Improved Contraction-Expansion Subspace Ensemble for High-Dimensional Imbalanced Data Classification
IEEE Transactions on Knowledge and Data Engineering(2024)
摘要
Imbalanced data biases the classifier towards the majority class. Accompanied with high-dimensional characteristics, classification performance is further degraded. Existing researches for skewed data mainly involve resampling, cost-sensitive learning, and classifier ensemble. However, these approaches have some limitations: 1) resampling suffers from noisy and redundant features in high-dimensional skewed data; 2) cost-sensitive learning is hard to construct an optimal cost matrix for sample misclassification; 3) ensemble with random feature subspace easily leads to information loss; 4) ensemble with sample subspace on small-size data easily leads to insufficient description of sample space and suffers from negative impacts of high-dimensional data. This paper proposes an improved contraction-expansion subspace ensemble (ICESE) for high-dimensional imbalanced data classification. First, a contraction-expansion subspace optimization (CESO) is designed to perform subspace selection and transformation, which is beneficial for enhancing the discrimination and diversity of subspace. Then, to strengthen classification capabilities, a CESO-based multilayer optimization structure is developed to construct the improved subspace. Finally, to mitigate the effects of skewed data, ICESE performs a resampling scheme on the improved subspace for constructing a rebalanced subset to base classifier. Experimental results on 24 high-dimensional imbalanced data sets demonstrate that our ICESE outperforms different mainstream ensemble systems in terms of F-score and G-mean.
更多查看译文
关键词
Ensemble learning,subspace optimization,class imbalance,high-dimensional data,classification
AI 理解论文
溯源树
样例
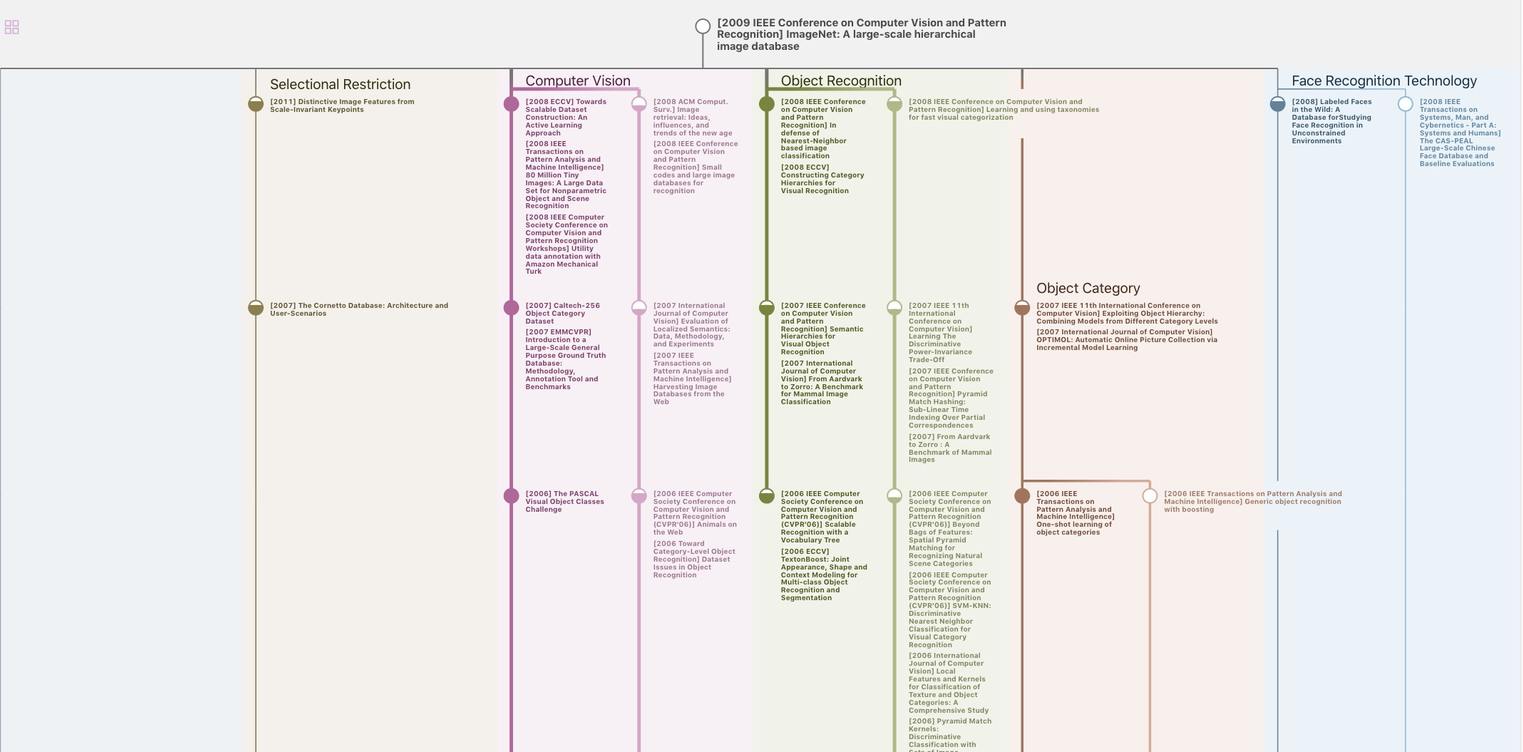
生成溯源树,研究论文发展脉络
Chat Paper
正在生成论文摘要