Autonomous trajectory learning using free configuration-eigenspaces
Signal Processing and Information Technology(2009)
摘要
This paper addresses the problem of autonomous trajectory learning in unknown environments through non-point based maps directly through the laser data. Our approach to solve the problem is based upon the hypothesis that in the low-dimensional manifolds of laser scanning data, there lies an eigenvector which corresponds to the free configuration space of the high order geometric representation of the environment. The vectorial combination of all these eigen-vectors at discrete time scan-frames manifest a trajectory, and once followed and mapped onto the two control signals of mobile robot will enable it to build an efficient and accurate online environment map. We demonstrate this process in robotic simulation and applied a probabilistic machine-learning aspect to find the free area likelihood which consequently builds the trajectory leading to improved map.
更多查看译文
关键词
eigenvalues and eigenfunctions,learning (artificial intelligence),mobile robots,path planning,autonomous trajectory learning,eigenvector,free configuration-eigenspaces,high order geometric representation,laser scanning data,mobile robot,online environment map,probabilistic machine-learning,robotic simulation,eigen-vector,free-configuration,mobile robotics,trajectory,machine-learning,eigen vector,lasers,configuration space,eigenvectors,discrete time,laser scanning,robot kinematics,machine learning,principal component analysis,learning artificial intelligence
AI 理解论文
溯源树
样例
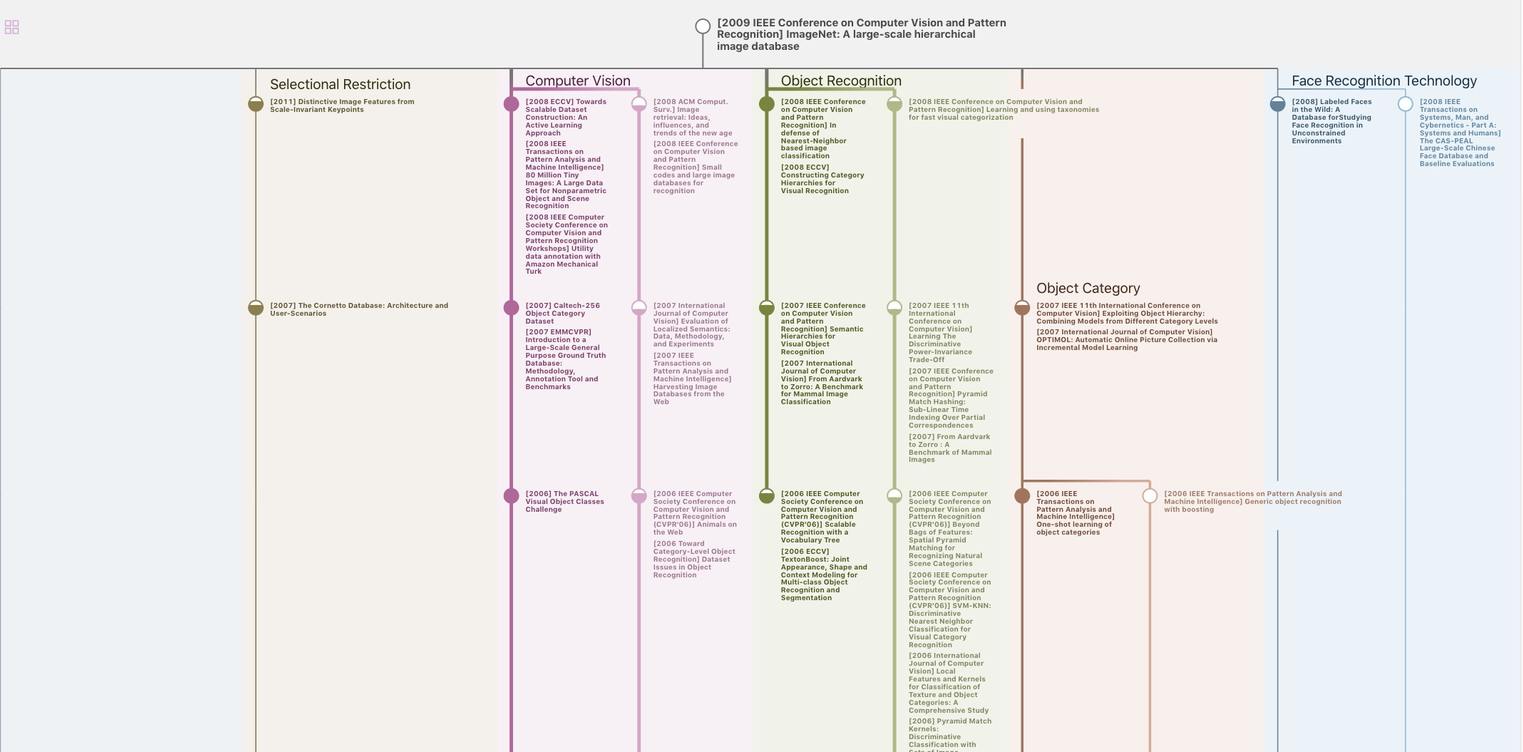
生成溯源树,研究论文发展脉络
Chat Paper
正在生成论文摘要