Image resolution improvement: A signal processing approach
Industrial and Information Systems(2010)
Abstract
High-resolution (HR) images are in demand because they not only give the viewer a pleasing view of the scene but also offer additional details that are important for analysis. Signal processing approach can be used to improve the resolution from several sub pixel shifted low resolution images. The image to be super-resolved is estimated from a set of low resolution (LR) observations, using Least squares (LS) technique. Ill-posedness is addressed by Regularized Least Squares (RLS) technique which utilizes smoothness prior. In this paper, we first demonstrate the improved noise robustness of RLS approach over the LS. Also, to avoid over smoothness, we model the image to be super-resolved as Markov random field and estimate it from the observations by a Graduated non convexity optimization procedure. A discontinuity adaptive regularizer is used to preserve the edges in the reconstructed image. Several experimental results are provided to demonstrate the robustness of the proposed algorithm.
MoreTranslated text
Key words
Markov processes,image resolution,least squares approximations,signal processing,Markov random field,discontinuity adaptive regularizer,graduated nonconvexity optimization,high-resolution images,image resolution improvement,low resolution images,regularized least squares technique,signal processing,Markov random field (MRF),Super-resolution,graduated nonconvexity (GNC),ill-posed problem,imaging process,prior constraints,regularization,
AI Read Science
Must-Reading Tree
Example
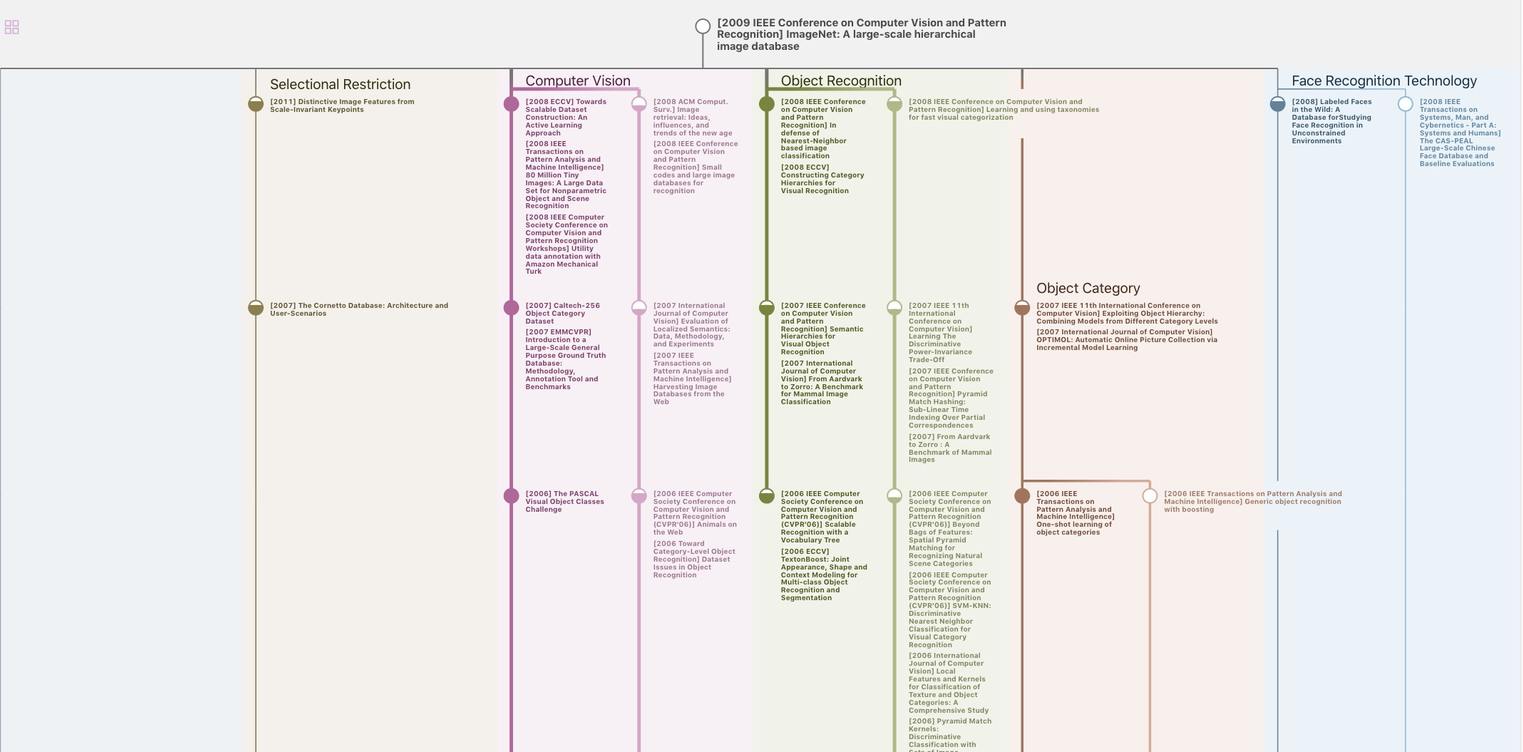
Generate MRT to find the research sequence of this paper
Chat Paper
Summary is being generated by the instructions you defined