Relevant subspace selection in Kernel feature space for speech recognition
India Conference(2010)
摘要
This paper describes an approach to select the most relevant subspace in Kernel PCA feature space applied on MFCC coefficients for speech recognition. It has been seen that the relevant information about a supervised classification problem is contained in a finite number of leading Kernel PCA components if the Kernel matches the underlying classification problem. In this paper our contribution is to foster an understanding about the appropriate Kernel selection for different phonemes in a speech database and then create an insight about the most relevant dimensions for those phonemes in that Kernel space. Using this approach we have obtained better results for speech recognition as compared to standard technique.
更多查看译文
关键词
feature extraction,principal component analysis,speech recognition,kernel pca feature space,kernel feature space,mfcc coefficients,speech database,supervised classification,kpca,relevant dimension estimation,relevant subspace selection,mel frequency cepstral coefficient,kernel pca,kernel,feature space,speech
AI 理解论文
溯源树
样例
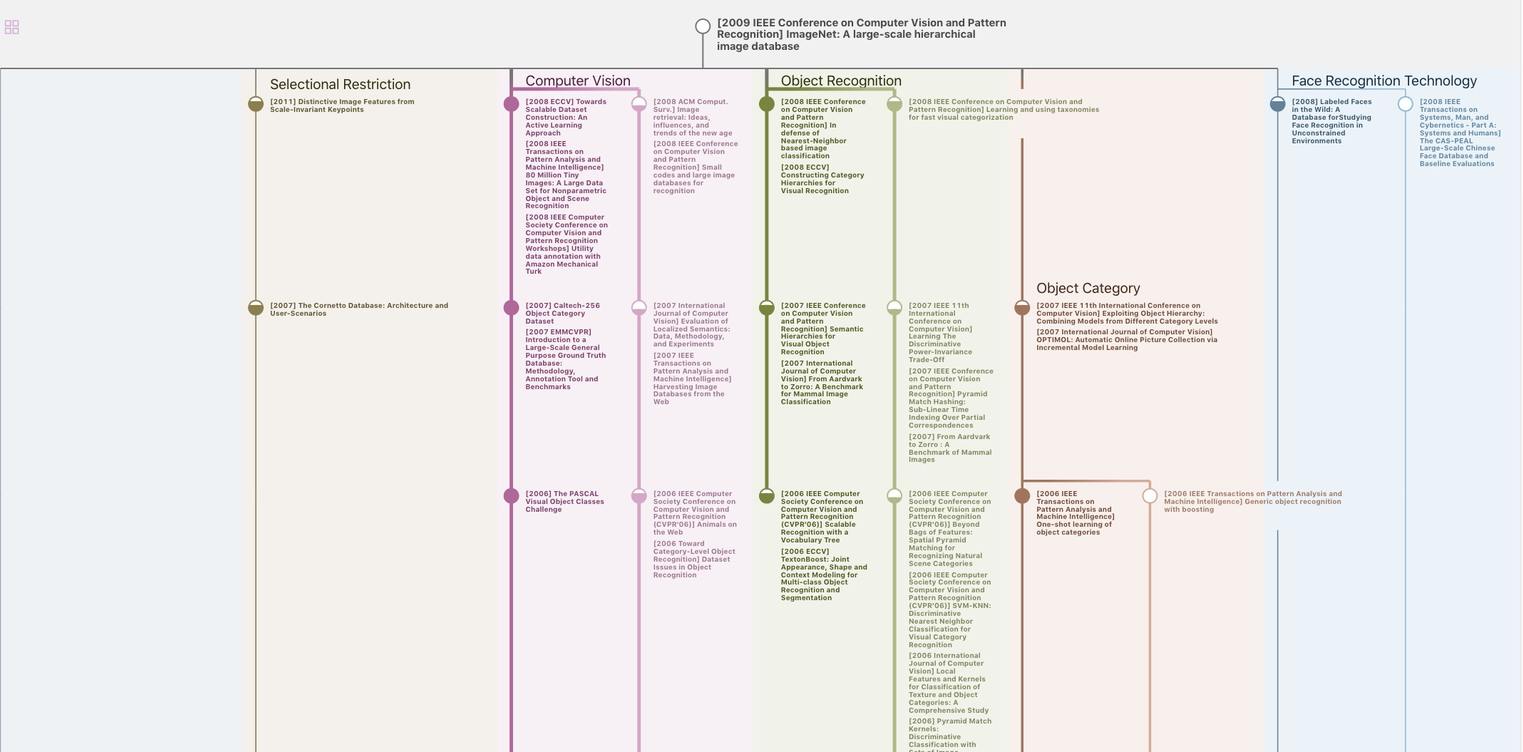
生成溯源树,研究论文发展脉络
Chat Paper
正在生成论文摘要