Towards a Common Understanding of Contributing Factors for Cross-Lingual Transfer in Multilingual Language Models: A Review
PROCEEDINGS OF THE 61ST ANNUAL MEETING OF THE ASSOCIATION FOR COMPUTATIONAL LINGUISTICS, ACL 2023, VOL 1(2023)
Abstract
In recent years, pre-trained Multilingual Language Models (MLLMs) have shown a strong ability to transfer knowledge across different languages. However, given that the aspiration for such an ability has not been explicitly incorporated in the design of the majority of MLLMs, it is challenging to obtain a unique and straightforward explanation for its emergence. In this review paper, we survey literature that investigates different factors contributing to the capacity of MLLMs to perform zero-shot cross-lingual transfer and subsequently outline and discuss these factors in detail. To enhance the structure of this review and to facilitate consolidation with future studies, we identify five categories of such factors. In addition to providing a summary of empirical evidence from past studies, we identify consensuses among studies with consistent findings and resolve conflicts among contradictory ones. Our work contextualizes and unifies existing research streams which aim at explaining the cross-lingual potential of MLLMs. This review provides, first, an aligned reference point for future research and, second, guidance for a better-informed and more efficient way of leveraging the cross-lingual capacity of MLLMs.
MoreTranslated text
Key words
multilingual language models,transfer,cross-lingual
AI Read Science
Must-Reading Tree
Example
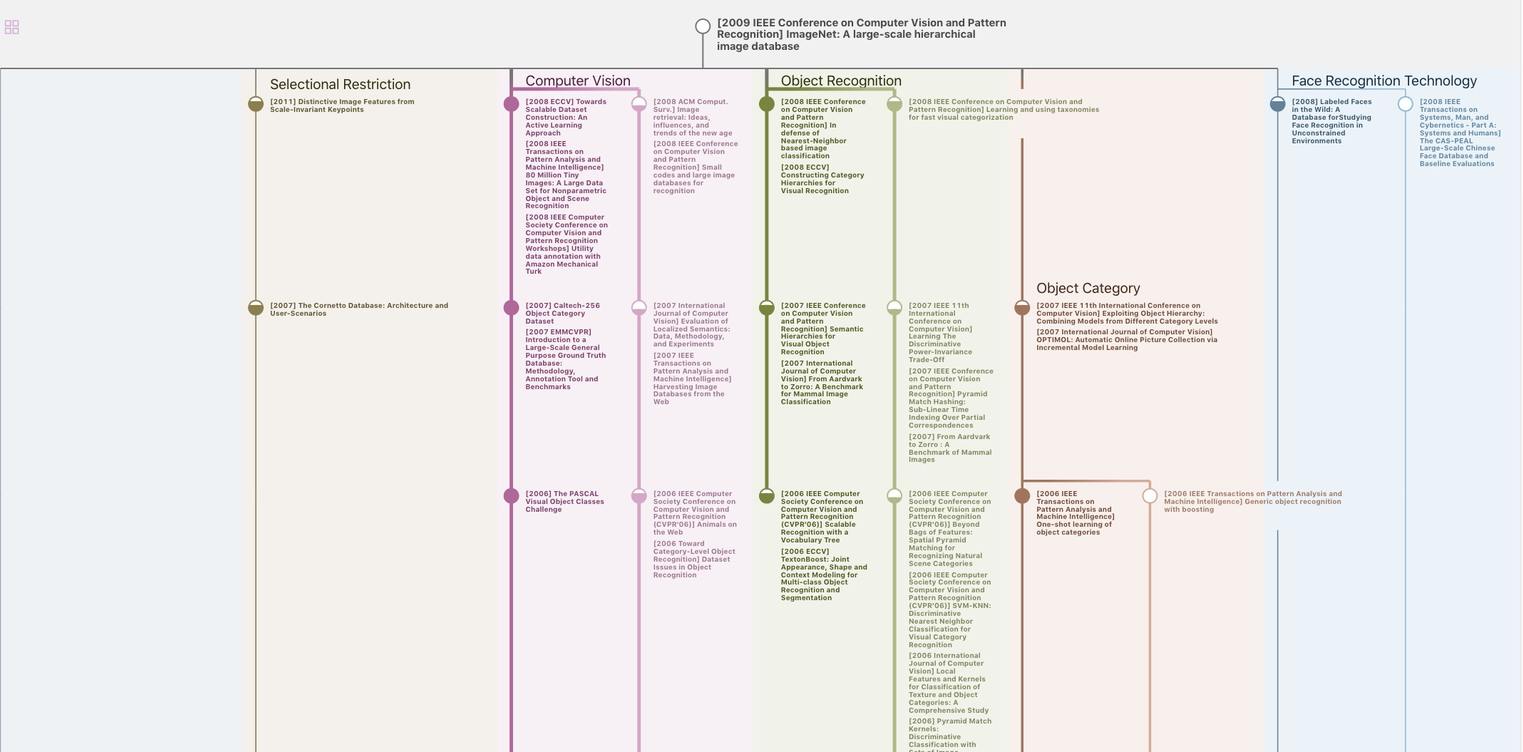
Generate MRT to find the research sequence of this paper
Chat Paper
Summary is being generated by the instructions you defined