Continuous wavelet transform based partial least squares regression for quantitative analysis of Raman spectrum.
IEEE transactions on nanobioscience(2013)
摘要
Quantitative analysis of Raman spectra using surface-enhanced Raman scattering (SERS) nanoparticles has shown the potential and promising trend of development in in vivo molecular imaging. Partial least square regression (PLSR) methods have been reported as state-of-the-art methods. However, the approaches fully rely on the intensities of Raman spectra and can not avoid the influences of the unstable background. In this paper we design a new continuous wavelet transform based PLSR (CWT-PLSR) algorithm that uses mixing concentrations and the average CWT coefficients of Raman spectra to carry out PLSR. We elaborate and prove how the average CWT coefficients with a Mexican hat mother wavelet are robust representations of Raman peaks, and the method can reduce the influences of unstable baseline and random noises during the prediction process. The algorithm was tested using three Raman spectra data sets with three cross-validation methods in comparison with current leading methods, and the results show its robustness and effectiveness.
更多查看译文
关键词
random noises,continuous wavelet transform based partial least squares regression,plsr,nanoparticles,raman peaks,surface-enhanced raman scattering,raman spectra,wavelet transforms,regression analysis,surface enhanced raman scattering,spectrochemical analysis,molecular biophysics,least squares approximations,random noise,quantitative analysis,in vivo molecular imaging,raman spectrum,cross-validation methods,mixing,mixing concentrations,state-of-the-art methods,mexican hat mother wavelet,cwt
AI 理解论文
溯源树
样例
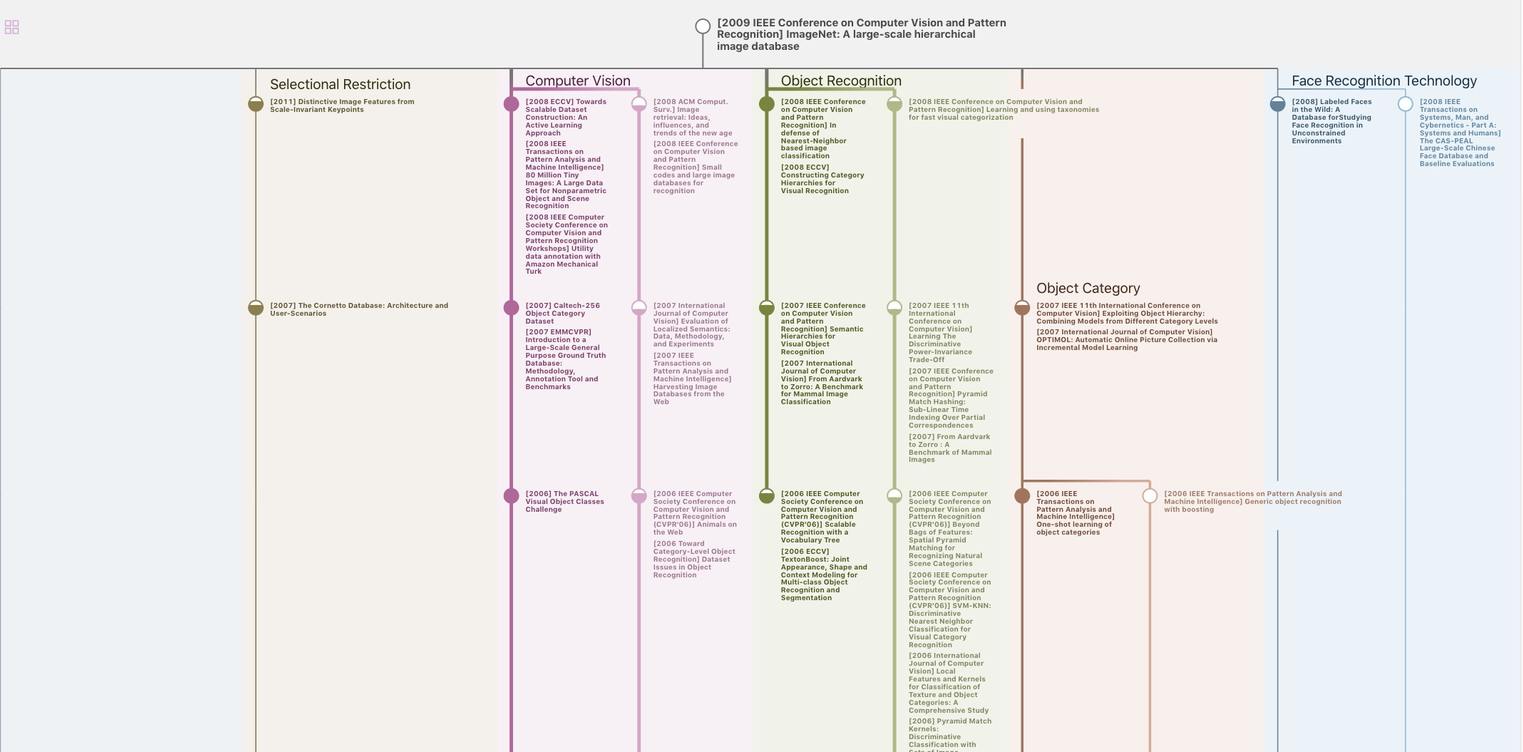
生成溯源树,研究论文发展脉络
Chat Paper
正在生成论文摘要