Force-directed tracking in wireless networks using signal strength and step recognition
ICL-GNSS(2012)
摘要
Force-directed approaches, also known as spring embedders, are widely used in the context of graph drawing and network embedding. In this paper, we study the application of these methods to signal-strength based tracking in wireless networks. The performance of the presented algorithms is evaluated based on pedestrian tracking experiments in a 60-node wireless sensor network (WSN). Additionally, we compare the outlined approach with implementations of Extended Kalman Filters (EKF) and examine similarities and distinctions between both approaches. The algorithms are developed in a 3-step process. First, we take a brief look at the trilateration problem, where a position is estimated based on a set of noisy signal strength measurements. From this we conclude how signal strengths can be translated into adequate spring forces. Subsequently, we establish a movement model by introducing additional forces between consecutive position estimates. Finally, we show how step information from a pedestrian can be used to improve the localization. Our experimental results indicate that force-directed methods offer an interesting and competitive approach to the tracking problem. Especially the possibility to easily include further information by introducing additional forces makes them very attractive. As modeled forces are not limited to linear functions, non-linear aspects such as distance estimates can be effortlessly modeled. We conclude that the application of force-directed approaches to the tracking problem offers a worthwhile direction for future research.
更多查看译文
关键词
kalman filters,target tracking,wireless sensor networks,distance estimates,extended kalman filters,force directed tracking,graph drawing,movement model,network embedding,noisy signal strength measurements,pedestrian tracking,spring embedders,spring forces,step recognition,trilateration problem,wireless networks,wireless sensor network,force,time measurement
AI 理解论文
溯源树
样例
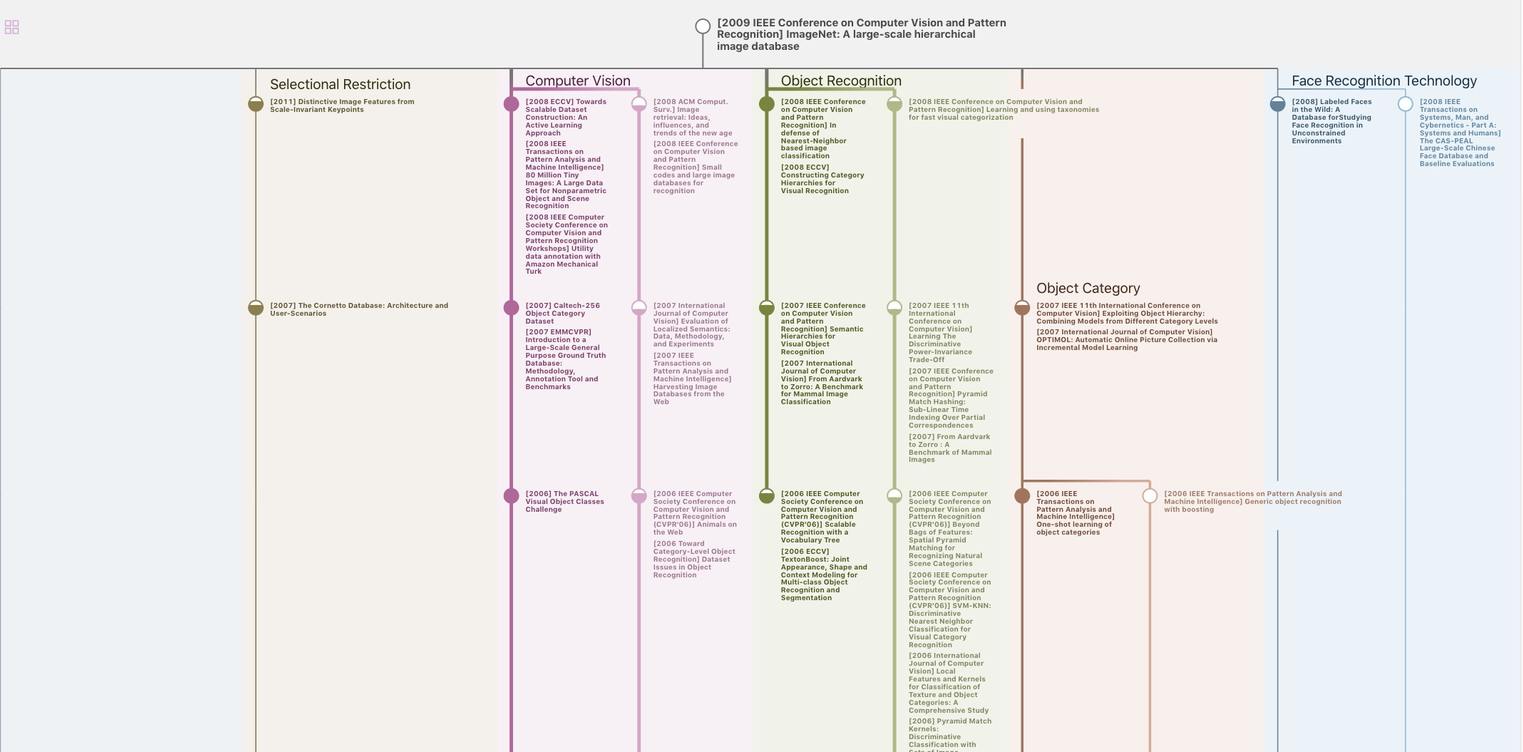
生成溯源树,研究论文发展脉络
Chat Paper
正在生成论文摘要