An unsupervised method for anomaly detection from crowd videos
Signal Processing and Communications Applications Conference(2013)
摘要
Anomaly detection from crowd videos is an issue that is becoming more important due to the difficulties in maintaining the public security in crowded places. Surveillance videos has a significant role for enabling the real time analysis of the captured events occurring in crowded places. This paper presents a method that detects anomalies in crowd in real-time using computer vision and machine learning techniques. The proposed method consists of extracting the crowd behavior properties (velocity, direction) by tracking scale invariant feature transform (SIFT) feature points and fitting the extracted behavior properties into a Gaussian Model. In this paper, only the global anomalies which occur on the overall video frame are handled. According to the test results, the method gives comparable results with the state-of-art methods and also can run in real-time. In addition, it is less complex than the compared state-of-art methods and works unsupervised.
更多查看译文
关键词
Gaussian processes,cognition,computer vision,feature extraction,object detection,object tracking,security of data,unsupervised learning,video surveillance,wavelet transforms,Gaussian model,SIFT feature point tracking,anomaly detection,computer vision,crowd behavior property extraction,crowd video,global anomaly handling,machine learning,public security,real time captured event analysis,scale invariant feature transform,unsupervised method,video frame,video surveillance,computer vision,crowd behavior analysis,video surveillance applications
AI 理解论文
溯源树
样例
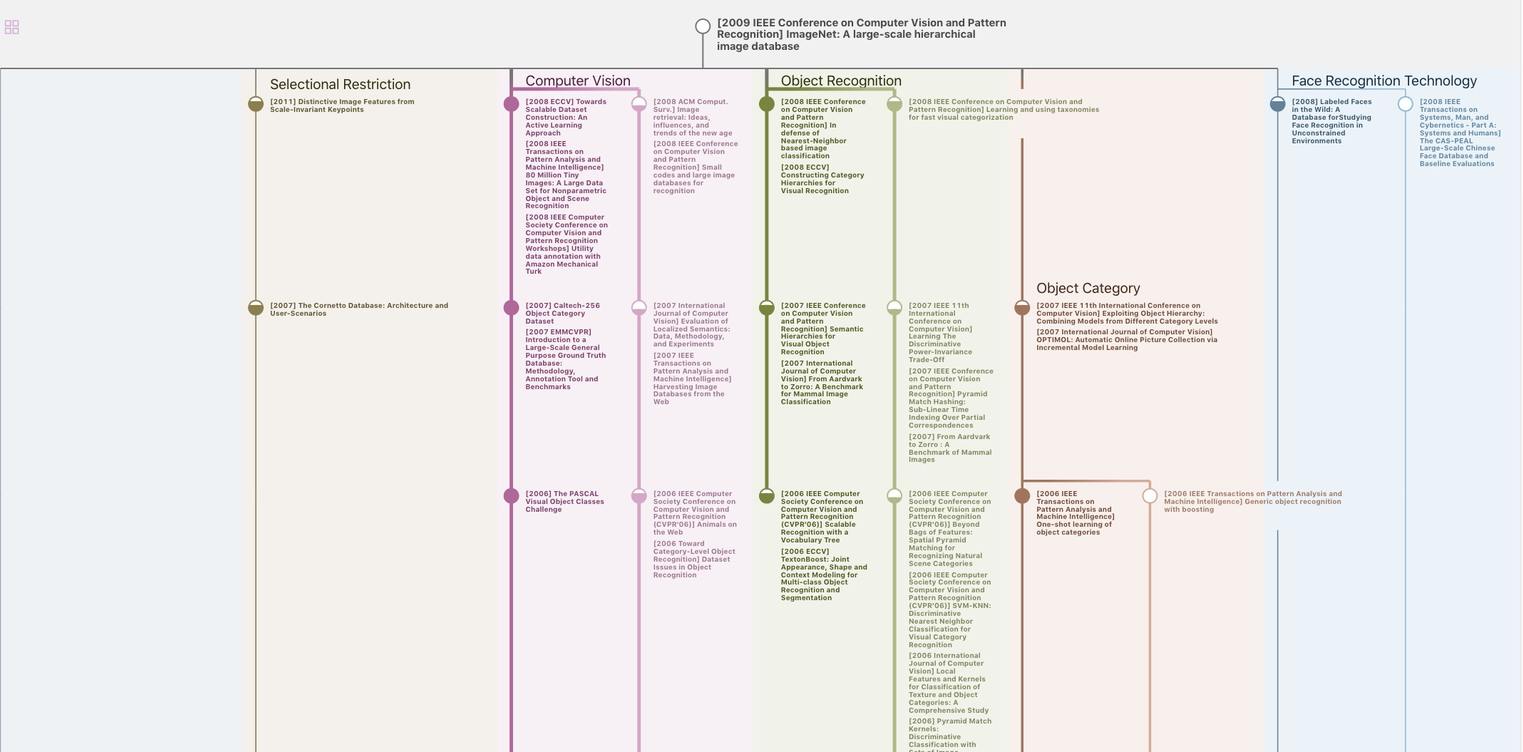
生成溯源树,研究论文发展脉络
Chat Paper
正在生成论文摘要