A statistical model for seasonal forecasting of spring break-up in Hudson Bay
Geoscience and Remote Sensing Symposium, 2004. IGARSS '04. Proceedings. 2004 IEEE International(2004)
摘要
A linear regression model is developed to predict spring ice conditions in Hudson Bay 3 months in advance. The model has 8 atmospheric, oceanic and sea ice predictors that together explain 90% of the variability in the predicted time series with a MAE of 0.26. The categorical forecast accuracy ranges from 73% to 77% A series of diagnostics including Monte Carlo simulations are used to ensure that the model is statistically significant and is not adversely affected by serial correlation or multicolinearity. The results suggest that for long-lead forecasts, sea ice severity may be forecasted using a simple statistical model that does not require any information regarding the future climate
更多查看译文
关键词
Monte Carlo methods,atmospheric techniques,climatology,oceanographic regions,oceanographic techniques,regression analysis,sea ice,weather forecasting,Hudson Bay,MAE,Monte Carlo simulations,atmospheric predictor,categorical forecast accuracy,climatology,linear regression model,long-lead forecasts,multicolinearity,oceanic predictor,sea ice predictor,sea ice severity,seasonal forecasting,serial correlation,spring break-up,spring ice condition,statistical model,time series
AI 理解论文
溯源树
样例
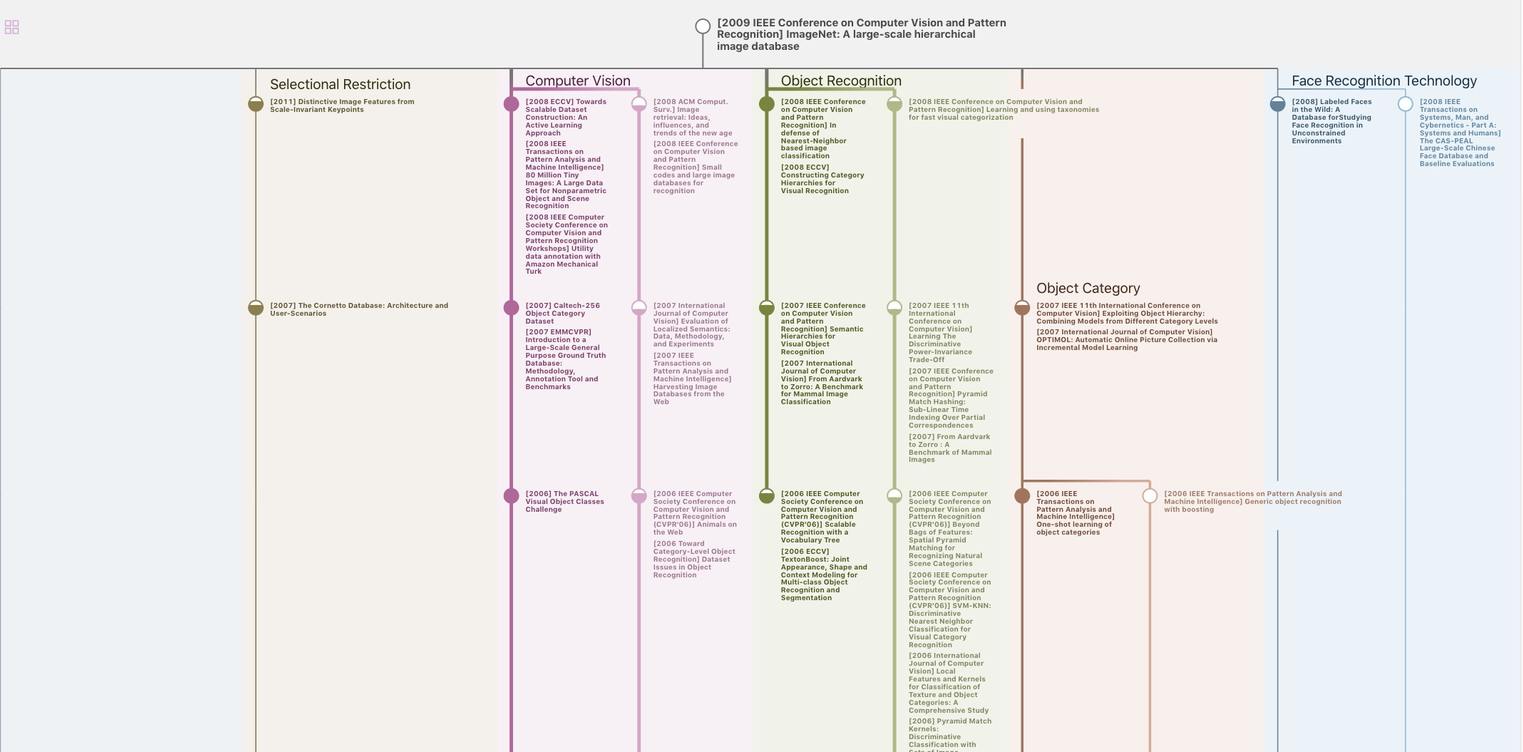
生成溯源树,研究论文发展脉络
Chat Paper
正在生成论文摘要