An algorithm for fast edit distance computation on GPUs
Innovative Parallel Computing(2012)
摘要
The problem of finding the edit distance between two sequences (and its closely related problem of longest common subsequence) are important problems with applications in many domains like virus scanners, security kernels, natural language translation and genome sequence alignment. The traditional dynamic-programming based algorithm is hard to parallelize on SIMD processors as the algorithm is memory intensive and has many divergent control paths. In this paper we introduce a new algorithm which modifies the dynamic programming method to reduce its amount of data storage and eliminate control flow divergences. Our algorithm divides the problem into independent `quadrants' and makes efficient use of shared memory and registers available in GPUs to store data between different phases of the algorithm. Further, we eliminate any control flow divergences by embedding condition variables in the program logic to ensure all the threads execute the same instructions even though they work on different data items. We present an implementation of this algorithm on an NVIDIA GeForce GTX 275 GPU and compare against an optimized multi-threaded implementation on an Intel Core i7-920 quad core CPU with hyper-threading support. Our results show that our GPU implementation is up to 8x faster when operating on a large number of sequences.
更多查看译文
关键词
dynamic programming,graphics processing units,multi-threading,shared memory systems,storage management,Intel Core i7-920 quad core CPU,NVIDIA GeForce GTX 275 GPU,SIMD processors,condition variables embedding,control flow divergence elimination,data storage,divergent control paths,dynamic-programming based algorithm,fast edit distance computation algorithm,hyperthreading support,longest common subsequence problem,multithreaded implementation,program logic,shared memory,
AI 理解论文
溯源树
样例
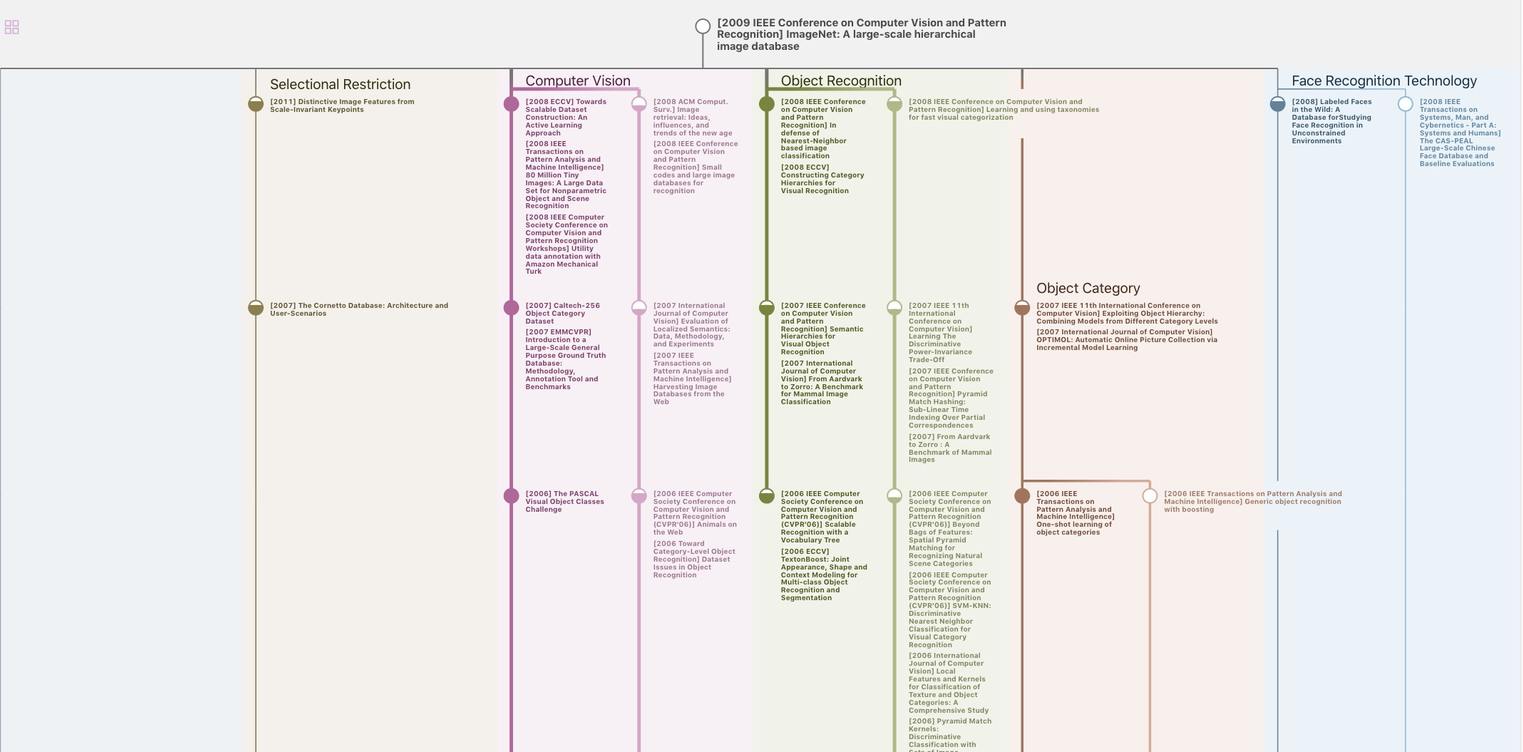
生成溯源树,研究论文发展脉络
Chat Paper
正在生成论文摘要