Non-asymptotic estimation of risk measures using stochastic gradient Langevin dynamics
arXiv (Cornell University)(2023)
摘要
In this paper we will study the approximation of arbitrary law invariant risk measures. As a starting point, we approximate the average value at risk using stochastic gradient Langevin dynamics, which can be seen as a variant of the stochastic gradient descent algorithm. Further, the Kusuoka's spectral representation allows us to bootstrap the estimation of the average value at risk to extend the algorithm to general law invariant risk measures. We will present both theoretical, non-asymptotic convergence rates of the approximation algorithm and numerical simulations.
更多查看译文
关键词
stochastic gradient
AI 理解论文
溯源树
样例
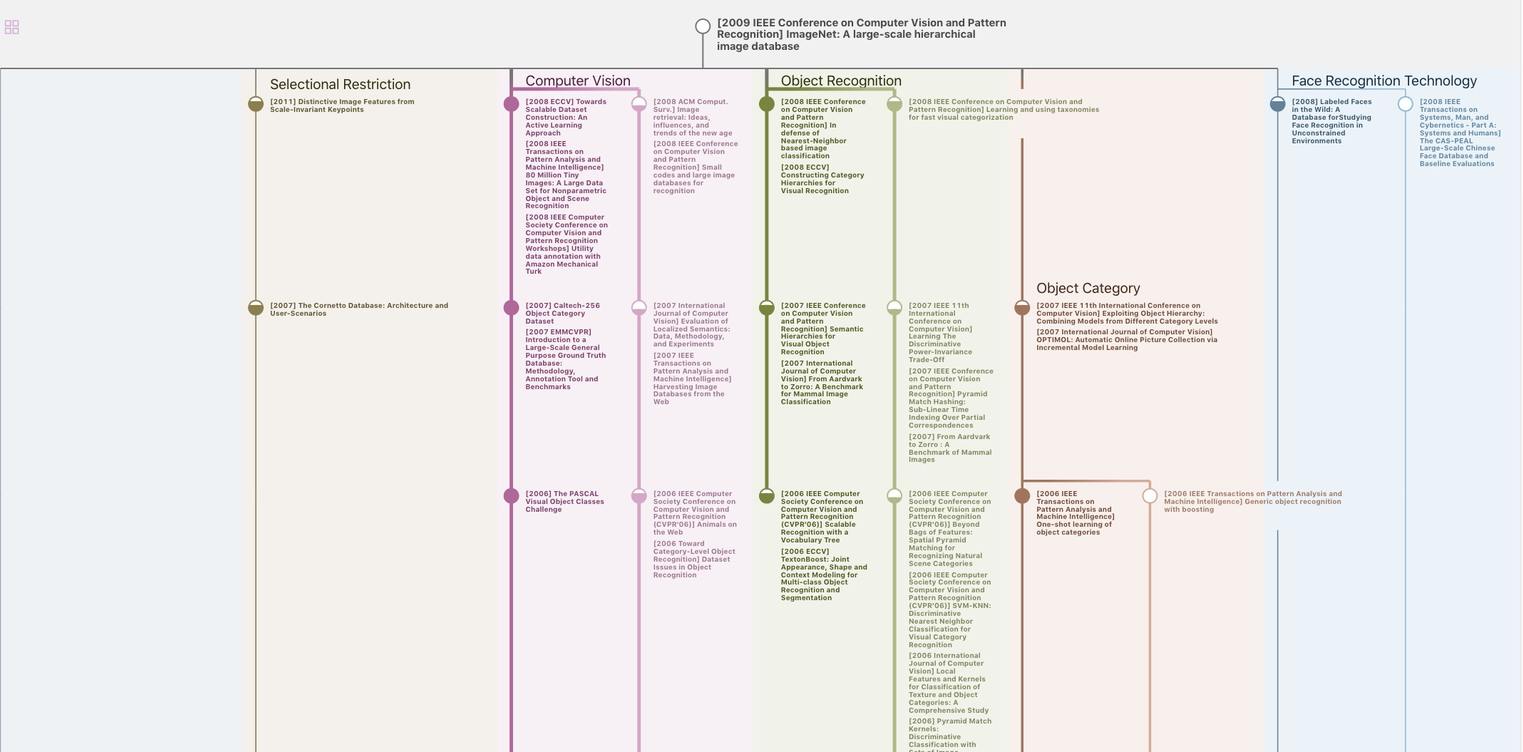
生成溯源树,研究论文发展脉络
Chat Paper
正在生成论文摘要