Transformation Pursuit for Image Classification
CVPR '14 Proceedings of the 2014 IEEE Conference on Computer Vision and Pattern Recognition(2014)
摘要
A simple approach to learning invariances in image clas- sification consists in augmenting the training set with transformed versions of the original images. However, given a large set of possible transformations, selecting a com- pact subset is challenging. Indeed, all transformations are not equally informative and adding uninformative transfor- mations increases training time with no gain in accuracy. We propose a principled algorithm--Image Transformation Pursuit (ITP)--for the automatic selection of a compact set of transformations. ITP works in a greedy fashion, by se- lecting at each iteration the one that yields the highest accuracy gain. ITP also allows to efficiently explore complex transformations, that combine basic transformations. We report results on two public benchmarks: the CUB dataset of bird images and the ImageNet 2010 challenge. Using Fisher Vector representations, we achieve an improvement from 28.2% to 45.2% in top-1 accuracy on CUB, and an im- provement from 70.1% to 74.9% in top-5 accuracy on Im- ageNet. We also show significant improvements for deep convnet features: from 47.3% to 55.4% on CUB and from 77.9% to 81.4% on ImageNet.
更多查看译文
关键词
greedy algorithms,image classification,iterative methods,vectors,CUB dataset,Fisher vector representations,ITP,ImageNet 2010 challenge,bird images,compact subset,complex transformations,greedy fashion,image classification,image transformation pursuit,invariance learning,iteration
AI 理解论文
溯源树
样例
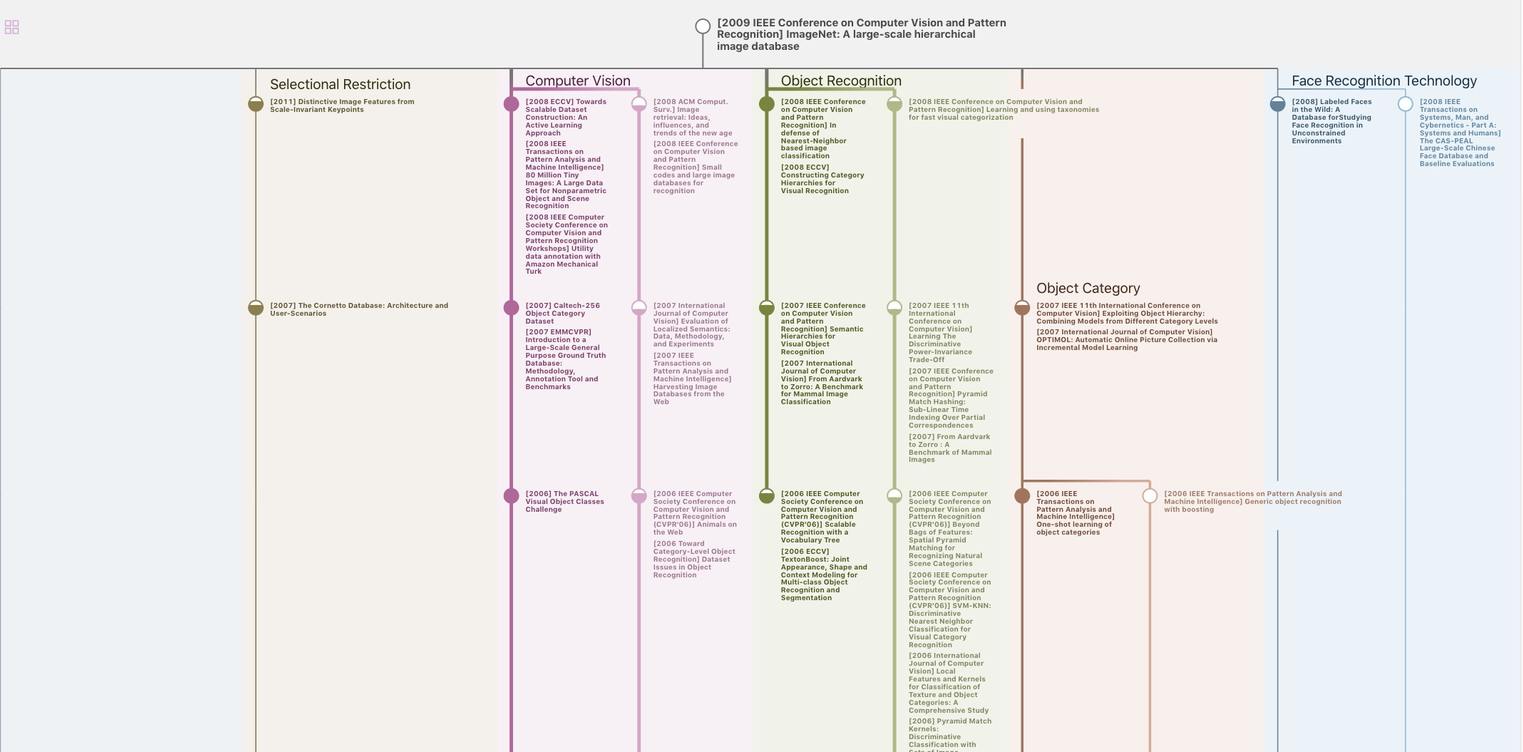
生成溯源树,研究论文发展脉络
Chat Paper
正在生成论文摘要