Voltage and Temperature-Aware SSTA Using Neural Network Delay Model
Semiconductor Manufacturing, IEEE Transactions(2011)
Abstract
With the emergence of voltage scaling as one of the most powerful power reduction techniques, it has been important to support voltage scalable statistical static timing analysis (SSTA) in deep submicrometer process nodes. In this paper, we propose a single delay model of logic gate using neural network which comprehensively captures process, voltage, and temperature variation along with input slew and output load. The number of simulation programs with integrated circuit emphasis (SPICE) required to create this model over a large voltage and temperature range is found to be modest and 4× less than that required for a conventional table-based approach with comparable accuracy. We show how the model can be used to derive sensitivities required for linear SSTA for an arbitrary voltage and temperature. Our experimentation on ISCAS 85 benchmarks across a voltage range of 0.9-1.1 V shows that the average error in mean delay is less than 1.08% and average error in standard deviation is less than 2.85%. The errors in predicting the 99% and 1% probability point are 1.31% and 1%, respectively, with respect to SPICE. The two potential applications of voltage-aware SSTA have been presented, i.e., one for improving the accuracy of timing analysis by considering instance-specific voltage drops in power grids and the other for determining optimum supply voltage for target yield for dynamic voltage scaling applications.
MoreTranslated text
Key words
spice,delays,integrated circuit design,integrated circuit modelling,logic design,logic gates,neural nets,statistical analysis,iscas 85 benchmark,deep submicrometer process,dynamic voltage scaling application,logic gate,neural network delay model,optimum supply voltage,power grids,simulation programs with integrated circuit emphasis,single delay model,temperature aware ssta,voltage 0.9 v to 1.1 v,voltage aware ssta,voltage scalable statistical static timing analysis,linear ssta,pvt-aware delay model,neural network,random local process variations,timing analysis in dvs,process variation,process control,neural networks,indexing terms,mathematical model,standard deviation,timing analysis
AI Read Science
Must-Reading Tree
Example
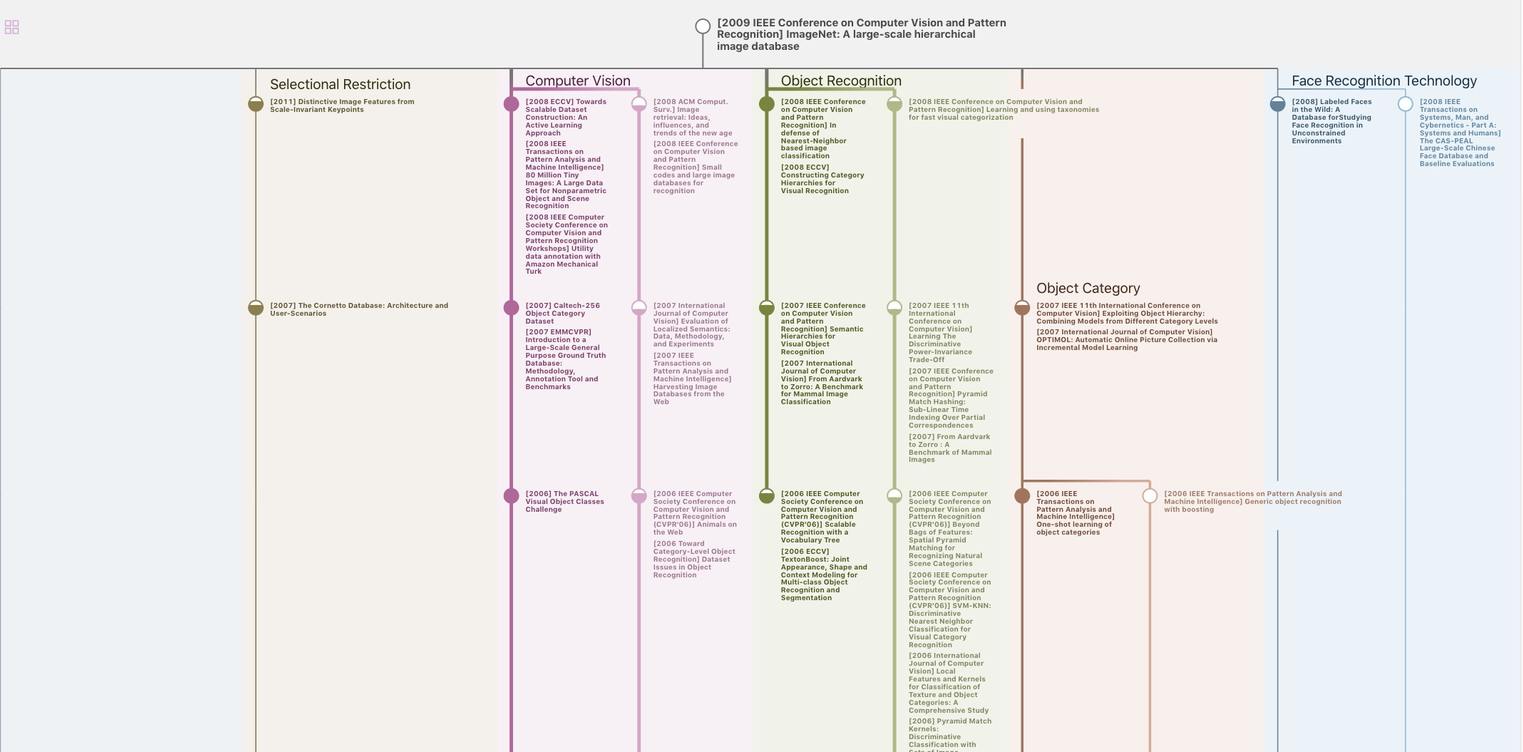
Generate MRT to find the research sequence of this paper
Chat Paper
Summary is being generated by the instructions you defined