Using Feature Selection And Classification To Build Effective And Efficient Firewalls
Information Reuse and Integration(2014)
摘要
Firewalls form an essential element of modern network security, detecting and discarding malicious packets before they can cause harm to the network being protected. However, these firewalls must process a large number of packets very quickly, and so can't always make decisions based on all of the packets' properties (features). Thus, it is important to understand which features are most relevant in determining if a packet is malicious, and whether a simple model built from these features can be as effective as a model which uses all information on each packet. We explore a dataset with real-world firewall data to answer these questions, ranking the features with 22 feature selection techniques and building classification models using four classifiers (learners). Our results show that the top two features are proto and dst (representing the network protocol and destination IP address, respectively), and that models built using these two features in combination with the Naive Bayes learner are highly effective while being minimally computationally expensive. Such models have the potential to replace conventional firewalls while lowering computational needs.
更多查看译文
关键词
Firewall,Intrusion Detection,Classification,Feature Selection
AI 理解论文
溯源树
样例
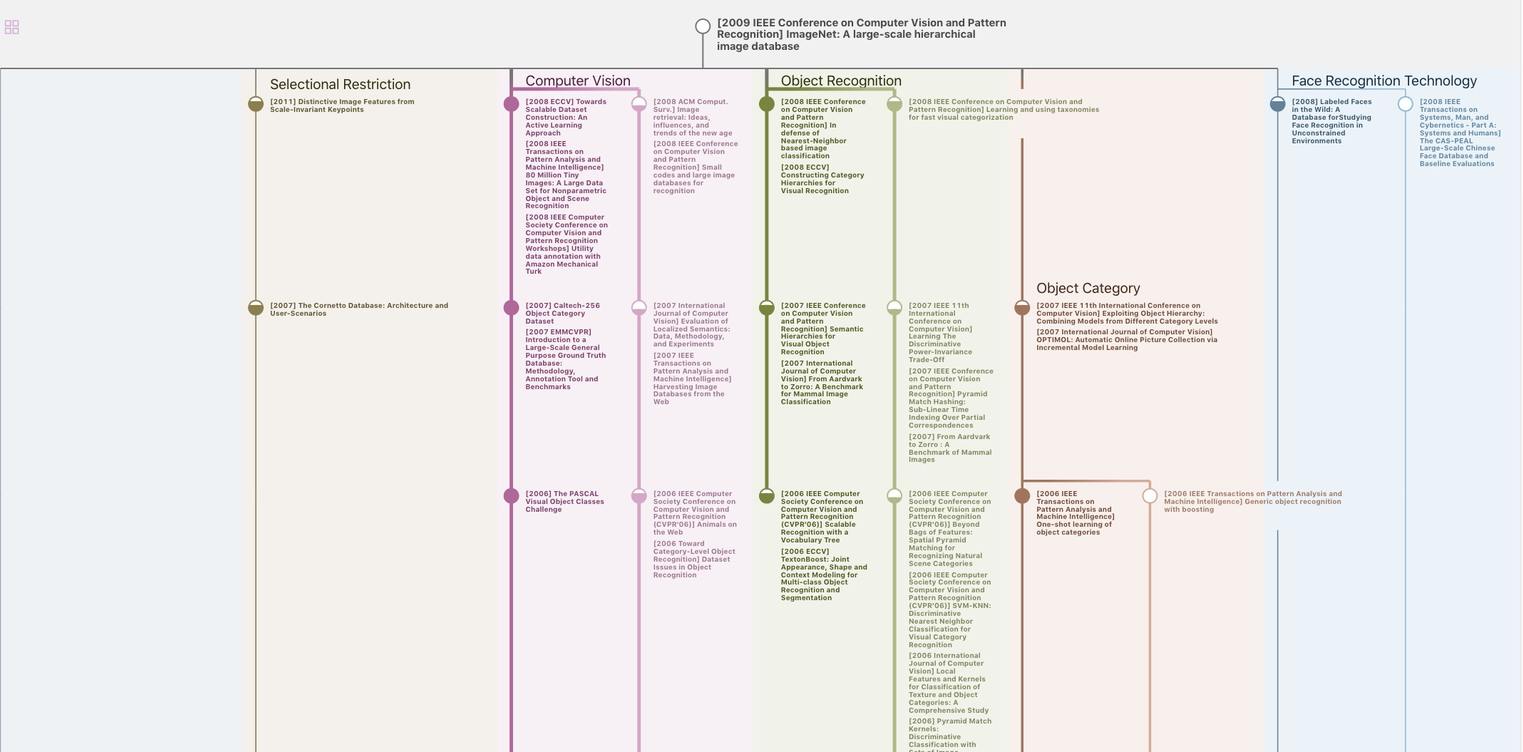
生成溯源树,研究论文发展脉络
Chat Paper
正在生成论文摘要