Steel defect classification with Max-Pooling Convolutional Neural Networks
Neural Networks(2012)
摘要
We present a Max-Pooling Convolutional Neural Network approach for supervised steel defect classification. On a classification task with 7 defects, collected from a real production line, an error rate of 7% is obtained. Compared to SVM classifiers trained on commonly used feature descriptors our best net performs at least two times better. Not only we do obtain much better results, but the proposed method also works directly on raw pixel intensities of detected and segmented steel defects, avoiding further time consuming and hard to optimize ad-hoc preprocessing.
更多查看译文
关键词
automatic optical inspection,image classification,image segmentation,neural nets,production engineering computing,steel,detected steel defects,error rate,maxpooling convolutional neural networks,production line,raw pixel intensities,segmented steel defects,supervised steel defect classification
AI 理解论文
溯源树
样例
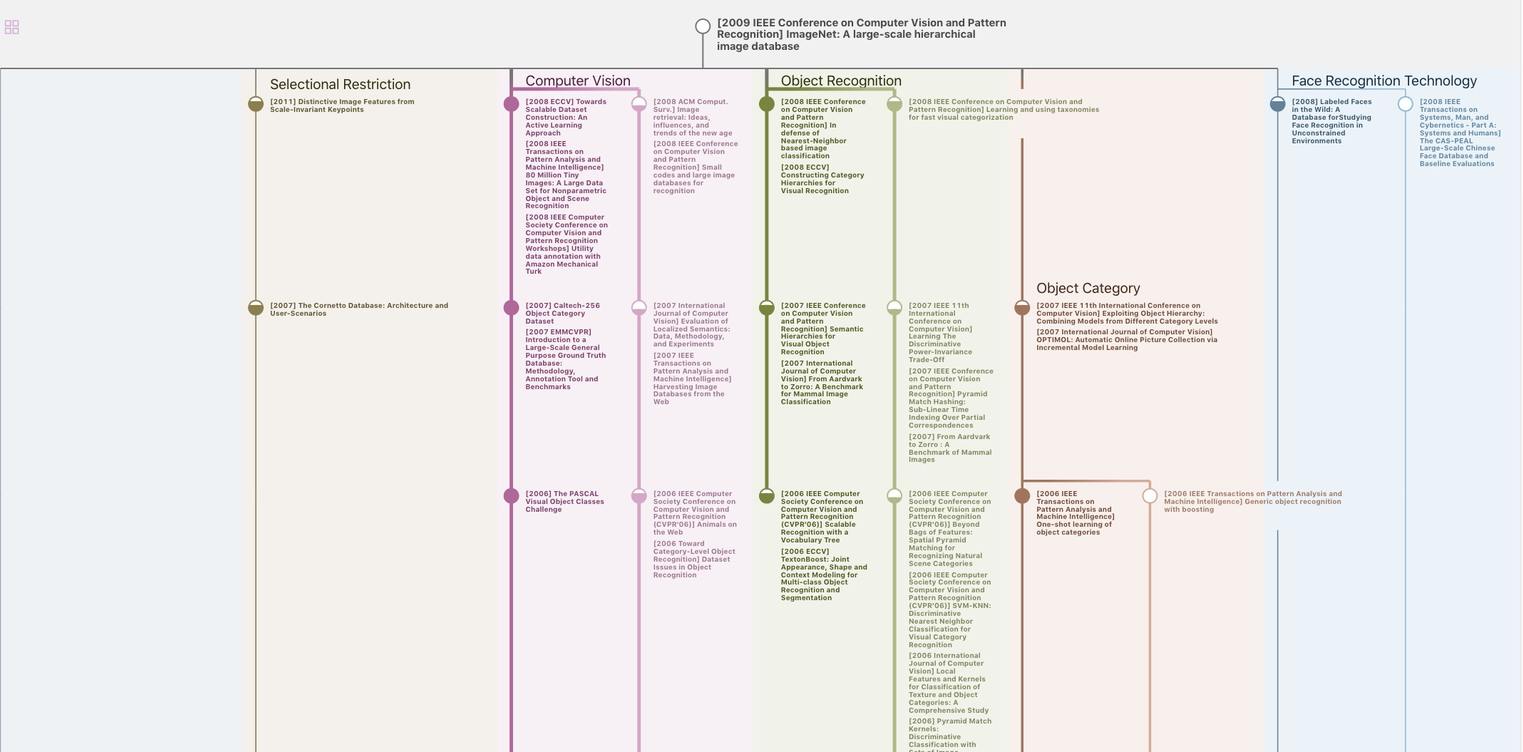
生成溯源树,研究论文发展脉络
Chat Paper
正在生成论文摘要