Multimodal Learning For Autonomous Underwater Vehicles From Visual And Bathymetric Data
Robotics and Automation(2014)
摘要
Autonomous Underwater Vehicles (AUVs) gather large volumes of visual imagery, which can help monitor marine ecosystems and plan future surveys. One key task in marine ecology is benthic habitat mapping, the classification of large regions of the ocean floor into broad habitat categories. Since visual data only covers a small fraction of the ocean floor, traditional habitat mapping is performed using shipborne acoustic multi-beam data, with visual data as ground truth. However, given the high resolution and rich textural cues in visual data, an ideal approach should explicitly utilise visual features in the classification process. To this end, we propose a multimodal model which utilises visual data and shipborne multi-beam bathymetry to perform both classification and sampling tasks. Our algorithm learns the relationship between both modalities, but is also effective when visual data is missing. Our results suggest that by performing multimodal learning, classification performance is improved in scenarios where visual data is unavailable, such as the habitat mapping scenario. We also demonstrate empirically that the model is able to perform generative tasks, producing plausible samples from the underlying data-generating distribution.
更多查看译文
关键词
autonomous underwater vehicles,feature extraction,image classification,image sampling,image texture,learning (artificial intelligence),marine engineering,mobile robots,oceanographic techniques,robot vision,AUV,autonomous underwater vehicles,bathymetric data,benthic habitat mapping,marine ecology,marine ecosystem monitoring,multimodal learning,ocean floor region classification,sampling task,shipborne acoustic multibeam data,shipborne multibeam bathymetry utilization,textural cues,visual data utilization,visual feature utilization,visual imagery
AI 理解论文
溯源树
样例
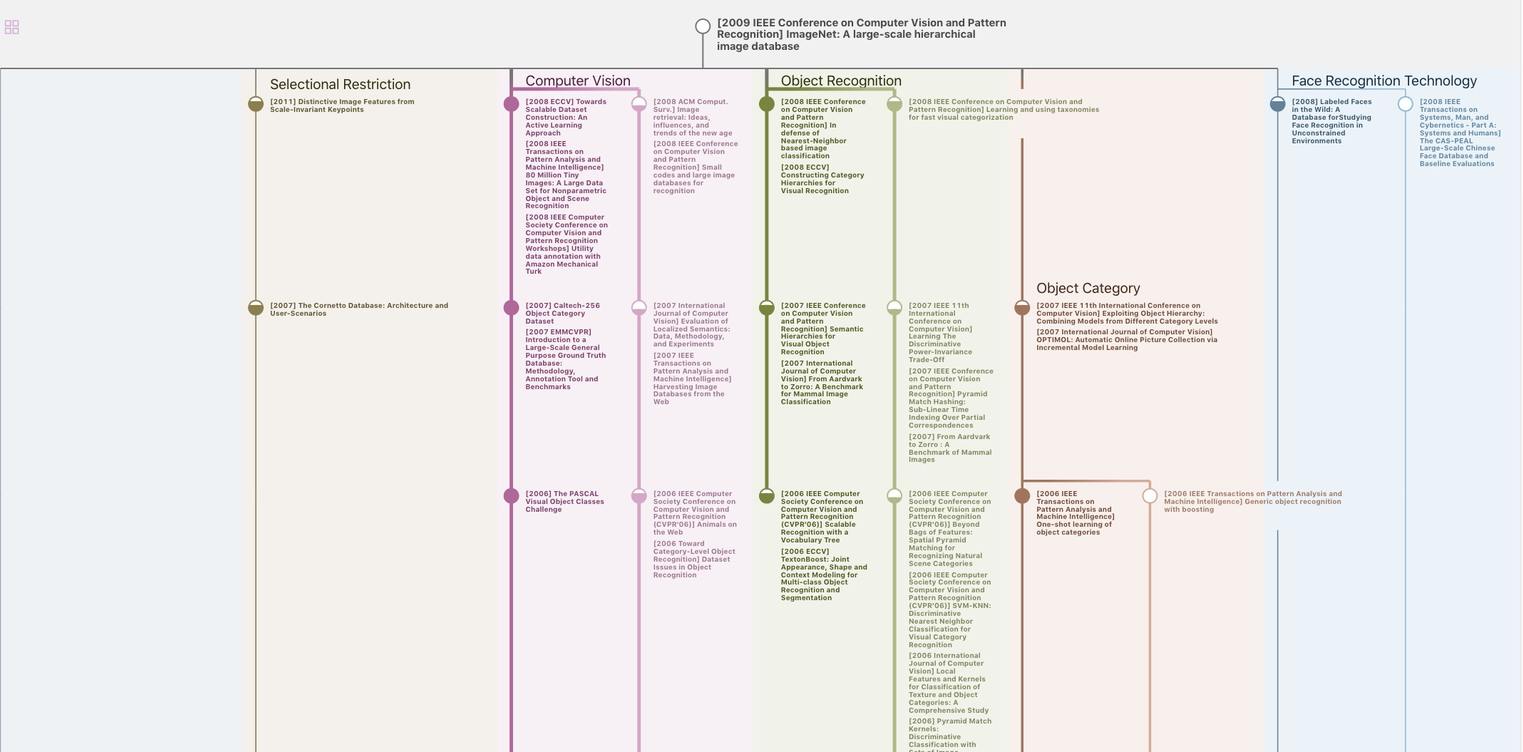
生成溯源树,研究论文发展脉络
Chat Paper
正在生成论文摘要