Augmenting Low-Resource Text Classification with Graph-Grounded Pre-training and Prompting
PROCEEDINGS OF THE 46TH INTERNATIONAL ACM SIGIR CONFERENCE ON RESEARCH AND DEVELOPMENT IN INFORMATION RETRIEVAL, SIGIR 2023(2023)
Abstract
Text classification is a fundamental problem in information retrieval with many real-world applications, such as predicting the topics of online articles and the categories of e-commerce product descriptions. However, low-resource text classification, with few or no labeled samples, poses a serious concern for supervised learning. Meanwhile, many text data are inherently grounded on a network structure, such as a hyperlink/citation network for online articles, and a user-item purchase network for e-commerce products. These graph structures capture rich semantic relationships, which can potentially augment low-resource text classification. In this paper, we propose a novel model called Graph-Grounded Pre-training and Prompting (G2P2) to address low-resource text classification in a two-pronged approach. During pre-training, we propose three graph interaction-based contrastive strategies to jointly pre-train a graph-text model; during downstream classification, we explore prompting for the jointly pre-trained model to achieve low-resource classification. Extensive experiments on four real-world datasets demonstrate the strength of G2P2 in zero- and few-shot low-resource text classification tasks.
MoreTranslated text
Key words
Text classification,graph neural networks,low-resource learning,pre-training,prompt-tuning
AI Read Science
Must-Reading Tree
Example
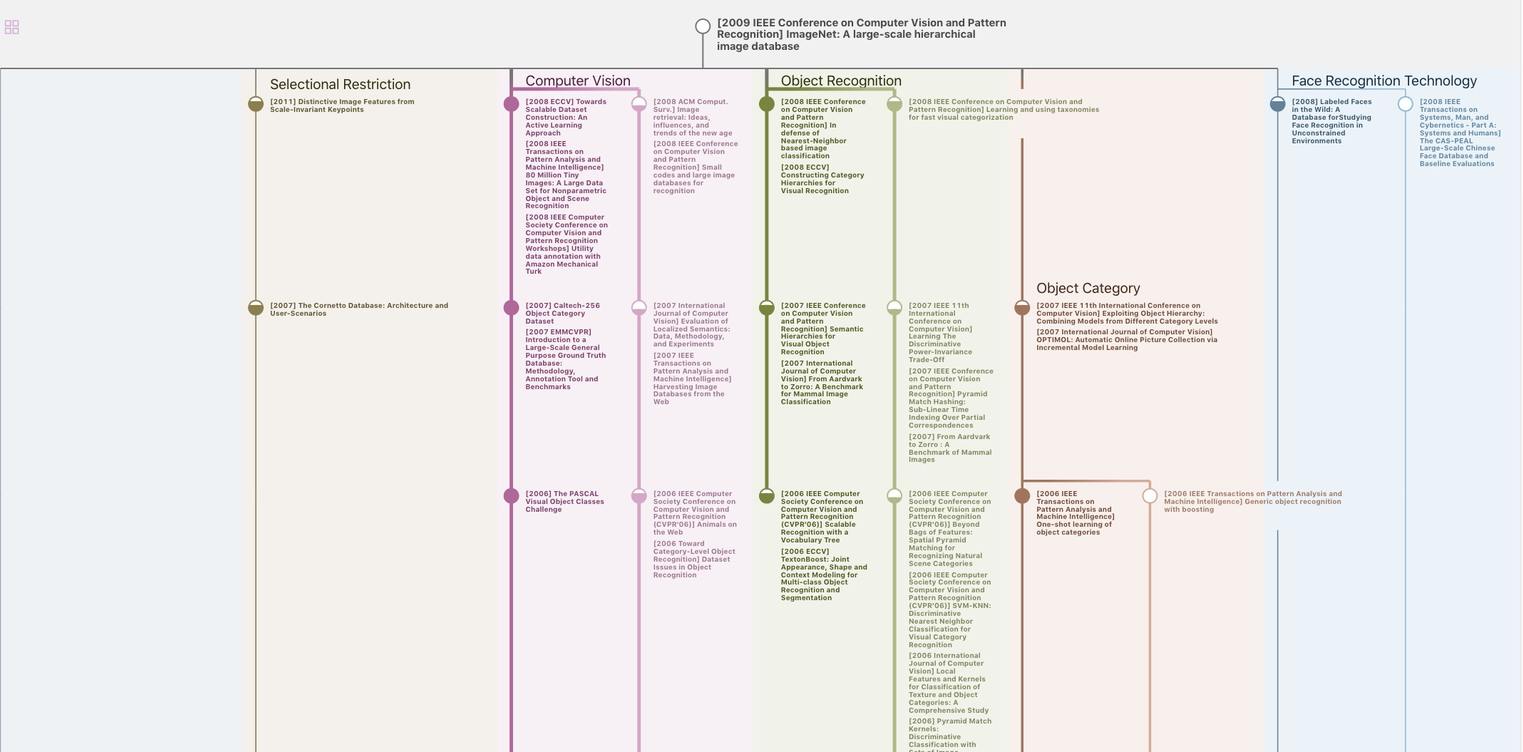
Generate MRT to find the research sequence of this paper
Chat Paper
Summary is being generated by the instructions you defined