Efficient LLM Jailbreak via Adaptive Dense-to-sparse Constrained Optimization
CoRR(2024)
摘要
Recent research indicates that large language models (LLMs) are susceptible
to jailbreaking attacks that can generate harmful content. This paper
introduces a novel token-level attack method, Adaptive Dense-to-Sparse
Constrained Optimization (ADC), which effectively jailbreaks several
open-source LLMs. Our approach relaxes the discrete jailbreak optimization into
a continuous optimization and progressively increases the sparsity of the
optimizing vectors. Consequently, our method effectively bridges the gap
between discrete and continuous space optimization. Experimental results
demonstrate that our method is more effective and efficient than existing
token-level methods. On Harmbench, our method achieves state of the art attack
success rate on seven out of eight LLMs. Code will be made available. Trigger
Warning: This paper contains model behavior that can be offensive in nature.
更多查看译文
AI 理解论文
溯源树
样例
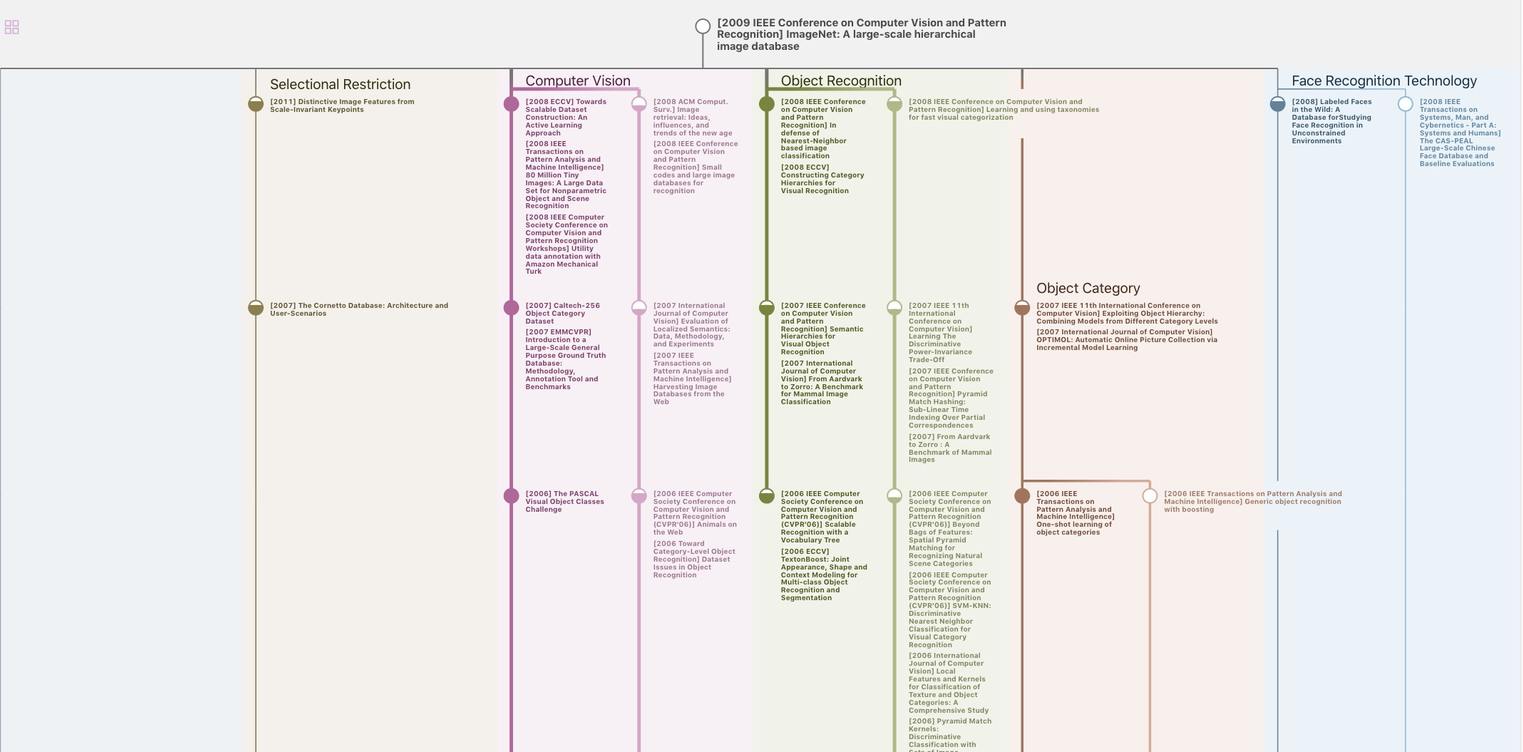
生成溯源树,研究论文发展脉络
Chat Paper
正在生成论文摘要