Rainfall Nowcasting From Multisatellite Passive-Sensor Images Using a Recurrent Neural Network
Geoscience and Remote Sensing, IEEE Transactions(2007)
摘要
The term nowcast in hydrometeorology reflects the need for timely and accurate predictions of risky environmen- tal situations, which are related to the development of severe meteorological events at short time scales. The objective of this paper is to apply a fully neural-network approach to the rain- fall field nowcasting from infrared (IR) and microwave (MW) passive-sensor imagery aboard, respectively, geostationary Earth orbit (GEO) and low Earth orbit (LEO) satellites. The multi- satellite space-time prediction procedure, which is named Neural Combined Algorithm for Storm Tracking (NeuCAST), consists of two consecutive steps. First, the IR radiance field measured from a geostationary satellite radiometer (e.g., Meteosat) is pro- jected ahead in time (e.g., 30 min); second, the projected radi- ance field is used in estimating the rainfall field by means of an MW-IR combined rain retrieval algorithm exploiting GEO-LEO observations. The NeuCAST methodology is extensively illus- trated and discussed in this paper. Its accuracy is quantified by means of quantitative error indexes, which are evaluated on selected case studies of rainfall events in Southern Europe in 2003 and 2005.
更多查看译文
关键词
hydrological equipment,hydrological techniques,neural nets,rain,remote sensing,storms,AD 2003,AD 2005,NeuCAST,Southern Europe,geostationary Earth orbit satellite,low Earth orbit satellite,multisatellite passive sensor image,neural combined algorithm for storm tracking,rain retrieval algorithm,rainfall field now casting,recurrent neural network,risky environmental situation,Neural network (NN),nowcasting methods,passive sensors,precipitation retrieval,satellite remote sensing
AI 理解论文
溯源树
样例
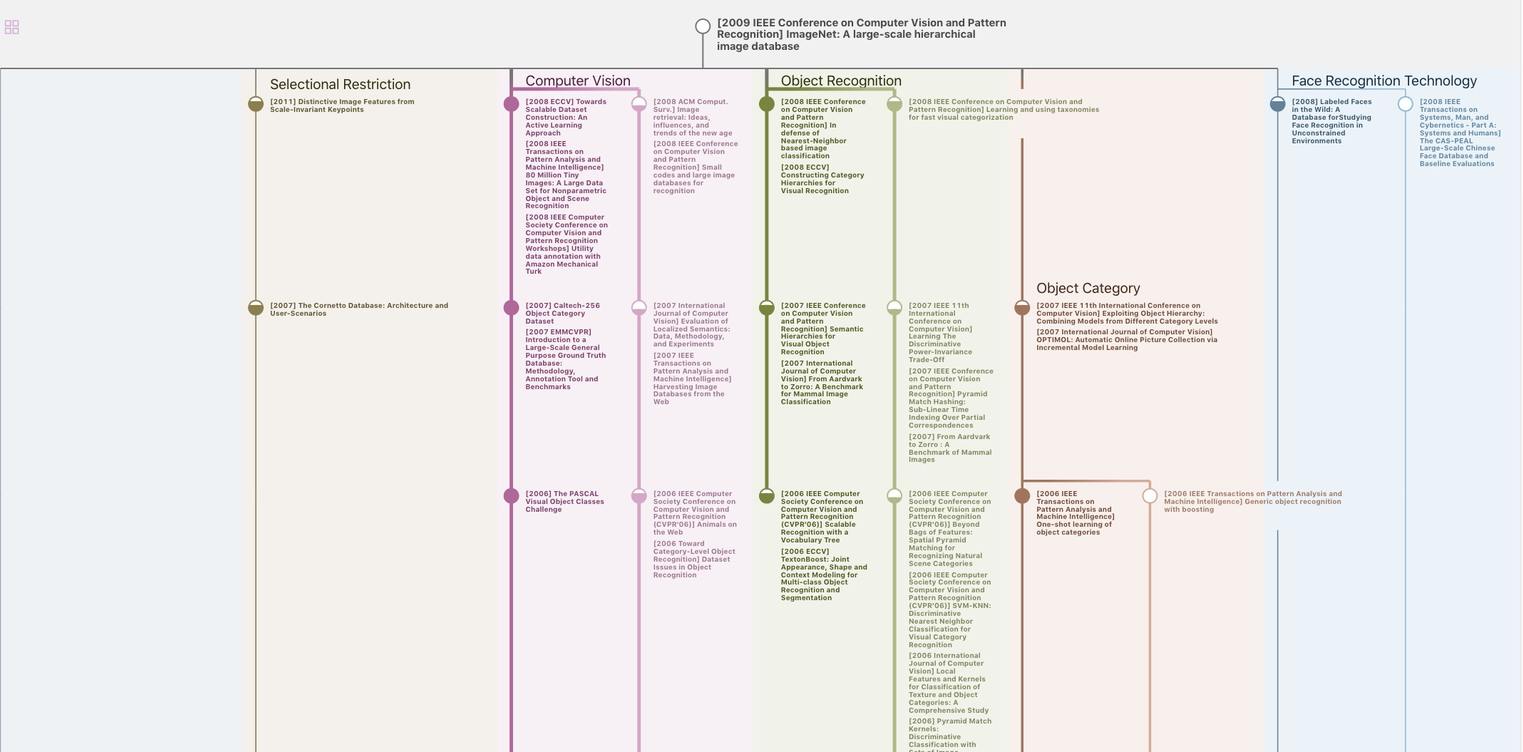
生成溯源树,研究论文发展脉络
Chat Paper
正在生成论文摘要