A Remaining Useful Life Prediction Method for Lithium-ion Battery Based on Temporal Transformer Network
Procedia Computer Science(2023)
Abstract
The remaining useful life prediction is significant for Lithium-ion batteries to ensure safety and reliability. Due to the advantages of handling time sequence data, recurrent neural network based methods have achieved impressive performance on RUL prediction. However, most of these methods develop the RUL prediction model without considering the operating time of the battery, which is an important factor on capacity degradation. Therefore, this paper proposed a Temporal Transformer Network (TTN) for RUL of Lithium-ion batteries. The proposed method combines the self-attention mechanism of the Transformer Network with Denoising Autoencoder to implement the noise of raw data. More importantly, the proposed method designs a temporal encoding layer to introduce the operating time to the input of RUL prediction model. The performance of the proposed method is evaluated on two frequently used battery datasets. The proposed method achieves the best result compared with other frequently used RUL prediction methods.
MoreTranslated text
Key words
useful life prediction method,lithium-ion prediction battery
AI Read Science
Must-Reading Tree
Example
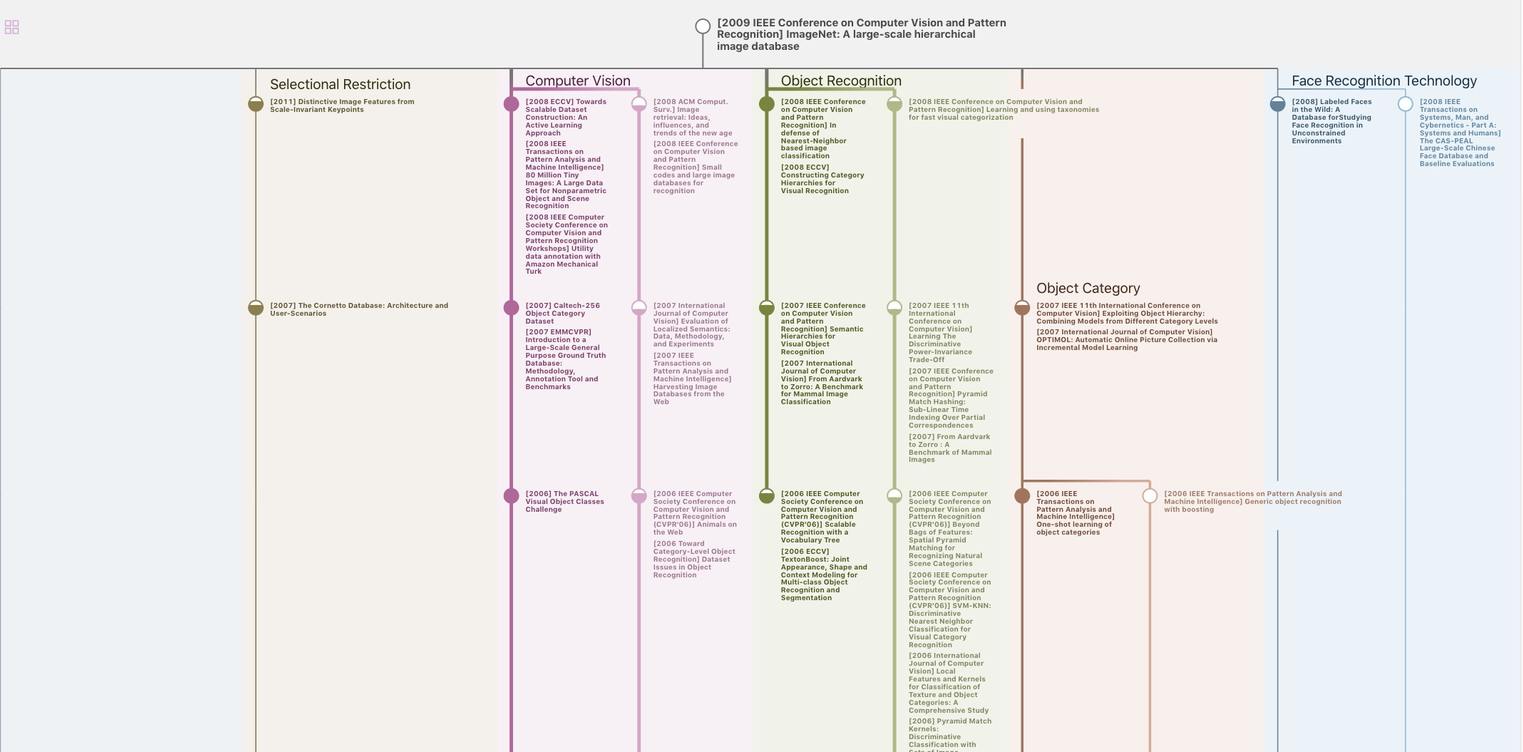
Generate MRT to find the research sequence of this paper
Chat Paper
Summary is being generated by the instructions you defined