The denoising of Monte Carlo dose distributions using convolution superposition calculations.
PHYSICS IN MEDICINE AND BIOLOGY(2007)
摘要
Monte Carlo ( MC) dose calculations can be accurate but are also computationally intensive. In contrast, convolution superposition ( CS) offers faster and smoother results but bymaking approximations. We investigatedMC denoising techniques, which use available convolution superposition results and new noise filtering methods to guide and accelerateMC calculations. Two main approaches were developed to combine CS information with MC denoising. In the first approach, the denoising result is iteratively updated by adding the denoised residual difference between the result and theMC image. Multi- scale methods were used ( wavelets or contourlets) for denoising the residual. The iterations are initialized by the CS data. In the second approach, we used a frequency splitting technique by quadrature filtering to combine low frequency components derived from MC simulations with high frequency components derived from CS components. The rationale is to take the scattering tails as well as dose levels in the high- dose region from the MC calculations, which presumably more accurately incorporates scatter; high- frequency details are taken from CS calculations. 3D Butterworth filters were used to design the quadrature filters. The methods were demonstrated using anonymized clinical lung and head and neck cases. The MC dose distributions were calculated by the open- source dose planning method MC code with varying noise levels. Our results indicate that the frequency- splitting technique for incorporating CS- guided MC denoising is promising in terms of computational efficiency and noise reduction.
更多查看译文
关键词
noise reduction,monte carlo,high frequency,low frequency
AI 理解论文
溯源树
样例
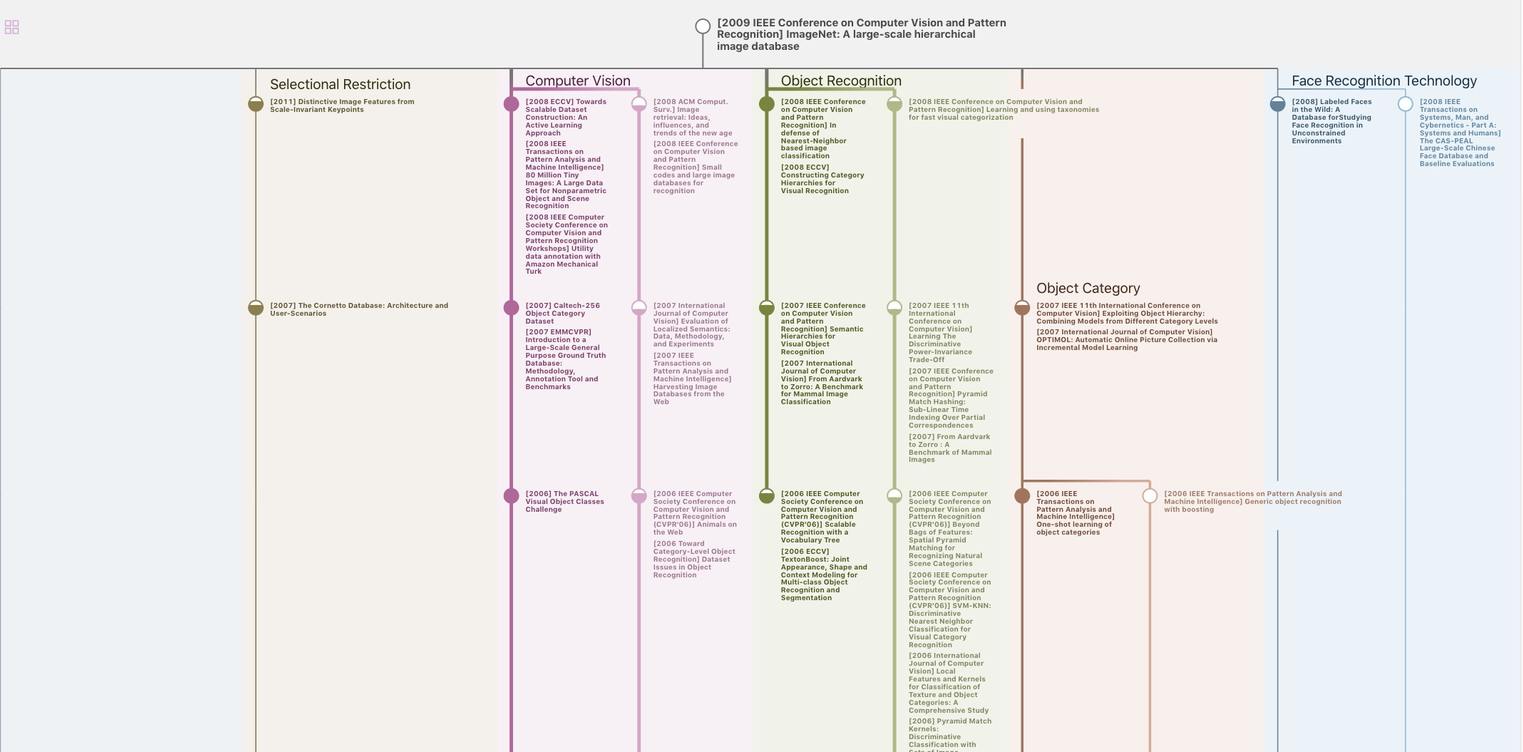
生成溯源树,研究论文发展脉络
Chat Paper
正在生成论文摘要