Spectral clustering of shape and probability prior models for automatic prostate segmentation.
EMBC(2012)
Abstract
Imaging artifacts in Transrectal Ultrasound (TRUS) images and inter-patient variations in prostate shape and size challenge computer-aided automatic or semi-automatic segmentation of the prostate. In this paper, we propose to use multiple mean parametric models derived from principal component analysis (PCA) of shape and posterior probability information to segment the prostate. In contrast to traditional statistical models of shape and intensity priors, we use posterior probability of the prostate region determined from random forest classification to build, initialize and propagate our model. Multiple mean models derived from spectral clustering of combined shape and appearance parameters ensure improvement in segmentation accuracies. The proposed method achieves mean Dice similarity coefficient (DSC) value of 0.96±0.01, with a mean segmentation time of 0.67±0.02 seconds when validated with 46 images from 23 datasets in a leave-one-patient-out validation framework.
MoreTranslated text
Key words
trus images,imaging artifacts,random forest,prior probability model,statistical shape and posterior probability models,computer aided semiautomatic segmentation,computer aided automatic segmentation,shape information,shape prior model,prostate segmentation,image segmentation,biomedical ultrasonics,pca,automatic prostate segmentation,transrectal ultrasound images,spectral clustering,shape parameters,posterior probability information,prostate size interpatient variations,intensity prior,principal component analysis,prostate shape interpatient variations,biological organs,multiple mean parametric models,appearance parameters,medical image processing
AI Read Science
Must-Reading Tree
Example
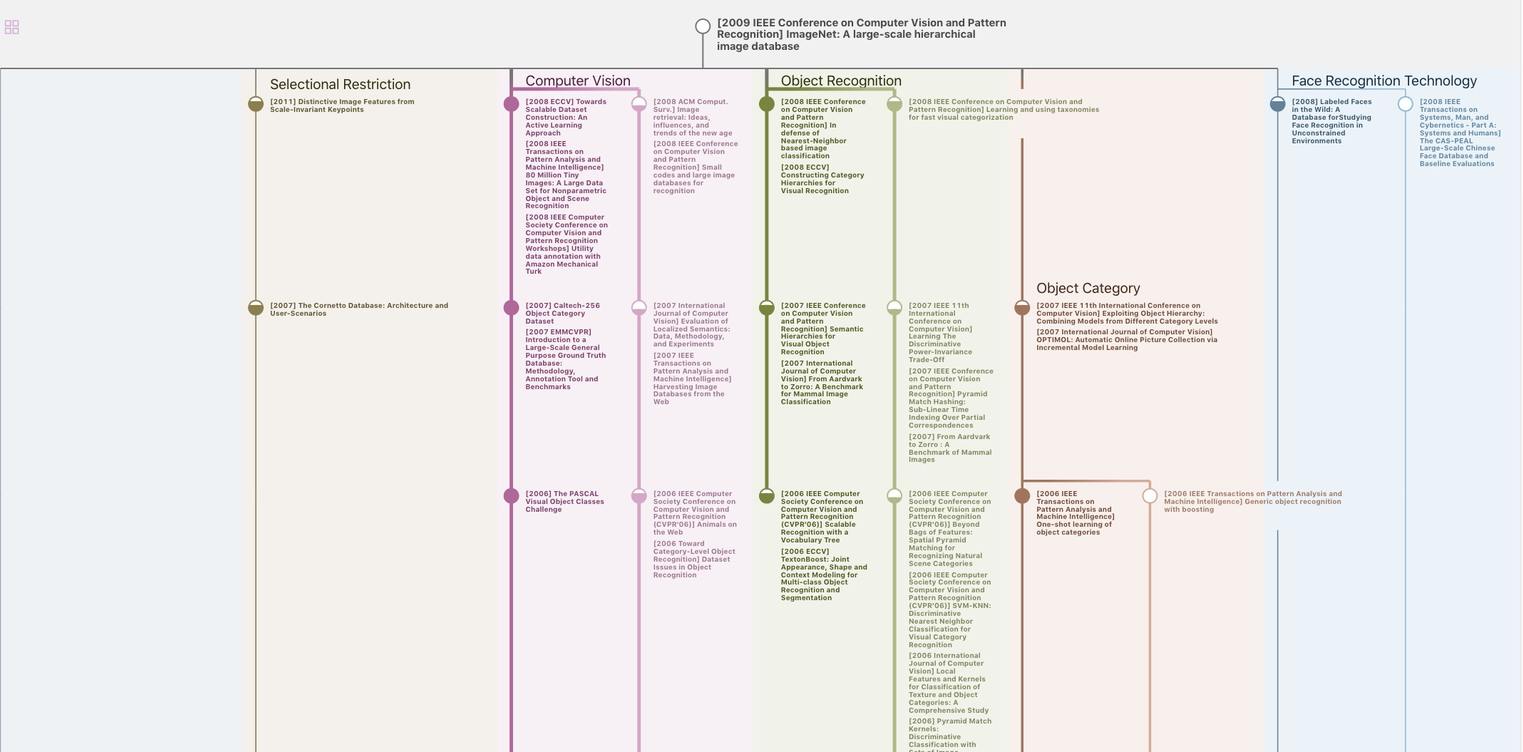
Generate MRT to find the research sequence of this paper
Chat Paper
Summary is being generated by the instructions you defined