Nonparametric Identification of Population Models: An MCMC Approach
Biomedical Engineering, IEEE Transactions(2008)
Abstract
The paper deals with the nonparametric identification of population models, that is models that explain jointly the behavior of different subjects drawn from a population, e.g., responses of different patients to a drug. The average response of the population and the individual responses are modeled as continuous-time Gaussian processes with unknown hyperparameters. Within a Bayesian paradigm, the posterior expectation and variance of both the average and individual curves are computed by means of a Markov Chain Monte Carlo scheme. The model and the estimation procedure are tested on both simulated and experimental pharmacokinetic data.
MoreTranslated text
Key words
Bayes methods,Gaussian processes,Markov processes,Monte Carlo methods,data analysis,drugs,expectation-maximisation algorithm,medical computing,nonparametric statistics,patient treatment,Bayesian paradigm,Markov Chain Monte Carlo scheme,biomedical data analysis,continuous-time Gaussian processes,drug,nonparametric identification,pharmacokinetic data,population models,posterior expectation,Bayesian estimation,Markov Chain Monte Carlo,neural networks,nonparametric identification,pharmacokinetic data,regularization,splines
AI Read Science
Must-Reading Tree
Example
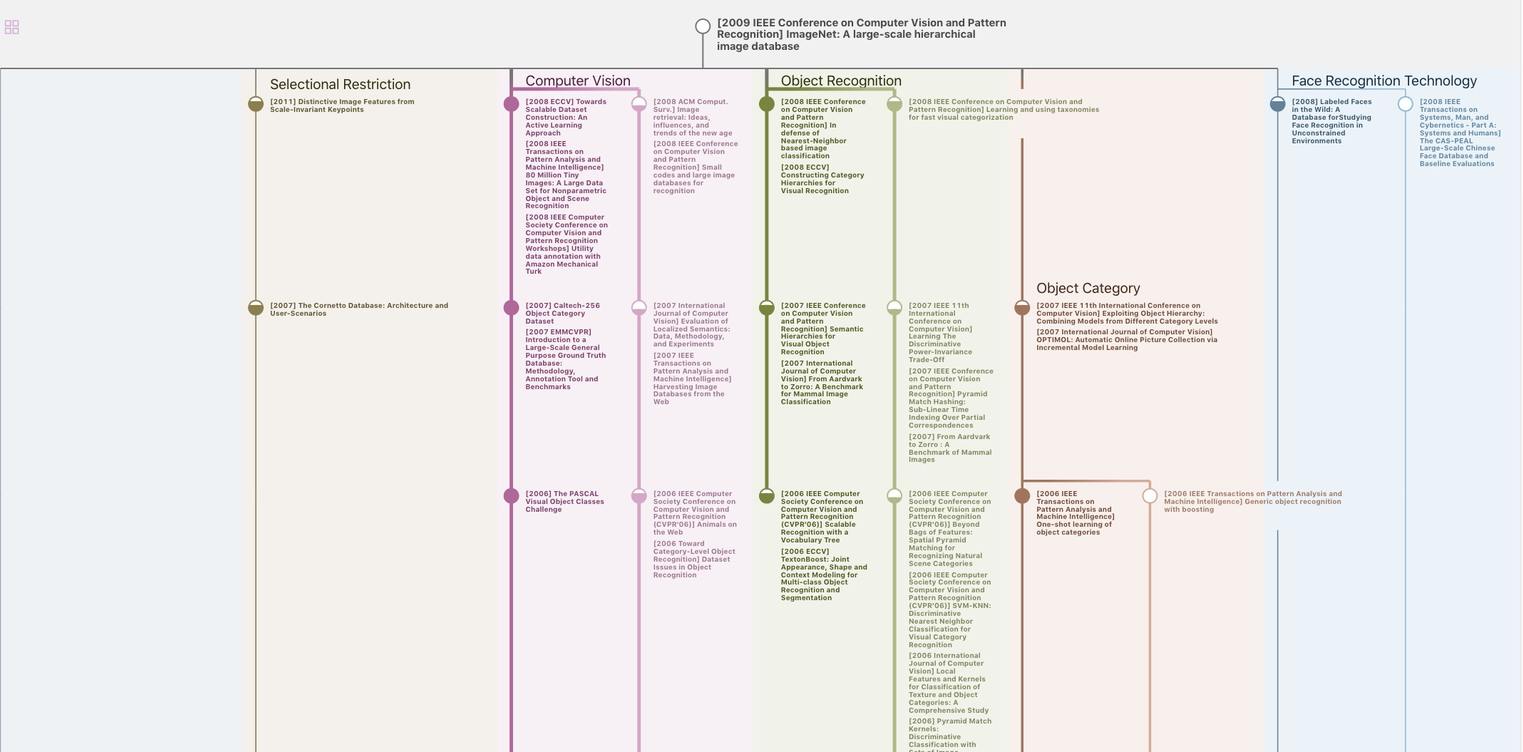
Generate MRT to find the research sequence of this paper
Chat Paper
Summary is being generated by the instructions you defined