A novel hardware efficient Digital Neural Network architecture implemented in 130nm technology
ICCAE), 2010 The 2nd International Conference(2010)
Abstract
Digital Neural Network implementations based on the perceptron model require the use of multi-bit representation of signals and weights. This results in the usage of multi-bit multipliers in each neuron, leading to prohibitively large chip areas. Another problem with hardware implementations of neural networks is the low utilization of chip area due to complex interconnection requirements between successive neuron layers. In this paper we propose an architecture having a single layer of digital neurons that is reused multiple number of times with different weight vectors in order to achieve significant reduction in the required silicon area. The proposed architecture results in a significantly reduced power consumption (55% reduction for an 8 layer, 4 neuron per layer network). The paper also includes the results obtained on implementing the proposed architecture in 130 nm technology using MAGMA blast-fusion design tool.
MoreTranslated text
Key words
multilayer perceptrons,neural chips,neural net architecture,MAGMA blast-fusion design tool,digital neurons,hardware efficient digital neural network architecture,multibit multipliers,multibit signal representation,power consumption,size 130 nm,weight vectors,digital,hardware,neural networks,
AI Read Science
Must-Reading Tree
Example
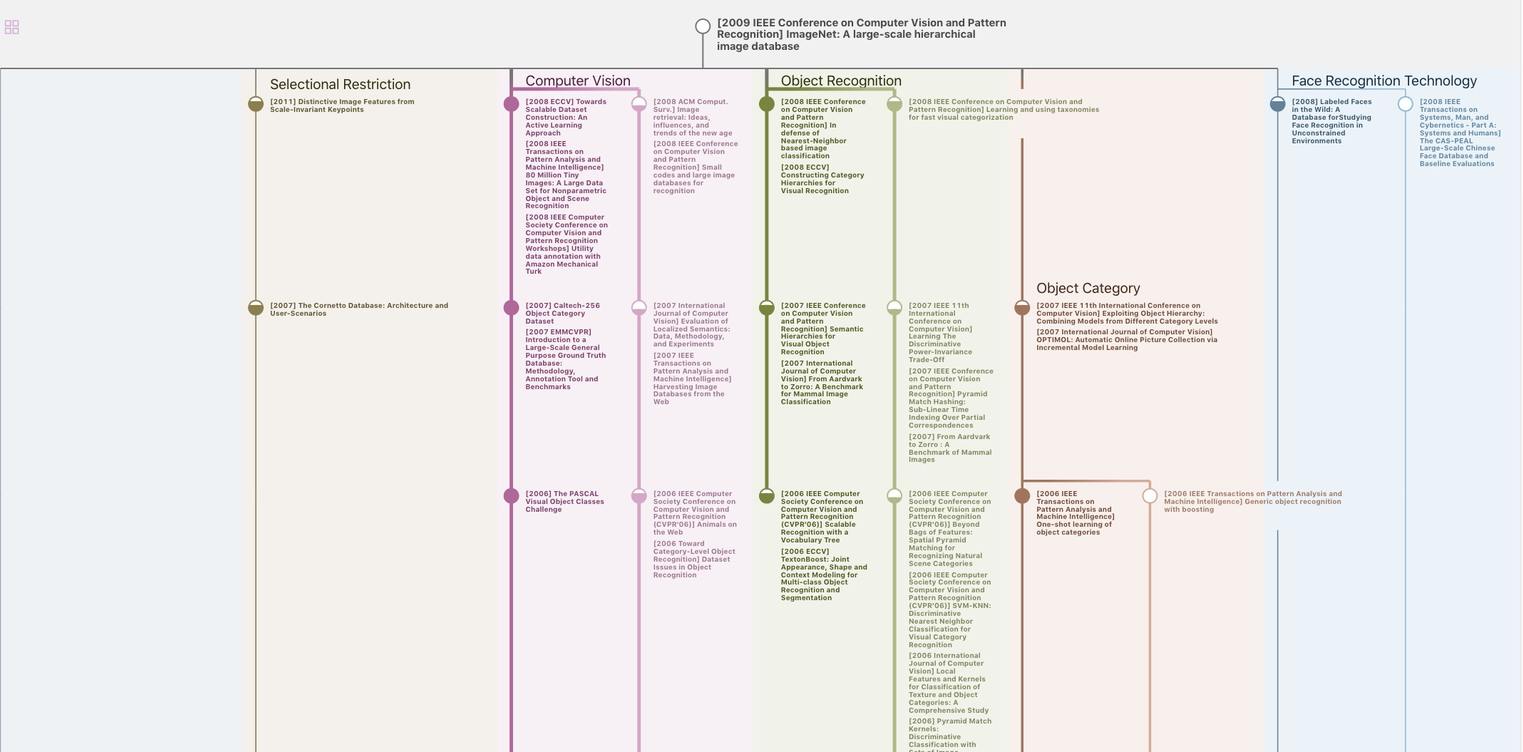
Generate MRT to find the research sequence of this paper
Chat Paper
Summary is being generated by the instructions you defined