Spectral–Spatial Classification of Hyperspectral Image Based on Low-Rank Decomposition
Selected Topics in Applied Earth Observations and Remote Sensing, IEEE Journal of(2015)
摘要
Spectral–spatial classification methods have been proven to be effective in hyperspectral image (HSI) classification. However, most of the methods make use of the correlation in a small neighborhood. In this paper, a novel low-rank decomposition spectral–spatial method (LRDSS) is proposed. LRDSS incorporates the global and local correlation where the global correlation is introduced by discovering the low-dimensional structure in the high-dimensional data, and local correlation is modeled by Markov Random Field (MRF). Specifically, all pixels’ spectrums in a homogeneous area are assumed to have low-dimensional structure. Low rankness is a fine property to characterize the low-dimensional structure and robust principal component analysis (RPCA) is used to extract the low-rank data. Then, the spectral information is obtained by the probabilistic support vector machine (SVM) classifier applied on the low-rank data. Moreover, the MRF models local correlation by encouraging neighboring pixels taking the same label. The maximum a posterior classification is computed by min-cut-based optimization algorithm. The experimental results suggest that LRDSS outperforms the other spectral–spatial classification methods investigated in this paper in terms of classification accuracies.
更多查看译文
关键词
hyperspectral image (hsi) classification,markov random field (mrf),low-rank decomposition,support vector machine (svm),maximum likelihood estimation,markov processes,image classification,mrf,support vector machines,hyperspectral imaging
AI 理解论文
溯源树
样例
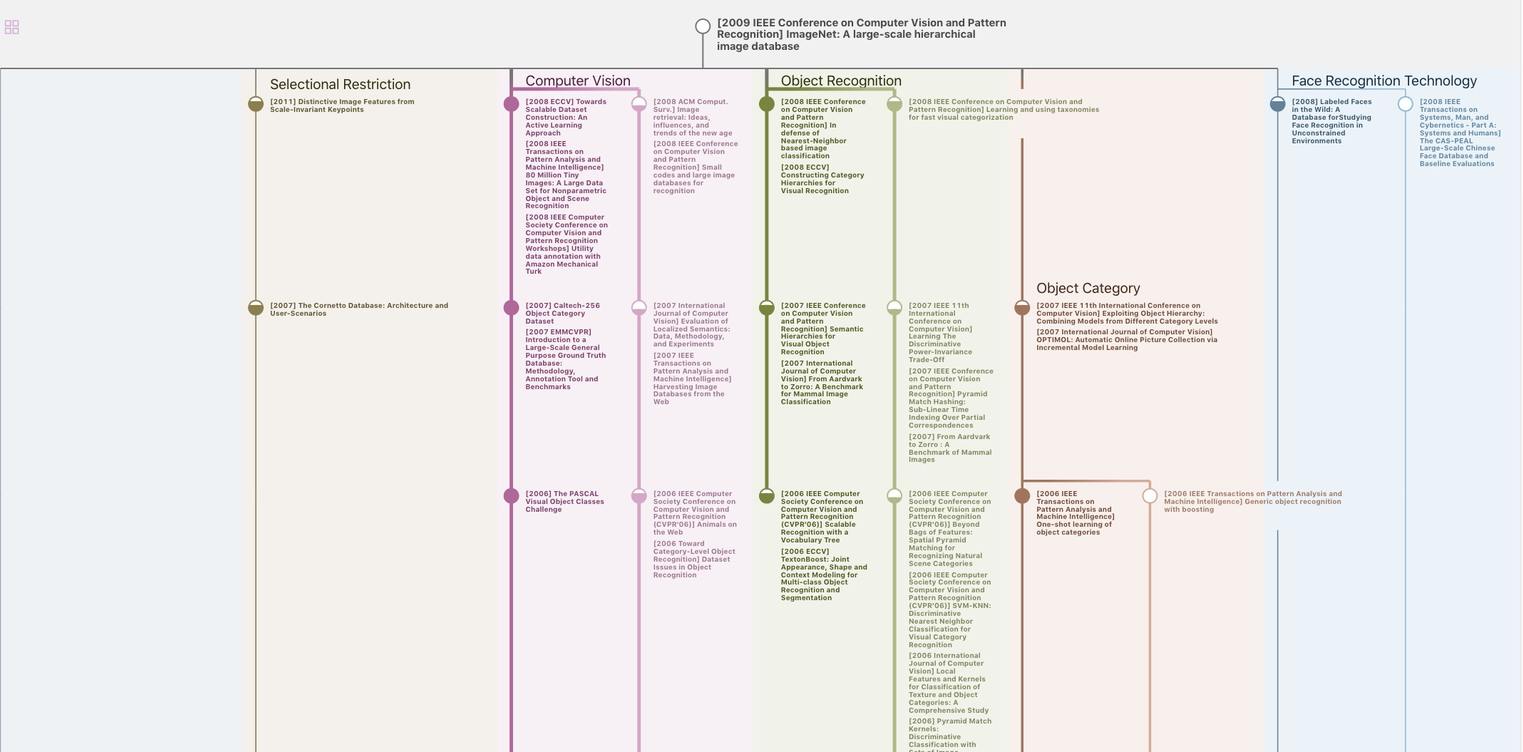
生成溯源树,研究论文发展脉络
Chat Paper
正在生成论文摘要