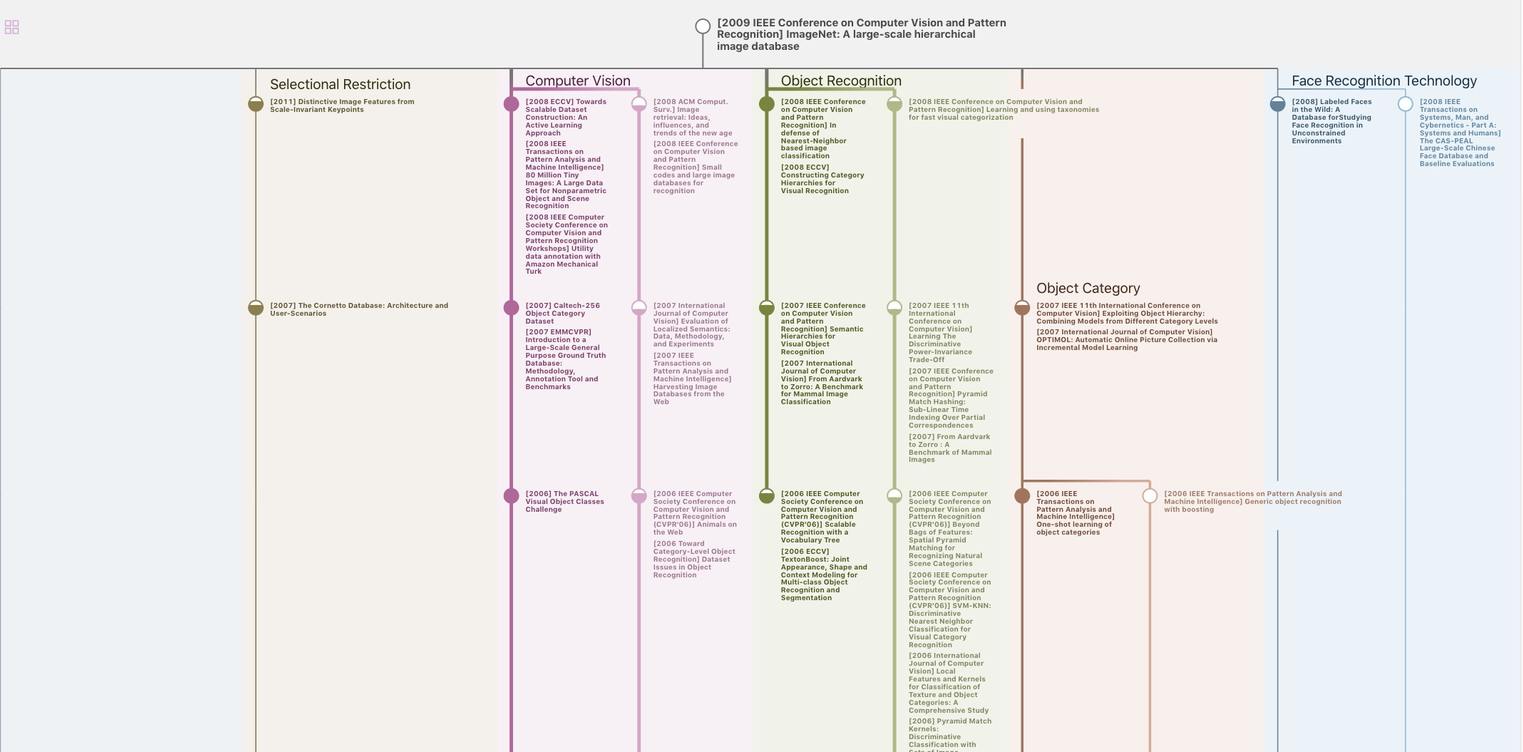
Generate MRT to find the research sequence of this paper
Chat Paper
Summary is being generated by the instructions you defined