Converting Neural Network Language Models into back-off language models for efficient decoding in automatic speech recognition
Acoustics, Speech and Signal Processing(2014)
摘要
Neural Network Language Models (NNLMs) have achieved very good performance in large-vocabulary continuous speech recognition (LVCSR) systems. Because decoding with NNLMs is very computationally expensive, there is interest in developing methods to approximate NNLMs with simpler language models that are suitable for fast decoding. In this work, we propose an approximate method for converting a feedforward NNLM into a back-off n-gram language model that can be used directly in existing LVCSR decoders. We convert NNLMs of increasing order to pruned back-off language models, using lower-order models to constrain the n-grams allowed in higher-order models. In experiments on Broadcast News data, we find that the resulting back-off models retain the bulk of the gain achieved by NNLMs over conventional n-gram language models, and give significant accuracy improvements as compared to existing methods for converting NNLMs to back-off models. In addition, the proposed approach can be applied to any type of non-back-off language model to enable efficient decoding.
更多查看译文
关键词
back-off language models,language modeling,back-off n-gram language model,speech recognition,natural languages,feedforward nnlm,large-vocabulary continuous speech recognition,neural network language models,decoding with neural network language models,large-vocabulary continuous speech recognition systems,feedforward neural nets,lvcsr system,higher-order models,broadcast news data,lvcsr systems,lower-order models,speech coding,neural network language model,non-back-off language model,decoding,lvcsr decoders,automatic speech recognition
AI 理解论文
溯源树
样例
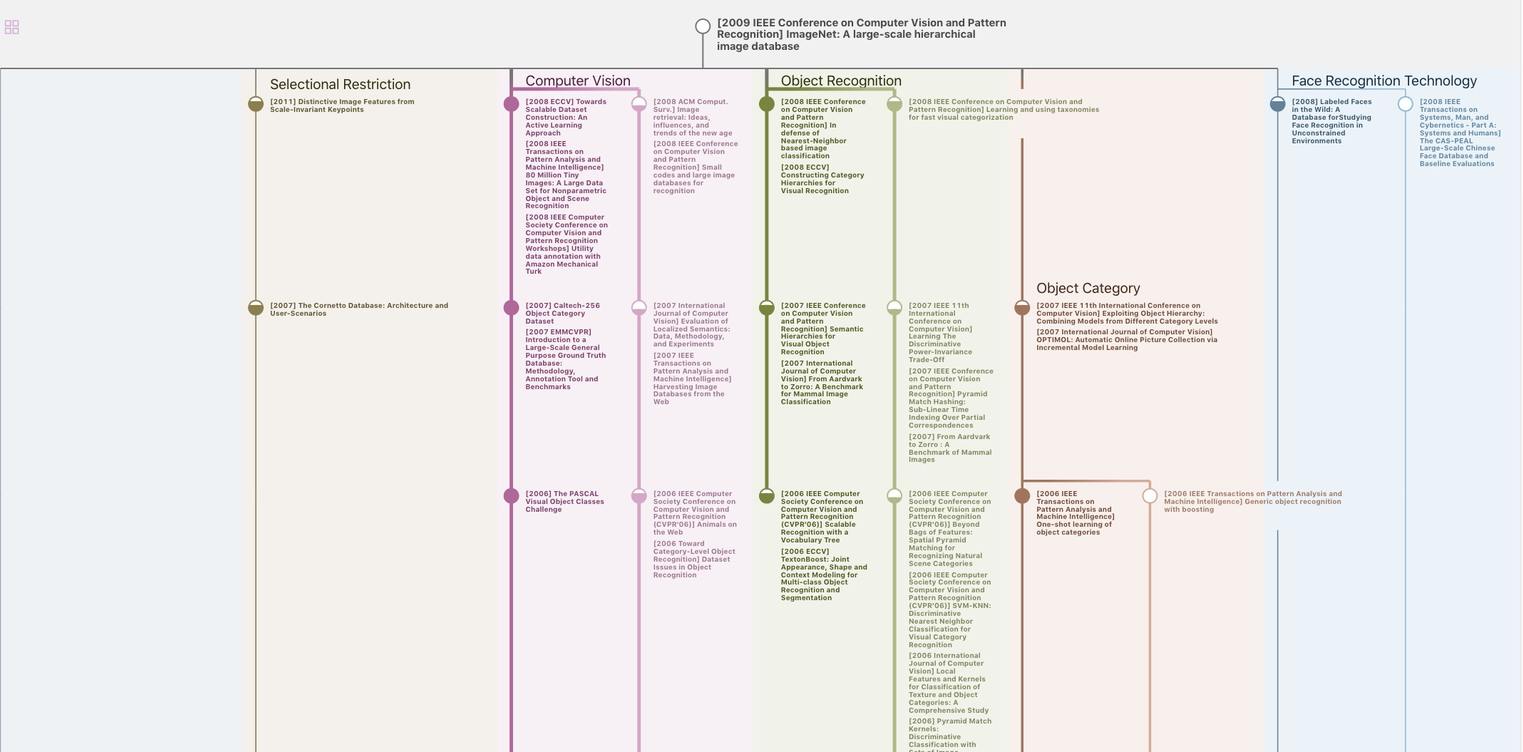
生成溯源树,研究论文发展脉络
Chat Paper
正在生成论文摘要