RANSAC for motion-distorted 3D visual sensors
Intelligent Robots and Systems(2013)
摘要
Visual odometry (VO) is a highly efficient and powerful 6D motion estimation technique; state-of-the-art bundle adjustment algorithms now optimize over several frames of temporally tracked, appearance-based features in real time. It is well known that the temporal feature correspondence process is highly prone to mismatches. The standard technique used for outlier rejection in this process is random sample consensus (RANSAC), which is an iterative and non-deterministic process used to find the parameters of a mathematical model that best describe a likely set of inliers. The traditional model used for RANSAC in the visual odometry pipeline is a rigid transformation between two camera poses; this model has long assumed the use of an imaging sensor with a global shutter. In order to use imaging sensors that do not operate with a global shutter, it is proposed that the RANSAC algorithm be modified to use a constant-camera-velocity model. Specifically, this paper investigates the use of a two-axis scanning lidar in the visual-odometry pipeline. Images are formed using lidar intensity data, and due to the scanning-while-moving nature of the lidar, the behaviour of the sensor resembles that of a slow rolling-shutter camera. We formulate a Motion-Compensated RANSAC algorithm that uses a constant-velocity model and the individual timestamp of each extracted feature. The algorithm is validated using 6880 lidar frames with a resolution of 480 × 360, captured at 2 Hz, over a 1.1 km traversal. Our results show that the new algorithm results in far more inlying feature tracks for rolling-shutter-type images and ultimately higher-accuracy VO results.
更多查看译文
关键词
distance measurement,motion compensation,motion estimation,real-time systems,6D motion estimation technique,VO,bundle adjustment algorithms,imaging sensor,motion-compensated RANSAC algorithm,motion-distorted 3D visual sensors,random sample consensus,real time systems,visual odometry pipeline
AI 理解论文
溯源树
样例
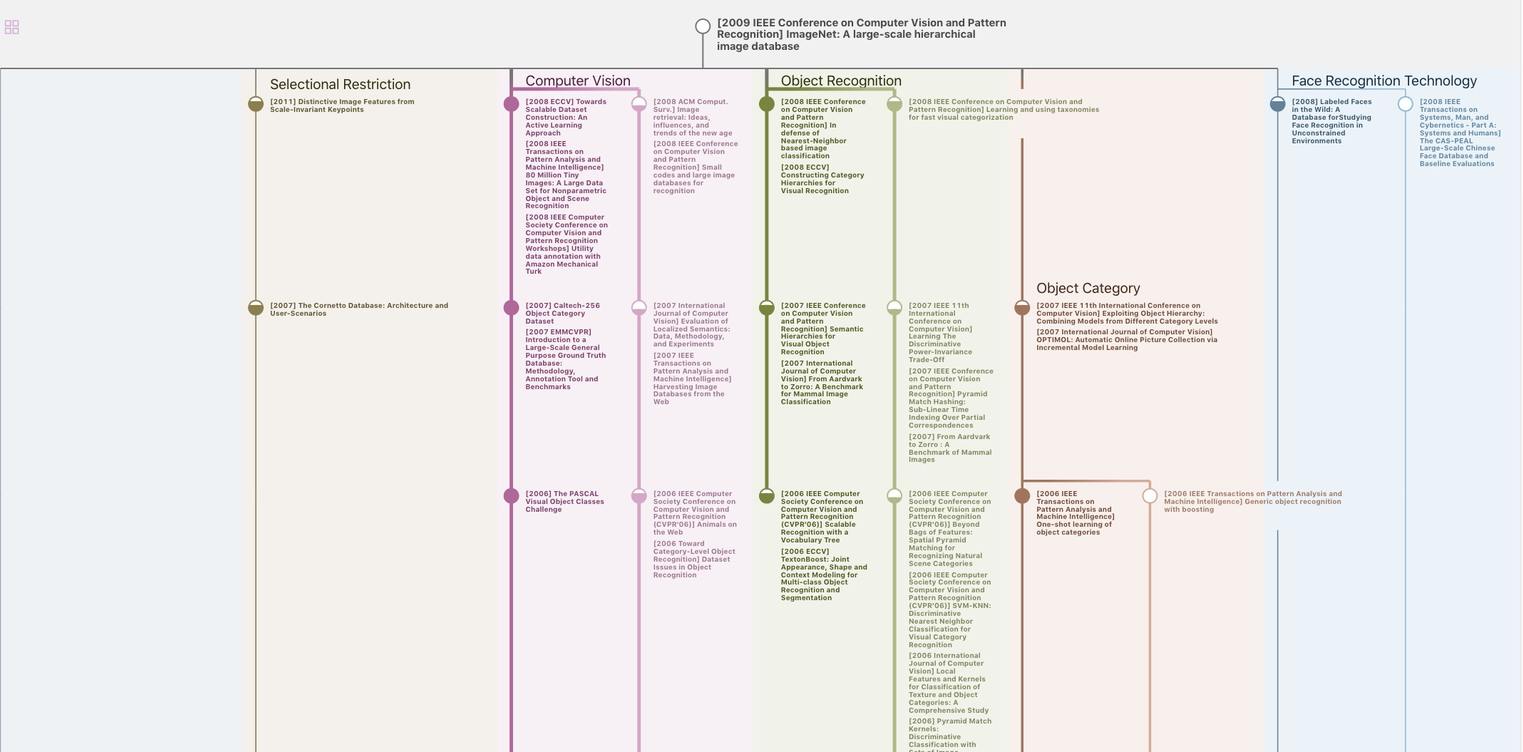
生成溯源树,研究论文发展脉络
Chat Paper
正在生成论文摘要