Traversability estimation for a planetary rover via experimental kernel learning in a Gaussian process framework
Robotics and Automation(2013)
摘要
A critical requirement for safe autonomous navigation of a planetary rover is the ability to accurately estimate the traversability of the terrain. This work considers the problem of predicting the attitude and configuration angles of the platform from terrain representations that are often incomplete due to occlusions and sensor limitations. Using Gaussian Processes (GP) and exteroceptive data as training input, we can provide a continuous and complete representation of terrain traversability, with uncertainty in the output estimates. In this paper, we propose a novel method that focuses on exploiting the explicit correlation in vehicle attitude and configuration during operation by learning a kernel function from vehicle experience to perform GP regression. We provide an extensive experimental validation of the proposed method on a planetary rover. We show significant improvement in the accuracy of our estimation compared with results obtained using standard kernels (Squared Exponential and Neural Network), and compared to traversability estimation made over terrain models built using state-of-the-art GP techniques.
更多查看译文
关键词
gaussian processes,aerospace robotics,learning (artificial intelligence),neurocontrollers,path planning,planetary rovers,regression analysis,gp regression,gaussian process framework,attitude angle,configuration angles,experimental kernel learning,explicit correlation,exteroceptive data,kernel function learning,neural network kernel,planetary rover,planetary rover autonomous navigation,squared exponential kernel,terrain traversability estimation,terrain traversability representation,vehicle attitude,vehicle configuration,learning artificial intelligence
AI 理解论文
溯源树
样例
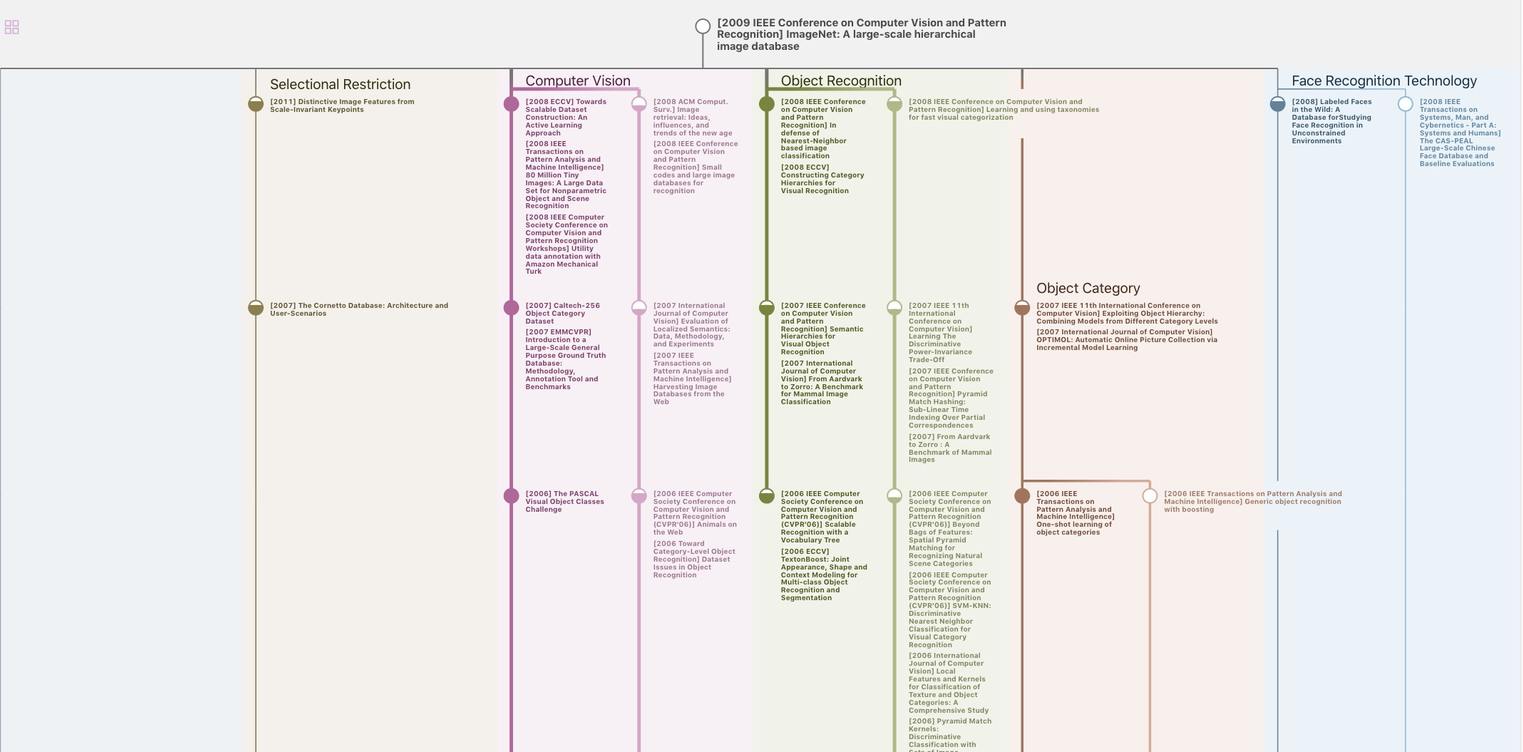
生成溯源树,研究论文发展脉络
Chat Paper
正在生成论文摘要