Cartification: A Neighborhood Preserving Transformation for Mining High Dimensional Data
Data Mining(2013)
摘要
The analysis of high dimensional data comes with many intrinsic challenges. In particular, cluster structures become increasingly hard to detect when the data includes dimensions irrelevant to the individual clusters. With increasing dimensionality, distances between pairs of objects become very similar, and hence, meaningless for knowledge discovery. In this paper we propose Cartification, a new transformation to circumvent this problem. We transform each object into an item set, which represents the neighborhood of the object. We do this for multiple views on the data, resulting in multiple neighborhoods per object. This transformation enables us to preserve the essential pair wise-similarities of objects over multiple views, and hence, to improve knowledge discovery in high dimensional data. Our experiments show that frequent item set mining on the certified data outperforms competing clustering approaches on the original data space, including traditional clustering, random projections, principle component analysis, subspace clustering, and clustering ensemble.
更多查看译文
关键词
data mining,pattern clustering,cartification,clustering ensemble,high dimensional data mining,knowledge discovery,neighborhood preserving transformation,principle component analysis,random projections,subspace clustering,clustering,frequent itemset mining,high dimensional data,subspace projections,transformation
AI 理解论文
溯源树
样例
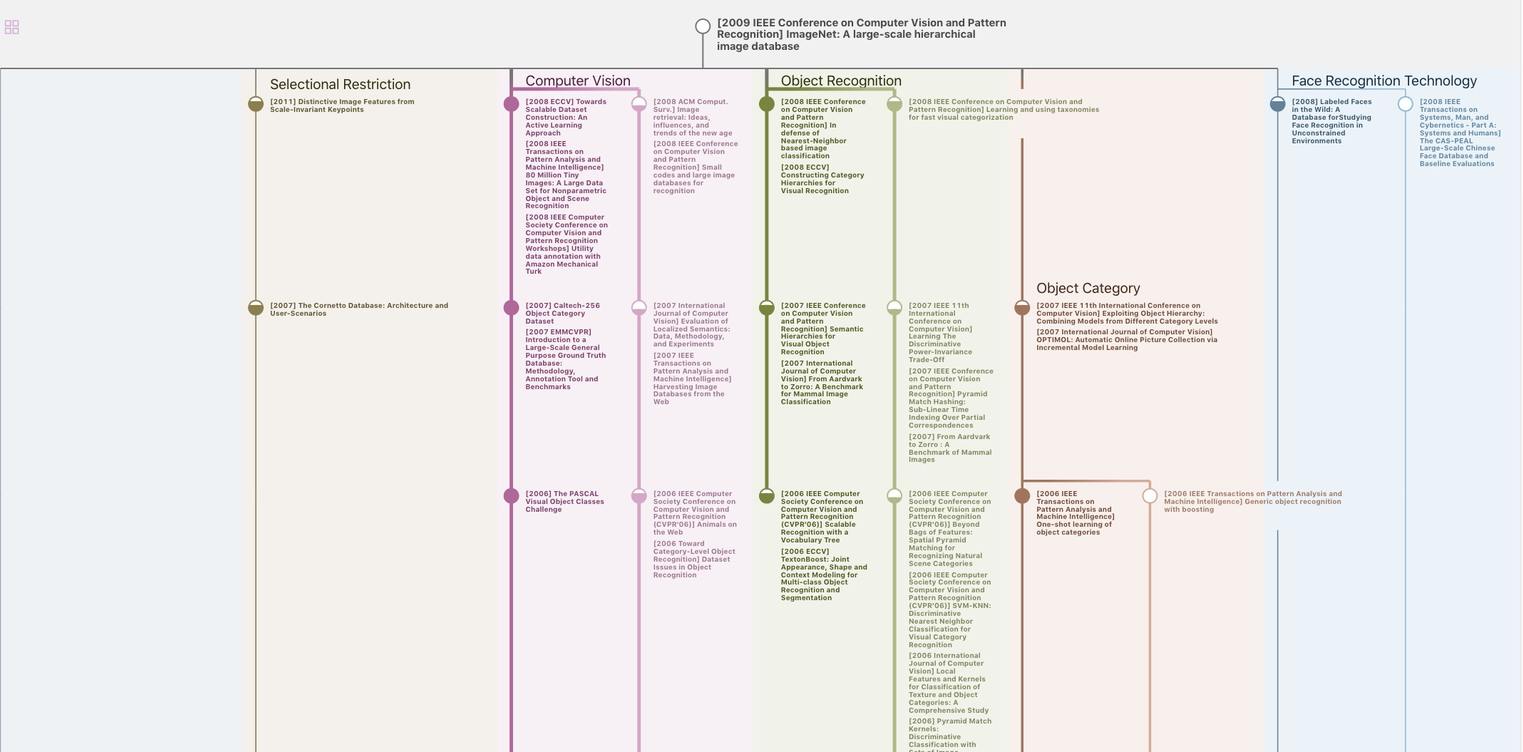
生成溯源树,研究论文发展脉络
Chat Paper
正在生成论文摘要