Beta-eigenspaces for autonomous mobile robotic trajectory outlier detection
Technologies for Practical Robot Applications(2011)
摘要
This paper addresses the problem of robotics trajectory building for efficient navigation and consequently exploration. A concept of beta-eigenvector has been introduced to find an outlier for the existing non-point based trajectory obtained from raw laser data. We have used our paradigm of “free configuration eigenspaces” method, with specific focus on outlier detection. The key idea proposed in this paper is to replace the Gaussian distribution utilized by the probabilistic principal component analysis (PPCA) with the beta-distribution. It is a well known issue that the conventional PCA is sensitive to anomalous observations because the calculation of sample mean and covariance matrix can be significantly influenced by a small number of outliers. Similarly, PPCA is not robust to outliers since the data are assumed to follow a multivariate Gaussian distribution that is easily affected by deviant observations. An outlier detection is done using Beta-Probabilistic Principal Component Analysis (BPPCA), further more an analysis is proposed to identify which variables contribute the most to the occurrence of outliers. The proposed technique is applied on real-time robotics sensor data as well as on the real-time robotics simulation environment.
更多查看译文
关键词
gaussian distribution,covariance matrices,eigenvalues and eigenfunctions,mobile robots,navigation,path planning,position control,principal component analysis,autonomous mobile robotic trajectory,beta eigenspaces,beta probabilistic principal component analysis,covariance matrix,free configuration eigenspaces,multivariate gaussian distribution,outlier detection,real time robotics sensor data,trajectory,lasers,mobile robot,mobile communication
AI 理解论文
溯源树
样例
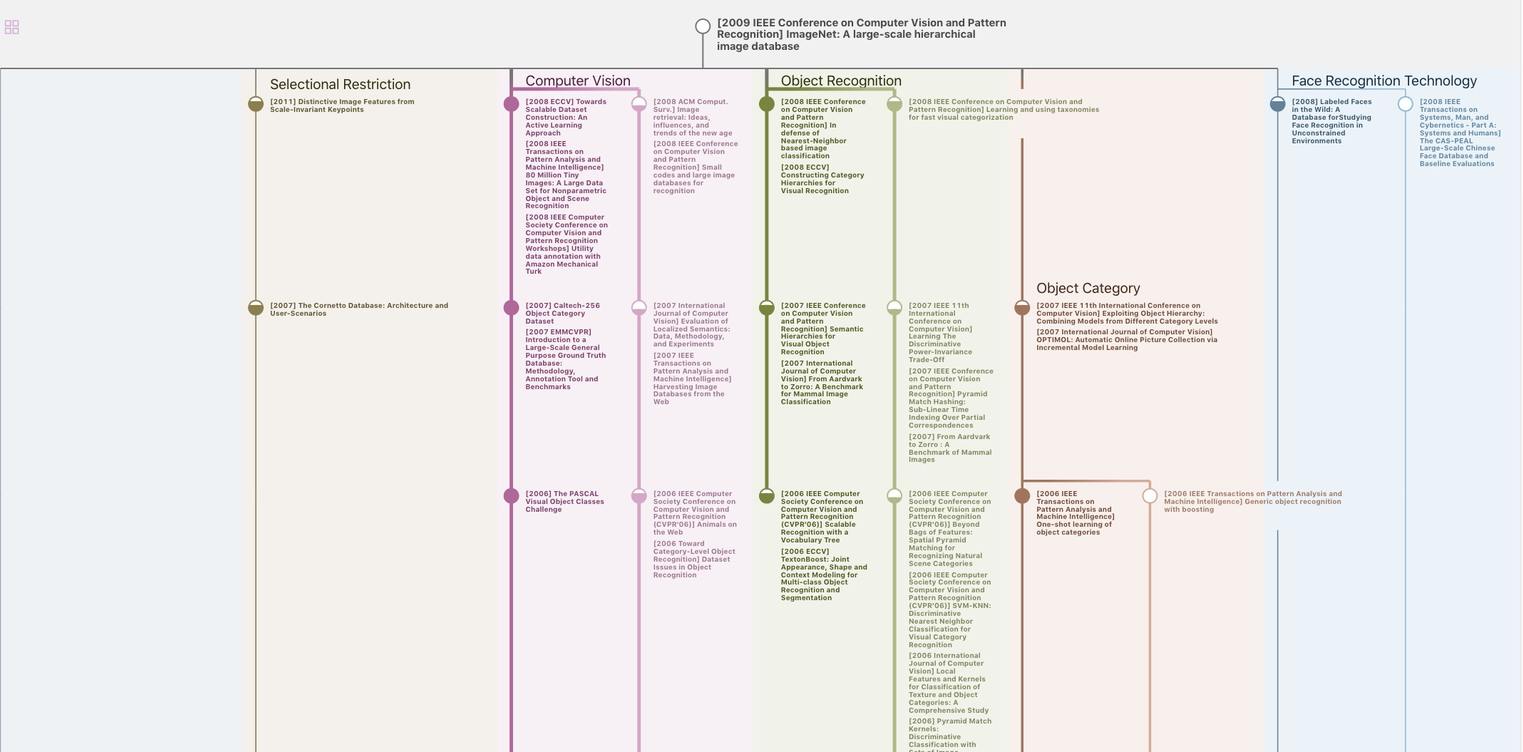
生成溯源树,研究论文发展脉络
Chat Paper
正在生成论文摘要