A new approach to system identification and parameter tuning with multiple model adaptive estimators
Image and Signal Processing and Analysis(2011)
摘要
Multiple model adaptive estimators have been used as a standard technique in system identification and systems state estimation. In its classical application, this method picks up the most probable model from a fixed set of pre-established models. This paper presents a new method, relying on multiple model adaptive estimators, that defines a set of models, with cardinality dependent on the number of unknown system parameters, and a strategy to successively update the parameters' values to approximate the system under identification. The method starts with a model for the unknown system and a suitable range of values for each unknown parameter, that can be chosen a priori or generated automatically, and from there a constellation of suitable models is constructed. In a first phase, a search is made in the parameters' space to seek the region where the true model's parameters dwell, and after that, the region's area is consecutively shrunk and the parameters are updated. Due to the adaptive nature of the constellation, for time-variant systems, when the system's parameters change the algorithm parameters can be reinitialized to estimate the new systems parameters.
更多查看译文
关键词
kalman filters,adaptive estimation,parameter estimation,state estimation,time-varying systems,kalman filter,multiple model adaptive estimators,parameter tuning,system identification,system state estimation,time-variant systems
AI 理解论文
溯源树
样例
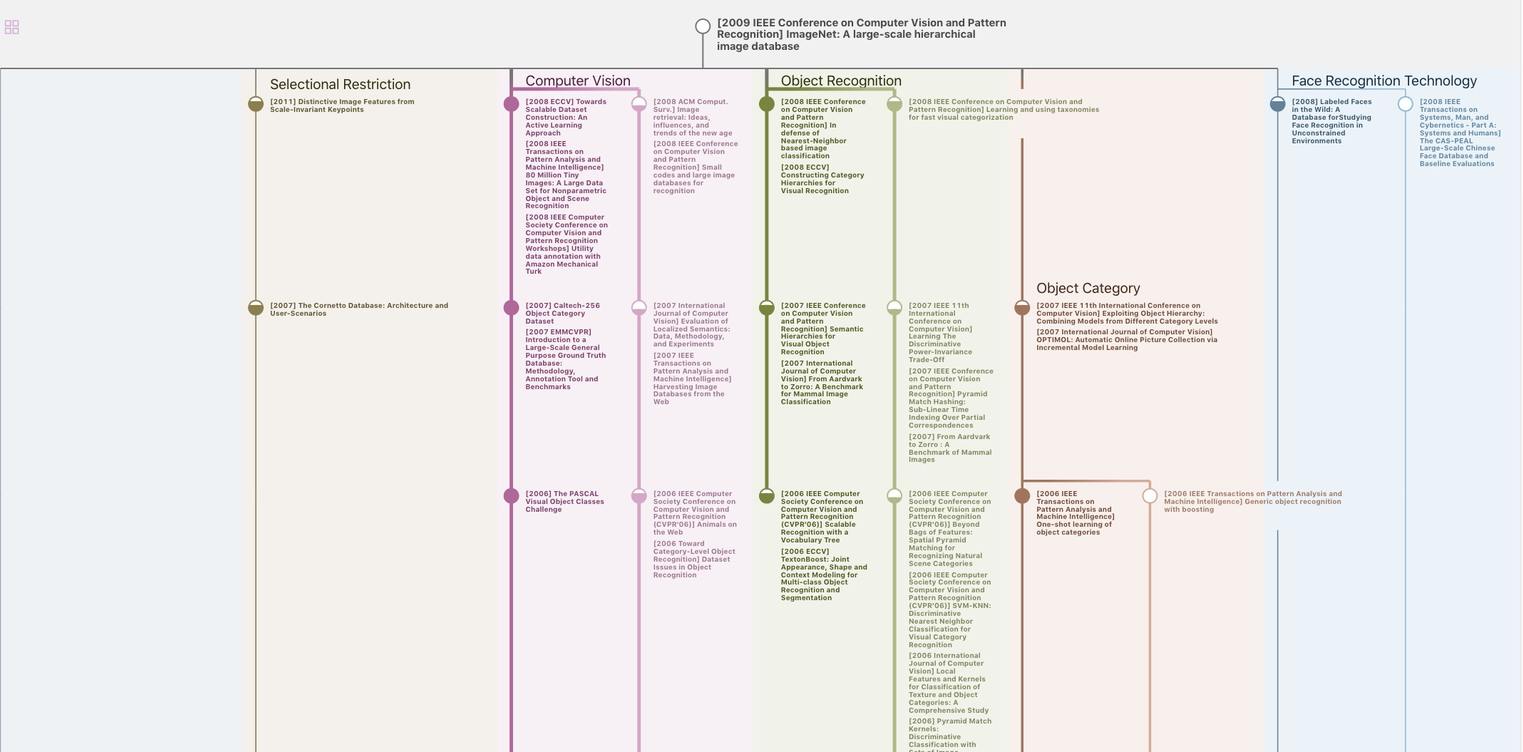
生成溯源树,研究论文发展脉络
Chat Paper
正在生成论文摘要