Continuous space language modeling techniques
Acoustics Speech and Signal Processing(2010)
摘要
This paper focuses on comparison of two continuous space language modeling techniques, namely Tied-Mixture Language modeling (TMLM) and Neural Network Based Language Modeling (NNLM). Additionally, we report on using alternative feature representations for words and histories used in TMLM. Besides bigram co-occurrence based features we consider using NNLM based input features for training TMLMs. We also describe how we improve certain steps in building TMLMs. We demonstrate that TMLMs provide significant improvements of over 16% relative and 10% relative in Character Error Rate (CER) for Mandarin speech recognition, over the trigram and NNLM models, respectively in a speech to speech translation task.
更多查看译文
关键词
natural language processing,neural nets,speech processing,speech recognition,Mandarin speech recognition,bigram co-occurrence,character error rate,continuous space language modeling techniques,feature representations,neural network based language modeling,speech-to-speech translation task,tied mixture language modeling,Continuous Space Modeling,Language Modeling,NNLM,Tied-Mixture Modeling
AI 理解论文
溯源树
样例
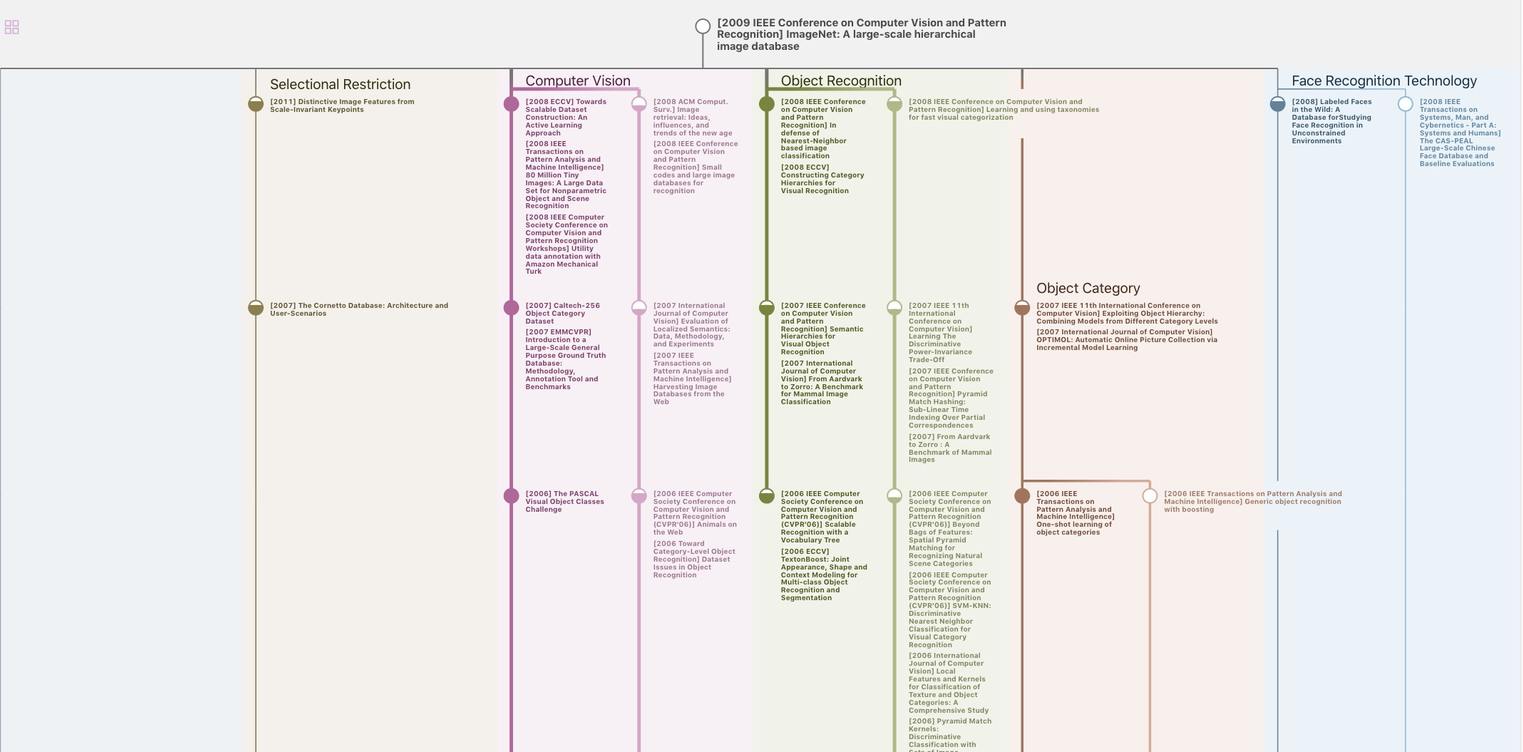
生成溯源树,研究论文发展脉络
Chat Paper
正在生成论文摘要