Negotiation and Learning in distributed MPC of Large Scale Systems
advances in computing and communications(2010)
摘要
A key issue in distributed MPC control of Large Scale Systems (LSS) is how shared variables among the different MPC controller in charge of controlling each system partition (subsystems) are handled. When these connections represent control variables, the distributed control has to be consistent for both subsystems and the optimal value of these variables will have to accomplish a common goal. In order to achieve this, the present work combines ideas from Distributed Artificial Intelligence (DAI), Reinforcement Learning (RL) and Model Predictive Control (MPC) in order to provide an approach based on negotiation, cooperation and learning techniques. Results of the application of this approach to a small drinking water network show that the resulting trajectories of the levels in tanks (control variables) can be acceptable compared to the centralized solution. The application to a real network (the Barcelona case) is currently under development.
更多查看译文
关键词
artificial intelligence,centralised control,distributed control,large-scale systems,learning (artificial intelligence),predictive control,barcelona case,centralized solution,distributed mpc control,distributed artificial intelligence,large scale systems,model predictive control,reinforcement learning,system partition,water network,cooperative systems,multi agent systems,negotiation
AI 理解论文
溯源树
样例
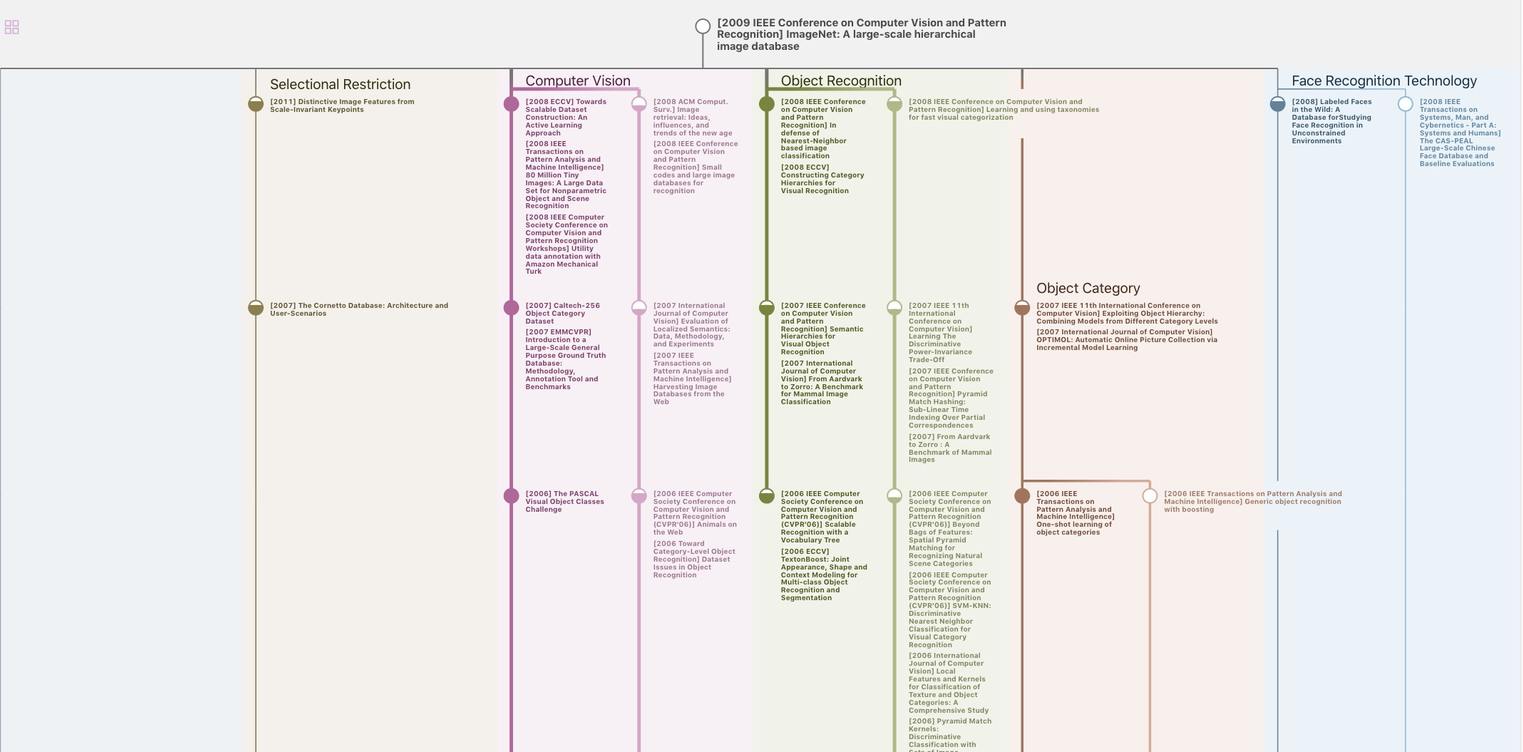
生成溯源树,研究论文发展脉络
Chat Paper
正在生成论文摘要