Mutation Rate Matters Even When Optimizing Monotonic Functions
Evolutionary Computation(2013)
摘要
Extending previous analyses on function classes like linear functions, we analyze how the simple (1+1) evolutionary algorithm optimizes pseudo-Boolean functions that are strictly monotonic. These functions have the property that whenever only 0-bits are changed to 1, then the objective value strictly increases. Contrary to what one would expect, not all of these functions are easy to optimize. The choice of the constant c in the mutation probability p(n) = c/n can make a decisive difference. We show that if c < 1, then the (1+1) EA finds the optimum of every such function in Θ(n log n) iterations. For c = 1, we can still prove an upper bound of O(n(3/2)). However, for c ≥ 16, we present a strictly monotonic function such that the (1+1) EA with overwhelming probability needs 2(Ω(n)) iterations to find the optimum. This is the first time that we observe that a constant factor change of the mutation probability changes the runtime by more than a constant factor.
更多查看译文
关键词
Evolutionary algorithms,computational complexity,pseudo-Boolean optimization,runtime analysis
AI 理解论文
溯源树
样例
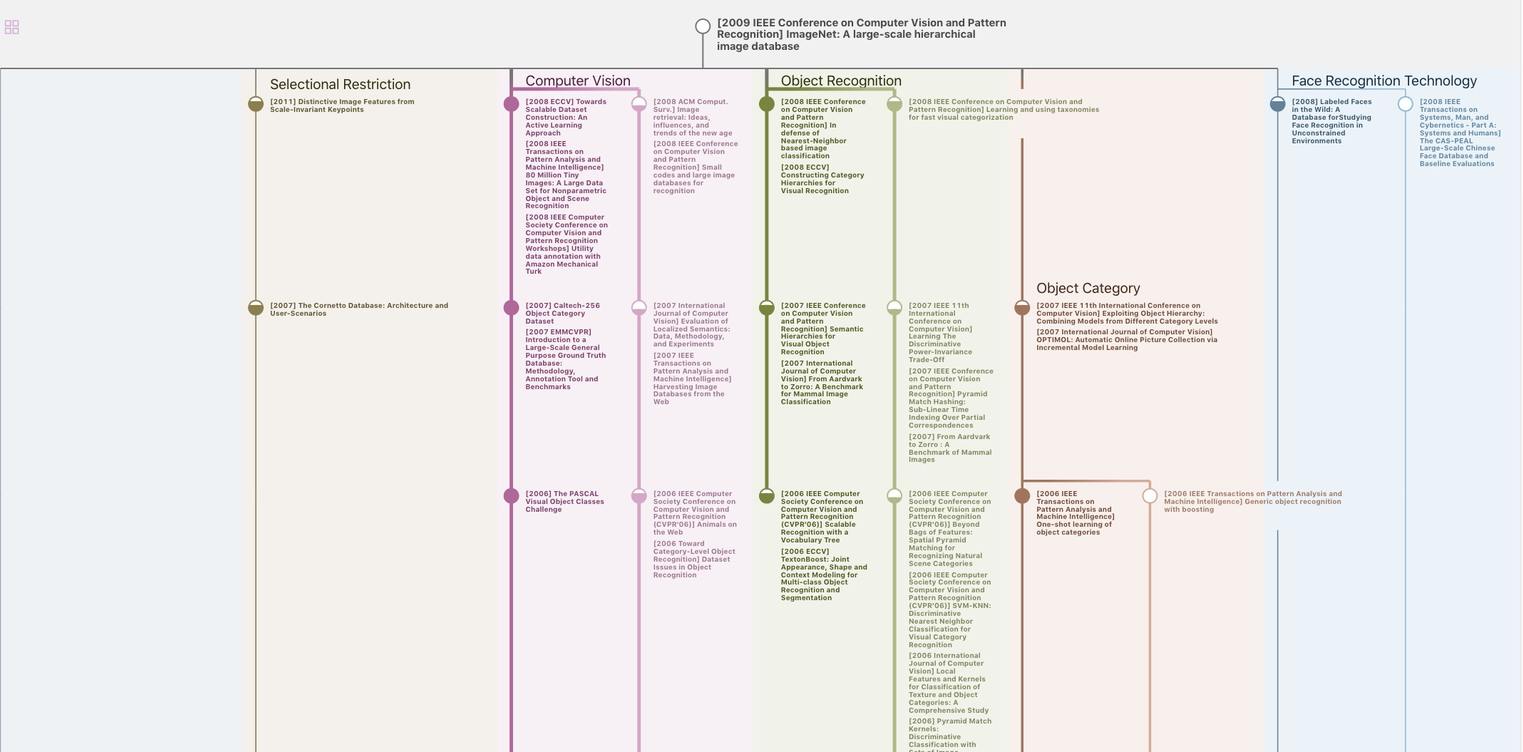
生成溯源树,研究论文发展脉络
Chat Paper
正在生成论文摘要